Artificial intelligence is revolutionizing numerous industries and healthcare is no exception. The fusion of AI with advanced medical technologies holds the potential to transform patient outcomes, streamline the healthcare system, and improve disease detection and treatment methods. This essay delves into various aspects of AI in healthcare, from machine learning algorithms to ethical considerations and practical applications.
Introduction to Autonomous AI Agents in Healthcare
Table of Contents
- 1 Introduction to Autonomous AI Agents in Healthcare
- 2 AI in Healthcare and its Importance
- 3 Essential Machine Learning Algorithms of Autonomous AI Agents in Healthcare
- 4 Natural Language Processing (NLP) and Healthcare | Autonomous AI Agents in Healthcare
- 5 Medical Imaging and Computer Vision | Autonomous AI Agents in Healthcare
- 6 AI Ethics and Privacy in Healthcare | Autonomous AI Agents in Healthcare
- 7 AI-Based Decision Support Systems of Autonomous AI Agents in Healthcare
- 8 Healthcare Data and Data Preprocessing
- 9 Deep Learning and Neural Networks in Healthcare
- 10 Autonomous AI Agents in Healthcare Case Studies
Artificial intelligence (AI) has transformed various industries, and healthcare is no exception. AI is a broad term used to define the simulation of human intelligence in machines, allowing them to learn, reason, and problem-solve autonomously or semi-autonomously. It encompasses various techniques and algorithms that enable computers to perform tasks usually associated with human cognition.
In recent years, Autonomous AI Agents in Healthcare have been increasingly applied to improve healthcare systems and deliver more efficient and personalized care, ultimately leading to better patient outcomes.
Machine learning (ML), a subset of AI, is one of the most significant drivers of this revolution in healthcare. It allows computers to recognize patterns and trends by drawing from vast amounts of data, improving their performance over time without being explicitly programmed. ML can be used in healthcare to make predictions, such as identifying high-risk patients, predicting disease progression, and optimizing treatment options. For instance, it can help generate more accurate and personalized treatment plans for cancer patients by analyzing complex biomolecular data, leading to better therapeutic outcomes.
Natural language processing (NLP), another subset of AI, focuses on the interaction between humans and computers using natural language. NLP is especially useful in healthcare as it can help extract valuable information from unstructured data sources, such as electronic health records (EHRs), radiology reports, and clinical notes.
The insights gained from this data can support various healthcare processes, from patient diagnosis to treatment planning and follow-up. Additionally, NLP algorithms can aid health professionals in staying up-to-date with the latest medical research, analyzing medical literature and synthesizing relevant information.
Computer vision, also a branch of AI, deals with the automatic extraction, analysis, and understanding of useful information from digital images or videos. In healthcare, computer vision is primarily used to analyze medical images (such as X-rays, CT scans, and MRIs) to detect anomalies and help diagnose a range of conditions.
For instance, AI-powered computer vision can be employed to quickly and accurately detect cancers in mammograms, brain tumours in MRIs, or pneumonia in chest X-rays, allowing for earlier intervention and better prognosis for patients.
Autonomous AI Agents in Healthcare technologies, such as machine learning, natural language processing, and computer vision, hold significant potential to revolutionize the healthcare industry. As vast amounts of data are generated in healthcare, AI systems can aid clinicians in making more informed decisions, leading to enhanced diagnostics, personalized treatments, and streamlined workflows.
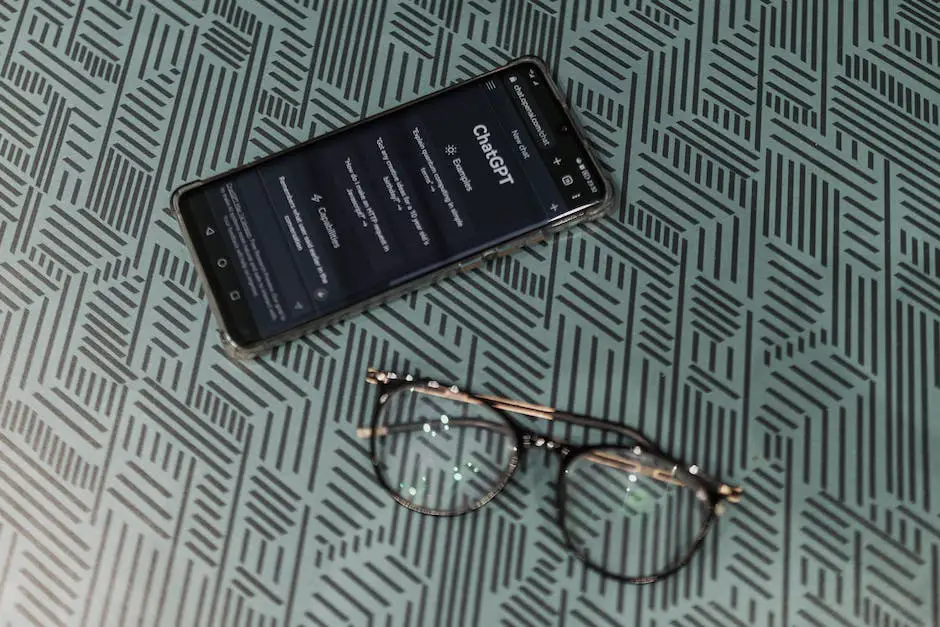
AI in Healthcare and its Importance
In addition to these applications, AI is transforming healthcare on several other levels, including detection, diagnosis, treatment, and medical equipment. AI-powered solutions enable healthcare professionals to collect crucial data, make smarter decisions, and optimize their workflow, which ultimately results in higher quality and improved patient care.
Machine learning algorithms play a key role in advancing numerous aspects of the industry, such as early disease detection, drug discovery, and tailor-made treatments for individual patients.
One major benefit of Autonomous AI Agents in Healthcare is the ability to improve diagnostic accuracy. AI algorithms analyze vast amounts of medical imaging data, such as MRI, X-ray, and CT scans, significantly faster than a human can, identifying patterns and abnormalities with exceptional precision.
This allows doctors to make more informed decisions about a patient’s condition and develop appropriate treatment plans. Additionally, AI can also assist in extracting crucial information from electronic health records and predict potential risks for patients, enabling healthcare providers to take preventive measures before complications arise.
Moreover, Autonomous AI Agents in Healthcare have the potential to significantly drive down healthcare costs, making it more accessible to a broader population. By automating mundane tasks and reducing administrative workload, AI-powered solutions offer an opportunity to save both time and money.
Furthermore, by leveraging AI for early disease diagnosis, potential long-term hospitalizations and expensive, invasive treatments can often be avoided entirely.
Despite its numerous benefits, there are challenges associated with AI implementation in the healthcare industry. Data privacy and security concerns arise when handling sensitive patient information, as cyberattacks can compromise systems and potentially expose critical data.
Ensuring patient anonymity, data encryption, and robust cybersecurity infrastructure are essential to overcome these challenges. Additionally, the integration of AI technology in healthcare requires significant investment in time, infrastructure, training, and research, creating hurdles for smaller healthcare facilities with limited resources.
In recent years, the healthcare industry has experienced a growing interest in harnessing the power of artificial intelligence (AI) to optimize productivity and efficiency. However, this shift also raises concerns surrounding the potential impact on job roles and the need for additional training and reskilling.
As a healthcare enthusiast, it’s crucial to adapt and learn how to integrate AI-powered solutions into daily workflows. At the same time, it’s essential to maintain a nuanced and empathetic approach to patient care. Striking a balance between addressing these challenges and reaping the benefits of improved accuracy, efficiency, and patient outcomes is vital for the successful implementation of AI in healthcare.
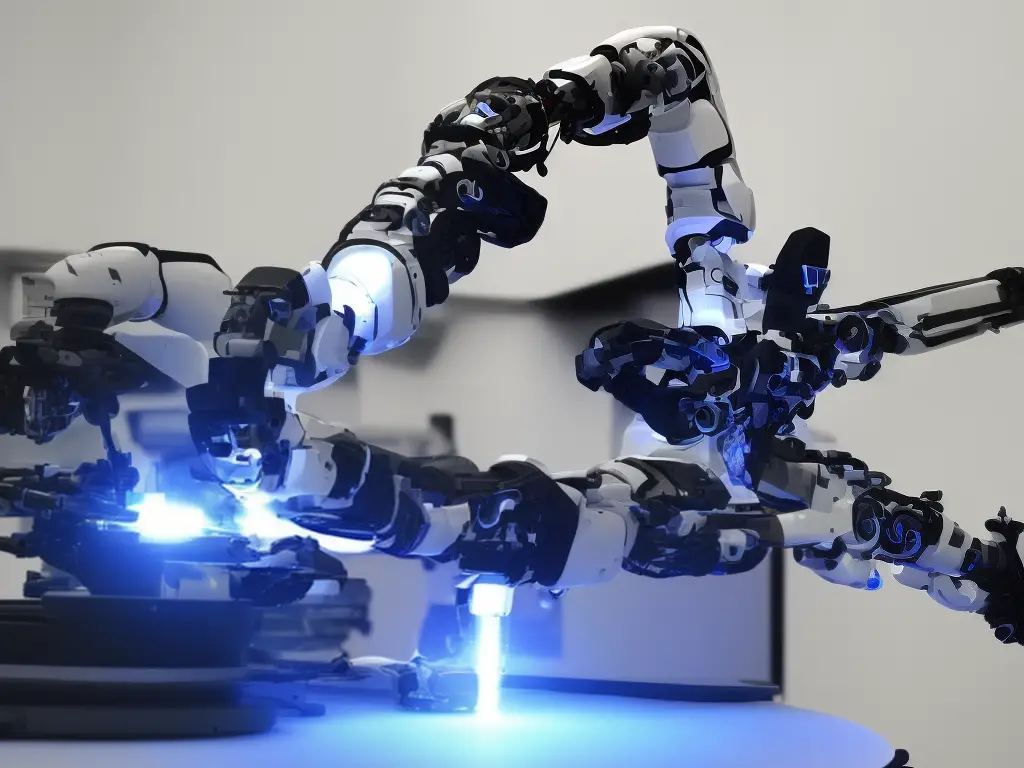
Essential Machine Learning Algorithms of Autonomous AI Agents in Healthcare
One particularly promising area of AI application in healthcare is machine learning algorithms, which are revolutionizing diagnosis and treatment. These algorithms can improve disease detection at early stages and provide personalized treatment recommendations to patients.
As an AI enthusiast, understanding the potential of machine learning in healthcare and developing the skills to work with these algorithms can lead to meaningful contributions to the industry and help shape the future of medical care.
One of the most commonly used machine learning algorithms in healthcare is a decision tree. Decision trees are a type of algorithm that models decisions based on certain conditions. In healthcare, they can be used to create clinical decision support systems that assist physicians in diagnosing diseases or suggesting optimal treatment plans based on patient data like age, gender, and medical history.
Another popular machine learning algorithm is K-nearest neighbours (K-NN). The K-NN algorithm is a simple yet effective method that works by classifying objects based on the majority voting of its K nearest neighbors in a feature space. In healthcare applications, K-NN can be used to detect patterns and classify patient data to identify early signs of diseases such as diabetes or heart disease. The algorithm’s ability to effectively learn from small amounts of data makes it ideal for early disease detection and personalized treatment plans.
Neural networks are another class of machine learning algorithms that have witnessed widespread adoption in healthcare. Inspired by the human brain, neural networks are a set of interconnected nodes or neurons that process and transmit complex patterns of data. These networks can be trained to recognize patterns and make predictions.
Applications of neural networks in healthcare include image analysis for radiology and pathology, drug discovery, and predicting patient outcomes. For example, convolutional neural networks (CNNs) have shown promise in diagnosing skin cancer and detecting lung nodules from CT scans, which helps in early detection and timely treatment.
An additional algorithm that has been making its mark in healthcare is support vector machines (SVM). SVM is a supervised learning method used for classification and regression analysis. It works by constructing a hyperplane in a high-dimensional feature space that separates different classes of data points. In the context of healthcare, SVM has been employed in tasks such as predicting cancer recurrence, identifying Alzheimer’s disease from MRI scans, and diagnosing psychiatric disorders based on brain connectivity patterns.
The integration of Autonomous AI Agents in Healthcare into the healthcare industry has the potential to revolutionize the way diseases are diagnosed, treated, and managed. By incorporating advanced machine learning algorithms, healthcare professionals can create personalized treatment plans based on individual patient’s genetic makeup and health history.
As the field of AI continues to progress, more sophisticated and accurate algorithms are developed, enabling better-informed decisions that can save lives and enhance patients’ quality of life.
Natural Language Processing (NLP) and Healthcare | Autonomous AI Agents in Healthcare
One promising subfield of AI is Natural Language Processing (NLP), which has emerged as a powerful tool for improving healthcare outcomes and optimizing patient care.
By connecting data-driven insights with the linguistic analysis of medical records and healthcare documents, NLP offers novel approaches for the medical community to refine their strategies and provide tailored care for each individual patient.
As healthcare providers continue their transition to electronic health records (EHRs), NLP helps process and analyze large volumes of unstructured clinical data. By extracting valuable insights from raw text, NLP enables better-informed decisions that lead to improved patient outcomes and better medical practices.
One prominent application of NLP in healthcare is the analysis of clinical notes. NLP algorithms can identify essential data elements such as diagnoses, treatment plans, and comorbidities buried within the clinical narrative, leading to better healthcare delivery and improved patient outcomes.
NLP can also process and analyze medical literature, helping healthcare professionals stay abreast of the latest research and ensuring evidence-based medicine. Medical chatbots are a promising area where NLP intersects with healthcare AI solutions, assisting healthcare professionals and providing quick advice to patients.
While AI in healthcare is an area of great potential, challenges like data privacy, accuracy, and mitigation of biases in machine learning models must be addressed to ensure sustainable growth in the industry.
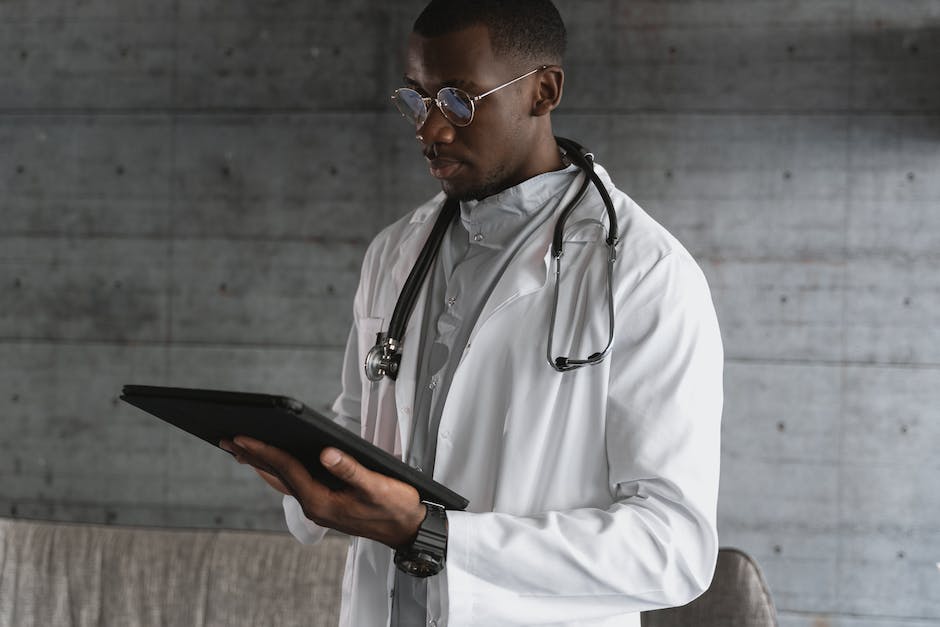
Medical Imaging and Computer Vision | Autonomous AI Agents in Healthcare
Among the various sub-fields of AI, medical imaging and computer vision are rapidly advancing, with the power to transform the diagnosis and treatment of various diseases. This technological progress allows for more seamless integration within the overall healthcare ecosystem.
One of the primary applications of computer vision in healthcare is image segmentation, where AI algorithms are utilized to separate and highlight different structures within medical images. This process is crucial in the identification and analysis of tumours, blood vessels, and other anatomical structures, assisting medical professionals in accurate and efficient diagnosis.
Another significant application of computer vision in healthcare is object detection, which involves identifying and localizing specific objects within medical images. This can be particularly important in detecting and analyzing fractures, cysts, or calcifications in X-ray or MRI scans.
Machine learning algorithms can learn to recognize specific patterns in the vast amounts of data from these medical images, eventually performing at levels comparable to, or even surpassing, human experts.
Image enhancement is a critical component of utilizing computer vision in healthcare, as it allows for improved interpretability of medical images by both AI algorithms and medical professionals. Through techniques such as noise reduction, contrast enhancement, and edge detection, image enhancement algorithms can dramatically increase the clarity of X-ray, MRI, and other medical imaging scans.
This not only provides better visualization of structures within the body but it can also highlight subtle features that could be indicative of disease or injury, leading to more accurate diagnoses and potential early detection.
Aside from these techniques, computer vision has been playing a crucial role in advancing other aspects of AI in healthcare. For instance, the field of augmented reality (AR) has benefited from computer vision by overlaying digital information on physical images, helping surgeons visualize patient data during surgical procedures.
Furthermore, computer-aided diagnosis systems using AI and computer vision have been shown to help reduce human error and improve diagnostic accuracy, particularly in fields such as dermatology, pathology, and radiology.
Medical imaging and computer vision play a crucial role in advancing the effectiveness of AI-powered tools in healthcare. By fostering improvements in image segmentation, object detection, and image enhancement, these technological advancements are transforming the diagnostic process and enhancing patient outcomes.
As AI continues to be integrated further into healthcare, medical professionals will be better equipped to make more accurate and timely decisions, raising the overall quality of care provided.
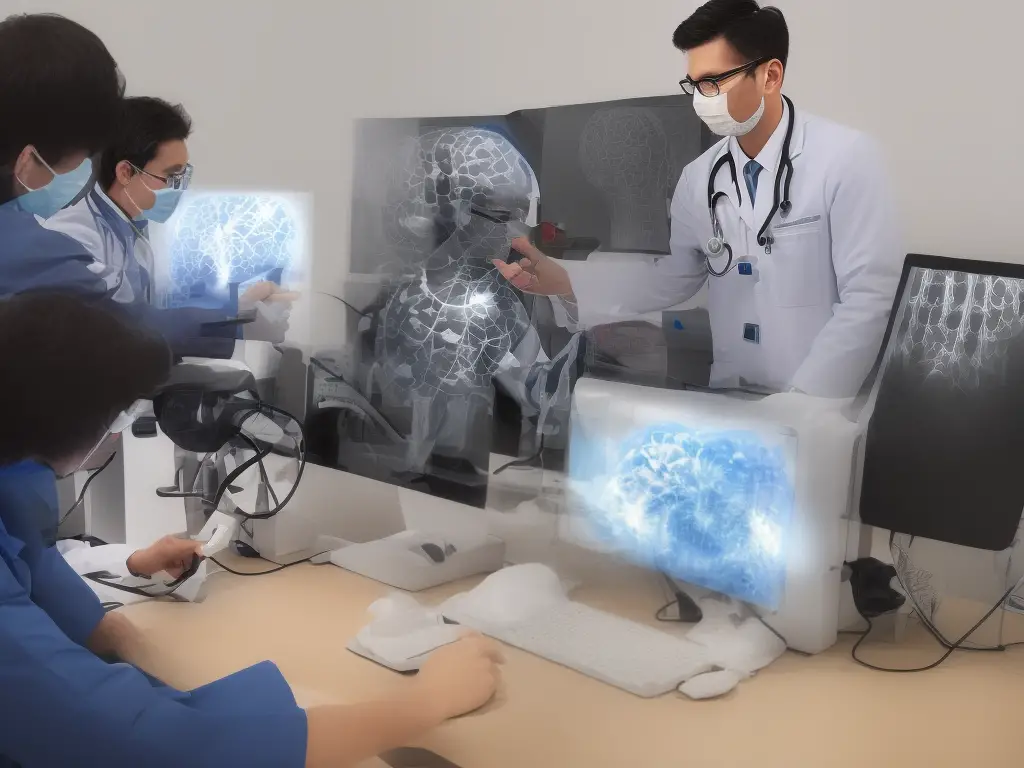
AI Ethics and Privacy in Healthcare | Autonomous AI Agents in Healthcare
As AI increasingly becomes central to revolutionizing the healthcare sector, it carries the potential to not only streamline clinical workflows and optimize healthcare processes but also to improve patient outcomes.
However, as AI in healthcare continues to progress, it also brings to the fore ethical considerations and privacy concerns that must be addressed to safeguard human rights and uphold medical ethics.
Among the many ethical challenges faced in AI healthcare applications, data privacy remains a significant concern. AI algorithms often require large amounts of patient data to function efficiently and accurately.
Ensuring that this sensitive information is securely stored and appropriately anonymized is crucial to protect patients’ rights and maintain public trust in AI technology. Additionally, consent management and data-sharing protocols need to be robust and transparent, allowing patients to have a clear understanding of how their data is being used.
Transparency plays a critical role in AI ethics and healthcare. It is essential that AI systems are designed to be explainable and interpretable to ensure doctors and healthcare professionals can understand and validate the generated results.
Transparent AI can help prevent potential medical errors, facilitate trust between medical practitioners and AI systems, and allow for the smoother integration of these new technologies into everyday clinical practice.
Fairness is another ethical aspect that necessitates attention in AI healthcare applications. AI systems should be explicitly designed to avoid exacerbating existing health inequalities and discrimination. Biases in AI algorithms can lead to unfair treatment or negative outcomes for specific population groups, such as minorities or individuals of low socioeconomic status.
To ensure equitable AI, it is essential to develop and test algorithms using diverse and representative datasets, as well as involve diverse perspectives throughout the AI development process.
Accountability is also of utmost importance when incorporating AI in healthcare. Defining who is responsible for AI-based decisions and outcomes, especially in cases of erroneous or harmful results, remains vital to maintaining public confidence and ensuring justice.
Establishing comprehensive regulatory frameworks and guidelines for AI applications in healthcare can help ensure the technology remains ethically grounded while preserving patient safety, confidentiality, and autonomy.
In conclusion, as AI continues to reshape the healthcare landscape, a comprehensive understanding of the ethical considerations and privacy issues is imperative to ensure the technology’s responsible implementation. By prioritizing transparency, fairness, and accountability, stakeholders can work together to promote the potential benefits of AI in healthcare while preserving public trust and human rights.
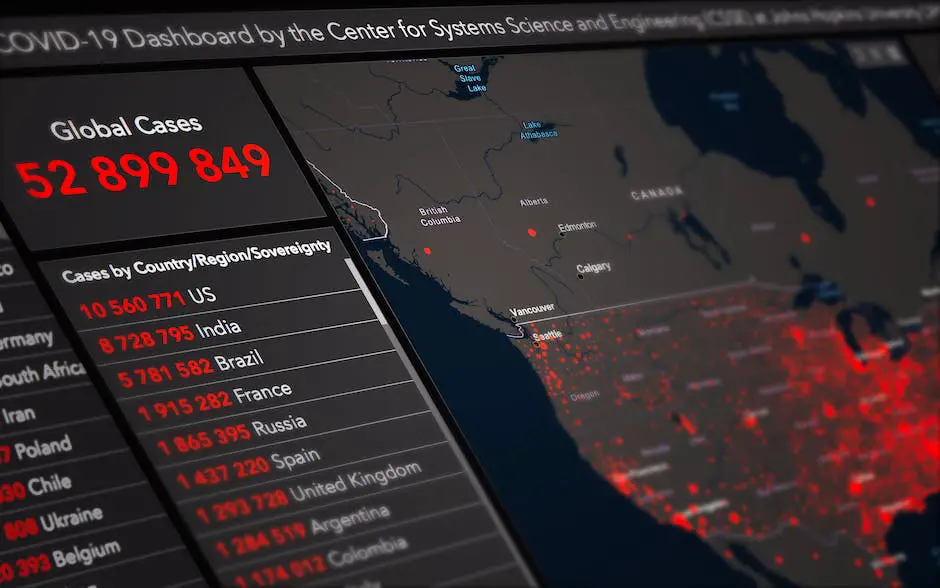
AI-Based Decision Support Systems of Autonomous AI Agents in Healthcare
One of the major ways AI is revolutionizing the healthcare industry is by providing significant support in decision-making. AI-based decision support systems (DSS) have the potential to transform how healthcare professionals diagnose, treat, and manage various medical conditions.
Leveraging advanced machine learning algorithms and vast datasets, AI-powered tools can detect subtle patterns and trends that may be difficult for humans to identify, ultimately leading to improved patient care. By maintaining ethical standards and addressing privacy concerns, we can ensure the widespread adoption of AI in healthcare and maximize its benefits.
One major area where AI-based DSS has made significant strides is an accurate diagnosis. By analyzing medical images, electronic health records, and other clinical data, these systems are able to identify diseases and conditions, such as cancer or diabetic retinopathy, with increased speed and precision.
For instance, AI algorithms have demonstrated the capacity to analyze chest X-rays or mammograms and detect abnormalities with a level of efficacy comparable to that of expert radiologists. This not only reduces the burden on medical professionals but also minimizes the risk of misdiagnosis, improving patient outcomes.
AI-powered decision support systems also play a critical role in developing personalized treatment plans for patients. By utilizing AI and complex data analytics, healthcare professionals are able to consider a wide range of variables, such as patient demographics, lifestyle, and medical history, allowing them to tailor specific interventions and therapies for each individual.
This personalized approach to treatment has proven effective in addressing complex medical conditions, such as cancer and cardiovascular diseases, where one-size-fits-all strategies may be inadequate.
Another important aspect of AI-based DSS in healthcare is the facilitation of personalized medicine. This relatively new field aims to develop customized medical solutions for each individual, taking into account their genetic makeup, environmental factors, and various health indicators.
AI algorithms can analyze genomic data and predict how individuals will respond to certain medications or treatments, enabling healthcare providers to offer targeted personalized medical interventions. In turn, this personalized approach can lead to more effective and efficient healthcare, saving both time and resources.
In conclusion, AI-based decision support systems are revolutionizing the healthcare industry by enhancing the capabilities of medical professionals in diagnosing, treating, and implementing personalized medicine. These AI-powered tools provide valuable insights, making them crucial for improving patient care and achieving better health outcomes.
As AI technology continues to progress and become further integrated into healthcare, these decision support systems will keep evolving, shaping the future of medical practice and patient care.
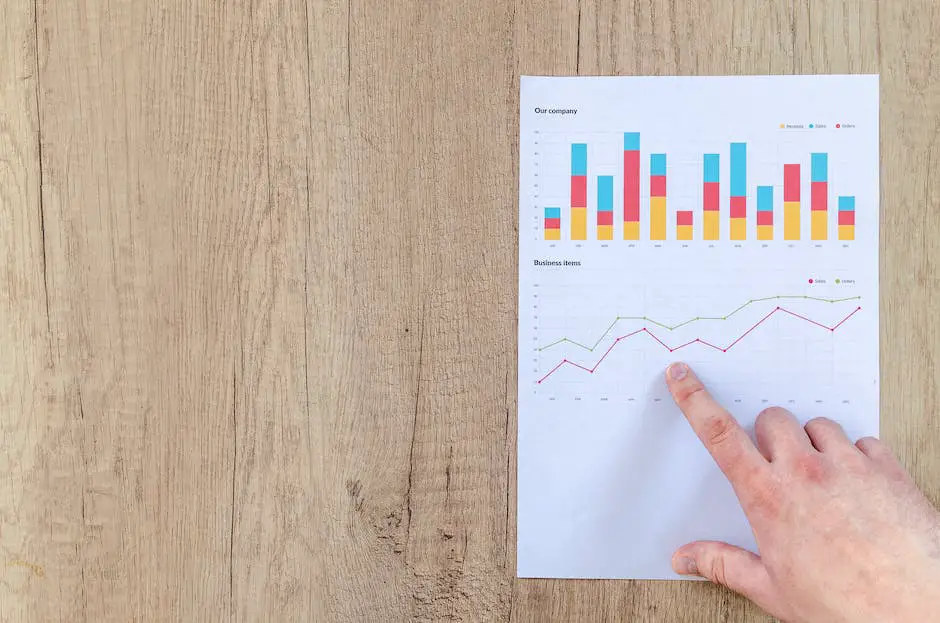
Healthcare Data and Data Preprocessing
For AI healthcare applications, data is a critical driving force that enables the creation and refinement of algorithms, models, and ultimately, the improvement of patient care. There are two primary types of data utilized in AI healthcare applications: structured and unstructured data.
Structured data is information that is neatly organized and formatted, making it easily comprehensible to computers. It is typically stored in databases, tables, or spreadsheets, and in healthcare, examples include patient demographics, lab results, and billing information.
On the other hand, unstructured data is more intricate as it lacks organization and a specific format. Examples of unstructured data in healthcare encompass doctor’s notes, audio recordings, and medical images.
Data preprocessing is an essential step in working with healthcare data, as it helps to improve the quality and accuracy of the dataset. One key aspect of data preprocessing is data cleaning, which involves removing errors, inconsistencies, and inaccuracies from the data.
In healthcare, this might involve correcting typos in patient records, filling in missing values, or resolving discrepancies between data sources. By cleaning the data, AI models can better understand the information and ultimately lead to more accurate predictions and results.
Another important aspect of data preprocessing in AI healthcare applications is data normalization. This technique ensures that data values are on a similar scale, making it easier for AI algorithms to process and compare the values.
Data normalization is particularly crucial in healthcare, as various measurements and values can have different units and scales, such as blood pressure readings and heart rate values. Normalization can include techniques such as min-max scaling or z-score normalization, which help to standardize the data and make it more accessible for AI models.
Data augmentation is another preprocessing technique that can enhance the quality of datasets used in AI healthcare applications. This method involves generating new training samples by applying various transformations to the existing data, such as rotating, flipping, or scaling images.
Data augmentation is commonly used in medical image analysis, as it allows AI models to learn from a more diverse and varied dataset, thus improving their performance and ability to generalize to new data.
One major challenge in AI healthcare is dealing with imbalanced datasets, which occur when certain classes or types of data are over or underrepresented in the dataset. This can lead to biased AI models, as they may not perform well in underrepresented classes.
To tackle this issue, preprocessing techniques such as oversampling, undersampling, or synthetic data generation can be used to balance the dataset and ensure a more reliable and accurate AI model for healthcare applications.
Data preprocessing plays a crucial role in AI healthcare, and by comprehending and implementing these techniques, enthusiasts and hobbyists can develop more effective and dependable AI healthcare solutions.
Deep Learning and Neural Networks in Healthcare
One major connection between AI and healthcare lies in the utilization of deep learning and neural networks, such as convolutional neural networks (CNNs) and recurrent neural networks (RNNs), which have a significant influence on the healthcare industry. These networks have paved the way for a transformative approach to analyzing various medical data and further enhanced AI healthcare’s potential for medical practitioners.
Convolutional neural networks are specifically designed for processing images and have proven to be incredibly effective in extracting and learning patterns and features from massive datasets of medical images.
Recurrent neural networks, on the other hand, have shown particular value in processing time-series and sequential data. This capability makes them well-suited for applications involving medical signal processing.
Deep learning and neural networks have been influential in healthcare, particularly in the realm of natural language processing (NLP). This subfield of AI allows systems to comprehend and process human language, enabling the analysis of electronic medical records (EMRs) and the extraction of valuable information, which leads to improved clinical decision-making.
The integration of deep learning and neural networks into healthcare can enhance various aspects of patient care, such as early disease detection, personalized treatment planning, and advanced clinical decision support. Consequently, healthcare professionals can provide better and more accurate care to their patients. The ongoing advancements in AI technology are anticipated to generate even more groundbreaking innovations in the field, transforming healthcare delivery and ultimately improving patient outcomes.
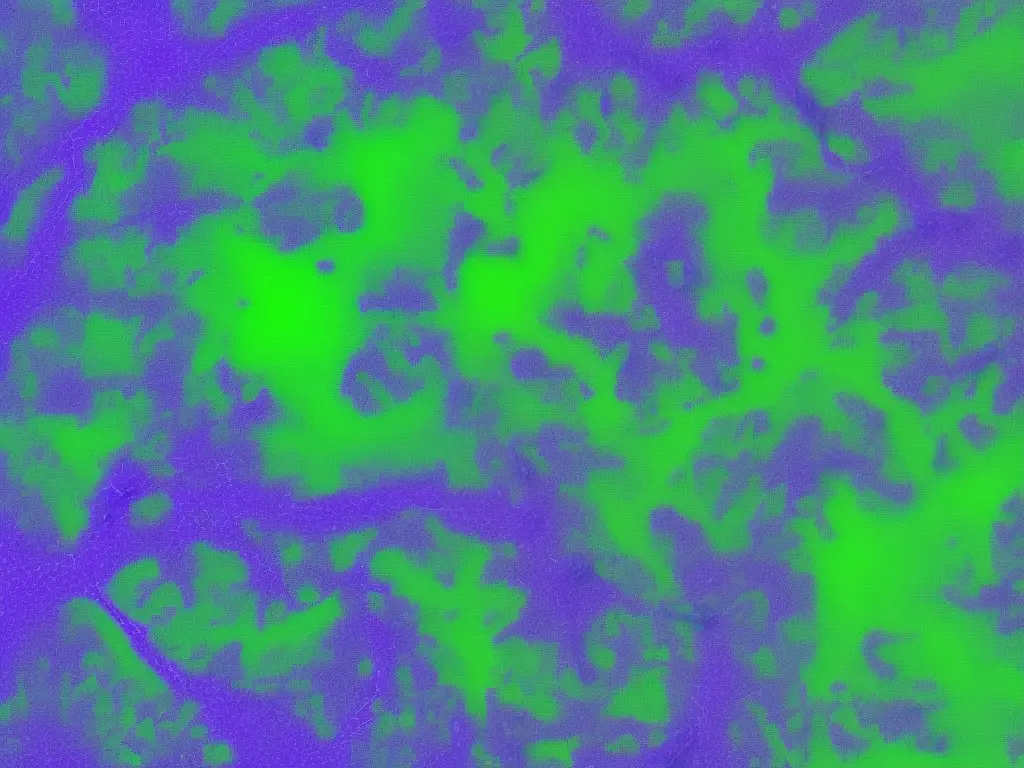
Autonomous AI Agents in Healthcare Case Studies
Artificial intelligence (AI) has demonstrated a plethora of applications and potential in the healthcare sector, positively impacting patient outcomes and revolutionizing the ways in which care providers diagnose and treat various diseases.
By building on the aforementioned applications of AI, such as natural language processing and deep learning, healthcare professionals can continue to innovate and enhance both diagnostic and treatment methods, making the entire healthcare ecosystem more efficient and effective.
One real-world case study of AI implementation in healthcare settings is the collaboration between PathAI and Philips. PathAI algorithms aim to expedite and improve the accuracy of cancer diagnosis by automating the time-consuming task of analyzing pathology images.
This type of AI technology processes the imagery, enabling pathologists to work more efficiently and effectively, ultimately leading to better patient outcomes.
Another notable example of AI technologies being deployed in healthcare is the use of IBM’s Watson. The University of Tokyo, in collaboration with IBM, developed an AI system that aids in diagnosing rare diseases.
AI technology augments the knowledge of doctors by rapidly scanning through large datasets, research articles, and patient records to recommend potential diagnoses with high accuracy.
The Rio Grande do Sul Cancer Registry in Brazil is an example of how AI-powered analytics can be used to compile disease registries, allowing public health professionals to monitor the burden of disease in the population more effectively.
The use of AI in predicting patient risk factors and supporting clinical decision-making is also gaining traction. Google’s DeepMind, for example, developed an AI-assisted system that can predict acute kidney injuries 48 hours before they occur, allowing doctors to intervene and adjust treatment plans proactively. Additionally, Zebra Medical Vision has developed an AI algorithm that can identify high-risk patients for cardiovascular events such as heart attacks, enabling healthcare providers to prioritize and focus on preventive care for at-risk individuals.
However, despite the promising prospects of AI technologies in healthcare, there are several challenges to consider. The accuracy and reliability of the technology rely heavily on the quality and breadth of the data it’s trained on, making it crucial to ensure diverse and representative datasets.
Furthermore, ethical concerns surrounding patient privacy and data security require the establishment of robust policies and regulations. AI technologies must also integrate effectively within existing workflows to maximize their impact on patient care, which necessitates close collaboration between developers and healthcare professionals.
In conclusion, AI adoption in healthcare presents numerous opportunities to improve patient outcomes and streamline the delivery of care. Implementation case studies like PathAI, IBM Watson, and Google’s DeepMind demonstrate the range of applications and benefits that AI technologies can provide.
However, navigating challenges such as data quality, ethical concerns, and integration barriers will be crucial to the continued growth of AI in the healthcare sector.
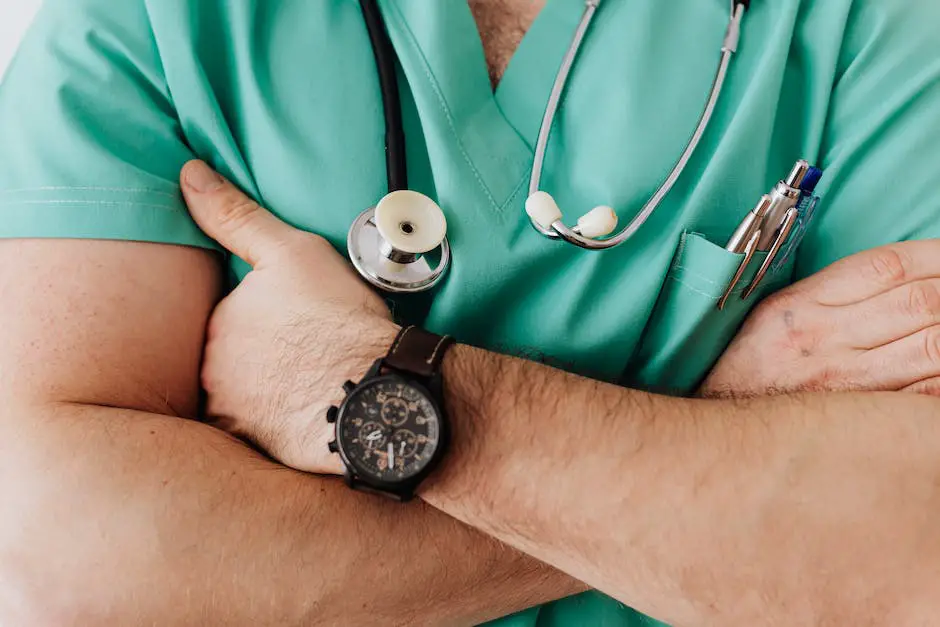
Throughout this essay, we have examined the numerous advancements and breakthroughs delivered by AI in the realm of healthcare. As we navigate the complex world of modern medicine, tools such as machine learning algorithms, natural language processing, and deep learning are proving to be invaluable in revolutionizing healthcare practices.
By understanding the potential benefits, challenges, and ethical concerns surrounding Autonomous AI Agents in Healthcare, we can make informed decisions on leveraging these cutting-edge technologies to drive better patient outcomes and shape the future of medicine in a responsible and effective manner.
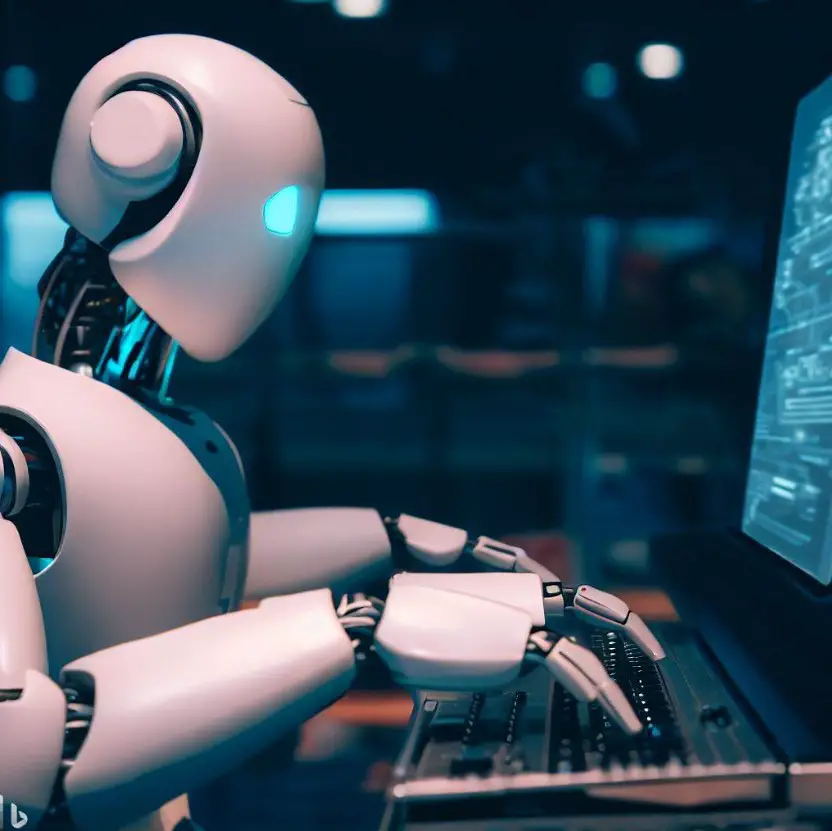
I’m Dave, a passionate advocate and follower of all things AI. I am captivated by the marvels of artificial intelligence and how it continues to revolutionize our world every single day.
My fascination extends across the entire AI spectrum, but I have a special place in my heart for AgentGPT and AutoGPT. I am consistently amazed by the power and versatility of these tools, and I believe they hold the key to transforming how we interact with information and each other.
As I continue my journey in the vast world of AI, I look forward to exploring the ever-evolving capabilities of these technologies and sharing my insights and learnings with all of you. So let’s dive deep into the realm of AI together, and discover the limitless possibilities it offers!
Interests: Artificial Intelligence, AgentGPT, AutoGPT, Machine Learning, Natural Language Processing, Deep Learning, Conversational AI.