In an increasingly digital age, the intersection of education and cutting-edge technology is consistently transforming the landscape of learning. One of the most intriguing developments in this intersection is the use of Artificial Intelligence (AI), particularly AI-based recommendation systems.
This essay will navigate through the fascinating realm of these systems, illuminating intricacies about their operation and underlying technology. Moreover, it will delve into their application in the field of education, uncovering how these algorithm-driven tools can be used to recommend relevant content, provide personalized instruction, and create more efficient learning pathways.
From there, we will look into the implementation strategies of these systems, exploring the complexities and considerations of integrating these systems into an educational setting. Through case studies and examinations of future trends, we will further exemplify the transformative power and potential of AI-based recommendation systems in education.
Understanding the basics of AI-based recommendation systems
Table of Contents
- 1 Understanding the basics of AI-based recommendation systems
- 2 Technology behind AI-based recommendation systems
- 3 AI-based recommendation systems in Education
- 4 Implementation strategies of AI-based recommendation systems
- 5 Case studies of successful AI-based recommendation system applications
- 6 Future trends in AI-based recommendation systems for education
- 6.0.1 Application of AI-Based Recommendation Systems in Education
- 6.0.2 Adaptive Learning and Personalization
- 6.0.3 Greater Inclusion and Accessibility
- 6.0.4 Predictive Analytics and Student Success Prediction
- 6.0.5 Real-time Feedback and Automated Assessment
- 6.0.6 Learning Course Optimization
- 6.0.7 Scalability and Affordability
- 6.0.8 Conclusion
AI-based Recommendation Systems
AI-based recommendation systems have become an essential aspect of technological applications. The principles of Artificial Intelligence (AI) underlie these systems’ functionality and effectiveness, enabling them to provide a seamless user experience. Tackling the concept of AI-based recommendation systems involves understanding these fundamentals and the different type of data these systems usually handle.
Understanding AI-based Recommendation Systems
To comprehend AI-based recommendation systems, one must first appreciate the role of AI in these systems. Essentially, AI is the technology behind intelligent actions and decision-making processes of computer systems. It combines algorithms, historical data, and predictive modeling to drive output or recommendations on these systems.
In AI-based recommendation systems, AI uses a set of algorithms to analyze user behavior patterns and preferences over certain periods. It processes this data to predict future preferences and subsequently make recommendations that cater to these anticipated preferences.
Data Types in AI-based Recommendation Systems
AI-based recommendation systems deal with different types of data. Primarily, these include implicit and explicit data. Explicit data involve direct feedback from the user, such as ratings and reviews. Implicit data, on the other hand, are derived from user behavior and interactions, such as browsing history and purchase records.
Other types of data handled by AI-based recommendation systems include demographic data, which provide useful information about the user, such as age, gender, and location, and contextual data, which focus on environmental factors that could influence user preferences, such as time, season, or even the weather.
Operating Principle of AI-based Recommendation Systems
AI-based recommendation systems follow a specific operating principle, typically utilizing a processing approach known as collaborative filtering. This approach is based on the idea that individuals who have agreed in the past are likely to agree again in the future. To implement this, the system uses past behavior to generate a matrix of user-item interactions. Through machine learning, the system can then make predictions about a user’s future preferences based on similar preferences exhibited by other users.
Content-based filtering is another approach commonly used alongside or as an alternative to collaborative filtering. This method focuses on presenting items similar to those that a user has liked in the past. It achieves this by comparing the content of the items to a user profile, which is built from previously rated or interacted with items.
Importance of AI-based Recommendation Systems
AI-based recommendation systems have become an essential feature in various sectors due to their ability to offer personalized experiences. These systems are especially critical in the e-commerce industry, where they guide users through a myriad of product choices, enhancing customer satisfaction and retention.
Moreover, these systems play an integral role in online streaming platforms, such as Netflix and Spotify, where they curate content based on the user’s watch or listen history. They also find applications in news portals and social media platforms, providing users with personally relevant content.
Ultimately, obtaining proficiency in AI-based recommendation systems brings about considerable advantages, particularly in an increasingly digital landscape. By comprehending the operations, the nature of the data utilized, and the significance of these systems, educators and teachers can arm themselves with the adequate skills required to unleash the full potential of AI in their respective domains.
This not merely amplifies their capacity for implementing technology, but also induces an AI-induced evolution in contemporary education.
Technology behind AI-based recommendation systems
AI-driven recommendation systems have become a pivotal element of various platforms, especially in sectors like e-commerce and entertainment. They optimize user interactions by proposing personalized recommendations, thereby enabling businesses to strengthen customer fidelity and bolster profits.
Nonetheless, these systems necessitate several technological components from across the globe for optimal functionality: machine learning, data mining, and deep learning being the most crucial ones. Gaining an insight into these technologies, their individual roles, and their collective interactions is imperative for achieving expertise in AI-based recommendation systems.
Machine Learning in AI-Based Recommendation Systems
Machine Learning (ML) enables AI systems to learn from data without explicit programming. It is, in essence, the study of algorithms and statistical models that allows a computer system to improve with experience. For AI-based recommendation systems, ML algorithms analyze users’ previous behavior to forecast their future preferences.
They can predict what products, services, or content a user may be interested in based on their browsing history, purchases, and other online interactions. This dynamic learning and prediction ability powers the personalization feature of AI recommendation systems. However, the power of ML lies in its effective collaboration with data mining.
Data Mining in AI-Based Recommendation Systems
Data Mining is the process of discovering patterns in large data sets by intersecting methods from statistics, machine learning, and database systems. It helps to transform raw data into an understandable format. For AI-based recommendation systems, data mining organizes and processes large amounts of data that include customers’ behaviors, interests, and preferences.
This data could be overt, like user ratings, or implicit, like browsing history. The output of this process provides the necessary material for machine learning algorithms, enabling the system to deliver personalized recommendations. However, data mining and machine learning are significantly enhanced when coupled with deep learning.
Deep Learning in AI-Based Recommendation Systems
Deep Learning, a subset of machine learning, uses artificial neural networks with several layers (known as deep neural networks) to model and understand complex patterns in datasets. For AI-Based recommendation systems, deep learning algorithms handle more abstract and high-level features embedded in the data.
Deep learning algorithms can discover intricate structures within a data set by using these layered architectures to recognize patterns on multiple levels of abstraction. This capacity results in more precise and contextually accurate recommendations, providing an enhanced user experience.
AI-Based recommendation systems are a product of collective contributions from machine learning, data mining, and deep learning. Each plays a crucial role in understanding user preferences, processing data to identify patterns, and ensuring the system can learn and adapt over time.
Despite the distinct roles they play, these technologies form a synergistic triad: the results of data mining fuel the learning capability of machine learning algorithms, and deep learning takes this learning to an advanced level.
Together, these three technologies form an interconnected web that powers AI-based recommendation systems, enabling decision-making based on insights and creating rich, personalized user experiences. It is essential to understand that these technologies do not operate in silos when working within a recommendation system—they learn from each other and evolve together.
Wrapping up, it becomes evident that the technical foundations of AI-based recommendation systems—machine learning, data mining, and deep learning—are not just vital, but intricately intertwined. A comprehensive understanding of these technologies paves the way for crafting more effective, sharp, and flexible recommendation systems.
As educators and teachers, harnessing this knowledge empowers us to teach our students with accuracy and ease, nurturing future innovators to contribute significantly to this technology’s advancement.
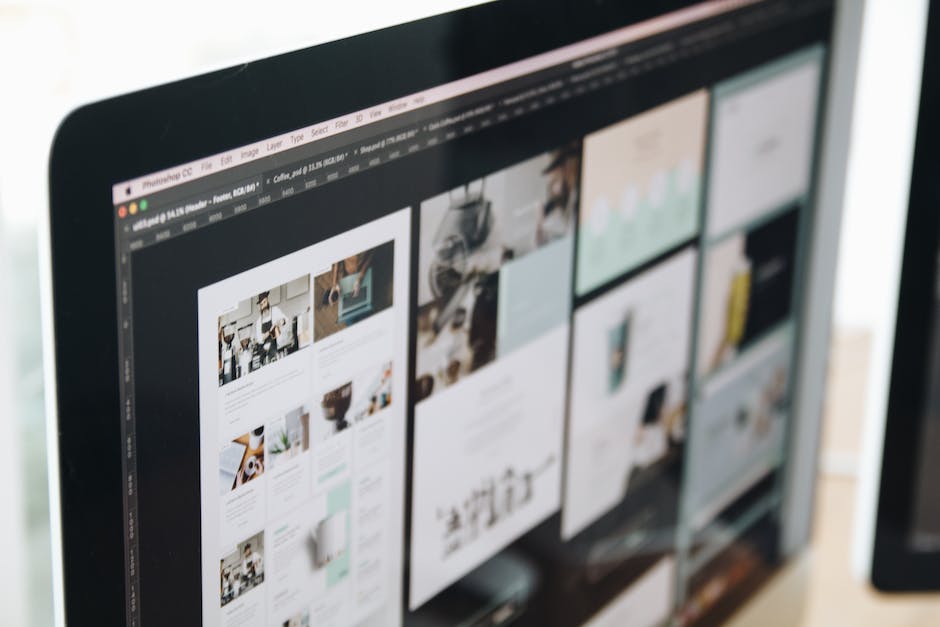
AI-based recommendation systems in Education
Diving into AI-Based Recommendation Systems
AI-based recommendation systems stretch across numerous industries, revolutionizing the modus operandi of businesses, healthcare entities, and even recreational activities. While most people are familiar with the effectiveness of these systems in suggesting movies on Netflix or merchandise on Amazon, there’s less awareness about their transformative impact on the educational field. This narrative delves into the rapid adoption of these systems in education and explores the significant role they play in recommending courses, resources, and educational content to learners.
Application of AI-Based Recommendation Systems in Education
Implementing AI-based recommendation systems in education is an innovative way to personalize the learning experience for students. It uses data on a student’s past performance and interests to recommend courses, reading materials, and other educational resources that align with their learning style and career aspirations.
For instance, if a student shows enthusiasm and performs well in mathematics, the system would recommend advanced mathematics courses or related disciplines like physics and engineering. These systems also consider a student’s weaknesses or areas where they need improvement. If a student is struggling in English literature, for instance, it might recommend additional resources or tutoring classes.
Furthermore, these recommendation systems are handy in digital learning platforms where they help suggest courses depending on the learner’s progress and preference. This empowers students to take control of their own learning pathways.
Benefits of AI-Based Recommendation Systems in Education
The adoption of AI-based recommendation systems in education carries immense benefits. First, it fosters personalization. Each student is unique in their learning style and pace; these systems consider these individual differences, offering learning recommendations tailored to meet their distinctive needs. This can lead to an improved learning experience and better learning outcomes.
Secondly, AI-based recommendation systems help to optimize study time by offering the most relevant study materials to the student. For instance, rather than a student wasting time scouting a vast library for resources, the system presents the most relevant resources depending on subject matter and learner’s preference.
Last but not least, teachers and educational institutions can also benefit from these recommendation systems. They provide teachers with insight into a student’s learning patterns, strengths, and weaknesses, assisting them in tailoring instruction more effectively.
Conclusion: Embracing the Future Now
As educators, we find ourselves standing at the epicenter where Artificial Intelligence intersects with Education. The impact of AI-based recommendation systems is undeniable, as they continue to revolutionize personalized learning experiences. By facilitating the delivery of tailored educational paths, these systems effectively cater to unique student learning needs.
As progress continues to be made in technology and research, we anticipate these systems to become even more advanced and efficient. The integration of AI-based recommendation systems in our teaching methods underlines the evolution of education – it’s not only propelled by technology but has also shifted towards deep personalization.
Implementation strategies of AI-based recommendation systems
Understanding AI-Based Recommendation Systems
AI-based recommendation systems have emerged as a cornerstone in the creation of personalized learning settings. Drawing upon algorithms and available data, these systems provide content suggestions tailored to users, based on their previous engagements and choices. However, successfully integrating these systems into your platform requires careful planning. Factors such as costs, the skills needed, tech specifications, and potential roadblocks must be considered and understood.
Understanding AI-Based Recommendation Systems
Before delving into the implementation strategies for AI-based recommendation systems, it’s crucial to have a clear comprehension of how these systems function. Principle workings of an AI-based recommendation system involve data collection, data processing, and data application. These systems collect data related to a user’s behavior, preferences, or interaction records. This data then undergoes processing using AI algorithms to make accurate predictions and deliver personalized recommendations.
Considerations for Implementing AI-Based Recommendation Systems
One of the pivotal considerations when implementing AI-based recommendation systems is your budgetary limitations or financial resources. Installation and maintenance of these systems can be cost-intensive. These costs could potentially encompass purchase of the necessary hardware and software, system integration, continued system maintenance and updates, as well as potential training for educators and administrators to effectively use the system.
In terms of skills, the implementation of recommendation systems requires multiple experts in the field of AI, machine learning, data analysis, education and curriculum decision-making. Data scientists work to fine-tune the AI algorithms, while educators and curriculum decision-makers work to ensure the system’s recommendations align with educational best practices.
Technical requirements for the system implementation are another vital consideration. The infrastructure should be able to handle large data sets, good network connectivity, and large storage capacity. It’s also necessary to ensure the system compatibility with the existing platforms and systems used within the educational institution.
Overcoming Challenges in Implementation
Implementation of AI-based recommendation systems comes with challenges, but strategic planning can help to mitigate them. One of the main challenges is privacy and security of user data. To tackle this, implementing robust security measures, following data privacy regulations, and effectively informing users how their data will be used are required steps.
Another potential challenge could be resistance from educators or students to adapt to the new system. This can be mitigated by effective training programs that highlight the benefits and usability of the system, as well as listening to and addressing educators’ and students’ feedback.
Identifying the Perfect AI-Based Recommendation Systems for Your Needs
Deciding on the most appropriate AI-based recommendation system requires careful evaluation to align with your institution’s specific needs. Factors such as the size of the educational facility, the extent of customization required for content recommendations, the ability of the system to cater to an increasing number of users, and its overall user-friendliness should guide your choice. Remember to assess the system’s previous results, its applicability and success in similar setups, and the after-sales support and services provided by the system’s developers.
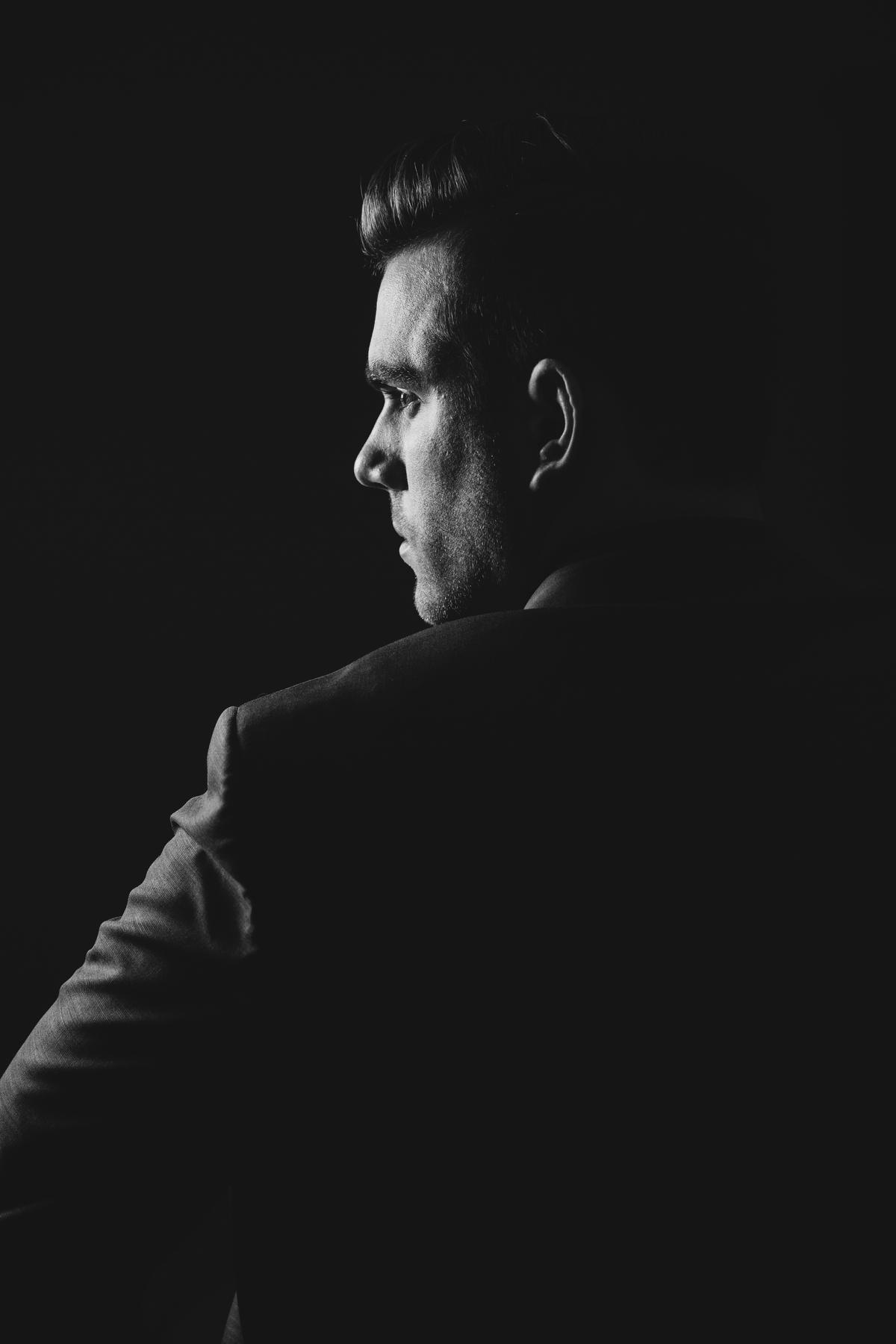
Photo by drew_hays on Unsplash
Case studies of successful AI-based recommendation system applications
A Brief Overview of AI-Based Recommendation Systems
The advent of Artificial Intelligence (AI)-based recommendation systems has rapidly transformed numerous industries, education being one of them. These systems employ complex algorithms that analyze data, decipher patterns, and subsequently come up with recommendations, tailored to the individual user.
Ranging from digital learning platforms to brick-and-mortar school structures, AI-based recommendation systems now work to curate personalized learning experiences, attuned to the unique characteristics of every learner, including their respective skills, needs, and interests.
AI in Education: Real-world Case Studies
There are many successful applications of AI-based recommendation systems in the education sector. A prominent example is the Georgia Institute of Technology, which introduced a virtual teaching assistant named “Jill Watson” in 2016.
This AI-driven tutor was powered by IBM’s Watson Analytics, providing tailored feedback, answering students’ queries, and recommending relevant resources in real-time. The assistant was so proficient that many students did not realize they were interacting with an AI. Jill Watson improved student engagement, response times, and overall learning outcomes.
Another example is “Brightspace,” a learning platform developed by Desire2Learn. Brightspace uses an AI recommendation system to identify learning gaps and recommend personalized learning trajectories for students. By predicting learners’ performance based on their activities, the system adjusts course content and learning paths accordingly. This allows educators to intervene early when students risk falling behind, helping boost academic outcomes.
The Impact of AI-Based Recommendation Systems
AI-based recommendation systems are transforming education by providing personalized and adaptive learning experiences. For instance, the adaptive learning platform “ALEKS”, developed by McGraw Hill, uses AI to assess a learner’s knowledge and skills, and then recommends the best learning path for them. Such systems have shown remarkable improvements in learning outcomes, educational equity, and student retention rates.
In K-12 education, a web-based tool known as “DreamBox Learning” leverages AI to deliver personalized math education. The system adapts the instruction level based on students’ individual performance and provides recommendations to teachers on where additional intervention might be needed. A Harvard study found students using DreamBox Learning showed a 5.5% higher achievement rate than those not using the tool.
A similar strategy is applied in the IBM Teacher Advisor program, which leverages AI to provide teachers with curated math resources based on the needs and levels of their students. It not only marks the areas where a student may need additional help but also suggests strategies and resources to bridge the gap.
The Future of AI-Based Recommendation Systems in Education
The utilization of AI-based recommendation systems in education has shown significant potential. As AI continues to evolve, we can anticipate a more seamless integration of such systems into education. For instance, these recommendation systems could propose real-time modifications to learning modules based on students’ performance on certain tasks. Similarly, they could identify learners at risk of falling off course and recommend proactive measures to keep them on track.
A more advanced future application could be multi-disciplinary learning pathways, where AI-based systems draw from a wide range of resources to support blended learning. Imagine a system that understands a student’s math strengths, English reading levels, and interest in space science, and recommends a learning project that involves calculating rocket trajectories. The possibilities are vast and can redefine the concept of personalizing education.
Wrapping Up
Case studies reveal how AI-based recommendation systems applications have successfully enhanced the educational process. They achieve this by offering tailored learning experiences and resources that tap into the unique needs of individual learners. These systems significantly influence learning outcomes and as technology advances, with more establishments employing these sophisticated tools, the educational ecosystem prepares itself for future evolutions that are advantageous to both educators and students.
Future trends in AI-based recommendation systems for education
Application of AI-Based Recommendation Systems in Education
AI-based recommendation systems are finding their place not only in e-commerce or content streaming platforms but in the academic field as well. This shift is driven by a growing demand for more personalized learning experiences and the dramatic increase of e-Learning platforms, a product of the ongoing COVID-19-induced realities. As the use of artificial intelligence in education maintains this momentum, it is expected that certain trends will shape the role and impact of AI-based recommendation systems in the education of the future.
Adaptive Learning and Personalization
AI-based recommendation systems can create highly adaptive learning environments. They assess individual learners’ needs, their learning patterns, weaknesses, and strengths, and subsequently develop personalized learning curriculums. This process enhances student engagement and increases the efficiency of learning by allowing students to focus on areas where they need improvement. The emergence of more sophisticated AI algorithms will make these recommendation systems even more accurate, leading to highly effective and individualized learning experiences.
Greater Inclusion and Accessibility
Another significant trend is the application of AI-based recommendation systems spreading to more diverse educational settings. This trend includes exclusive services for learners with special needs, disadvantaged students, or those from lower socio-economic backgrounds. AI can make education more inclusive by tailoring educational content to meet the unique needs of these learners, thereby reducing the education gap.
Predictive Analytics and Student Success Prediction
The use of predictive analytics in AI-based recommendation systems for education will usher in advancements that enable educators to monitor students’ progress accurately. They can track individual students’ performance, identify patterns, predict future outcomes, and provide timely interventions when needed. For instance, advanced AI algorithms can predict which students are at risk of falling behind or dropping out, thereby allowing educators and administrators to intercede in time.
Real-time Feedback and Automated Assessment
AI-based systems are becoming increasingly equipped to provide real-time feedback on students’ performance, further enhancing personalized learning. Besides, they can automate the assessment process, reducing teachers’ workload, and allowing them to focus more on actual teaching. As real-time feedback gets more precise, we can expect better student engagement, as students will know exactly where they stand and what they need to work on.
Learning Course Optimization
As AI-based recommendation systems evolve, one of the critical areas they are set to impact is learning course optimization. AI will assist in redefining the structure of courses by analyzing the effectiveness of different learning materials. Consequently, this could lead to the creation of more engaging and effective learning programs in the long run.
Scalability and Affordability
Finally, as AI technology becomes more commonplace, its implementation in educational contexts will likely become more affordable. This potential affordability will allow smaller educational institutions, individual educators, and even students to utilize AI-based recommendation systems, thus democratizing education.
Conclusion
As the realm of education continues to adopt and adapt to AI-based recommendation systems, it’s crucial for educators to stay updated with these trends. They’ll not only dictate the course curriculum but will also pave the way for how the future generation will learn – making it more individualized, inclusive, efficient, and engaging.
While there might be challenges along the way, the potential benefits that these recommendation systems could bring to the education sector are immense and exciting, making this an inspiring field to watch in the coming years.
As we look into the digital horizon, it’s undeniable that the use and influence of AI-based recommendation systems in the field of education will further penetrate and develop. While promptly addressing potential challenges, these systems offer unprecedented opportunities for evolving and enhancing both teaching and learning experiences.
They aid in personalizing the educational journey, providing content that is most relevant and beneficial per individual learning pathways. Furthermore, they can also address inequality in education, enabling access to quality, personalized learning resources for all students, regardless of geography or socio-economic background. The journey towards fully harnessing the potential of such technology is thrilling, and as we continue to explore, innovate, and adapt, the future of education is brighter than ever.
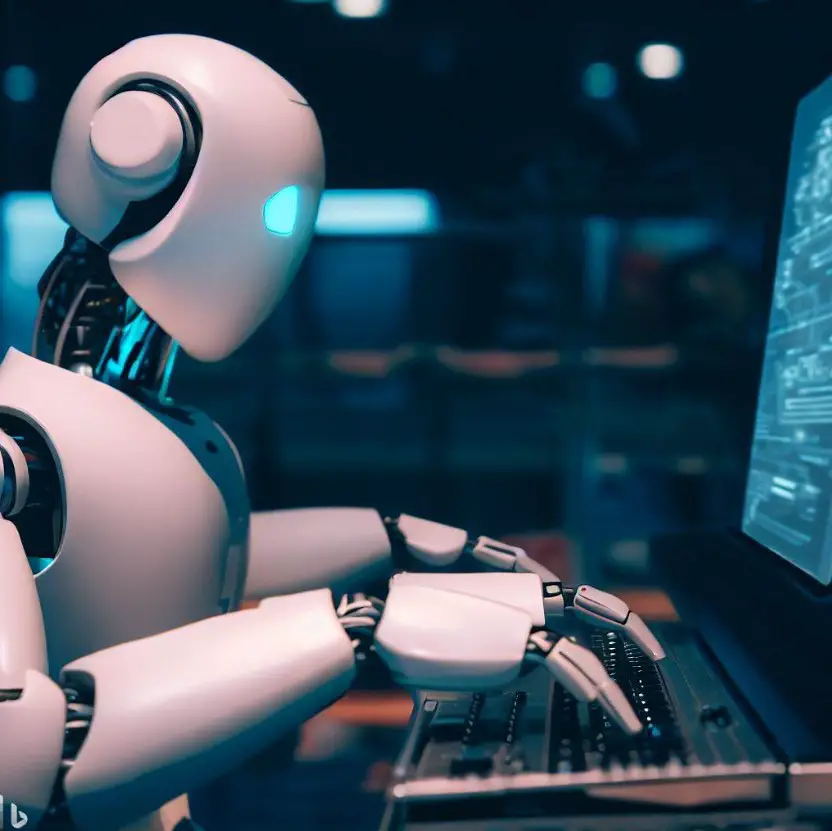
I’m Dave, a passionate advocate and follower of all things AI. I am captivated by the marvels of artificial intelligence and how it continues to revolutionize our world every single day.
My fascination extends across the entire AI spectrum, but I have a special place in my heart for AgentGPT and AutoGPT. I am consistently amazed by the power and versatility of these tools, and I believe they hold the key to transforming how we interact with information and each other.
As I continue my journey in the vast world of AI, I look forward to exploring the ever-evolving capabilities of these technologies and sharing my insights and learnings with all of you. So let’s dive deep into the realm of AI together, and discover the limitless possibilities it offers!
Interests: Artificial Intelligence, AgentGPT, AutoGPT, Machine Learning, Natural Language Processing, Deep Learning, Conversational AI.