Welcome to an in-depth exploration of OpenAI GPT, a groundbreaking technology that has revolutionized the world of artificial intelligence. In this article, we will examine the development of OpenAI GPT, discuss its objectives, and delve into the concepts driving its success in natural language processing.
OpenAI GPT Overview
Table of Contents
OpenAI GPT
OpenAI GPT, or Generative Pre-trained Transformer, is a groundbreaking language model developed by OpenAI, an artificial intelligence research organization. Its development began with the release of GPT in 2018 and has culminated in the most recent iteration, GPT-3, which was released in 2020.
This state-of-the-art language model is designed to perform a wide array of natural language processing tasks, including translation, summarization, question-answering, and much more. The primary objective of the GPT series is to create powerful AI that is not only capable of understanding but also generating human-like text based on a given prompt.
Transformer architecture
The key technology behind OpenAI GPT is the Transformer architecture, which facilitates the efficient processing of large-scale language tasks.
This architecture enables the model to learn contextual relationships between words and phrases, thus improving its capability to generate more contextually relevant responses. The model is pre-trained on massive volumes of text data from the internet, refining its knowledge of language, grammar, and various topics. Unlike other language models, GPT’s generative approach allows it to be fine-tuned for specific tasks by further training on smaller, specialized datasets.
Growth and Advancements in OpenAI GPT
OpenAI’s GPT language models have seen remarkable growth and advancements through various iterations. Specifically, GPT-3 boasts a staggering 175 billion parameters, significantly larger than its predecessor, GPT-2. The combination of increased size and advanced pre-training methods has led to substantial improvements in the model’s performance across a diverse set of tasks.
As OpenAI continues to innovate and develop the GPT series further, it is anticipated that the capabilities of this groundbreaking language model will evolve, pushing the boundaries of natural language understanding and generation to unprecedented heights.
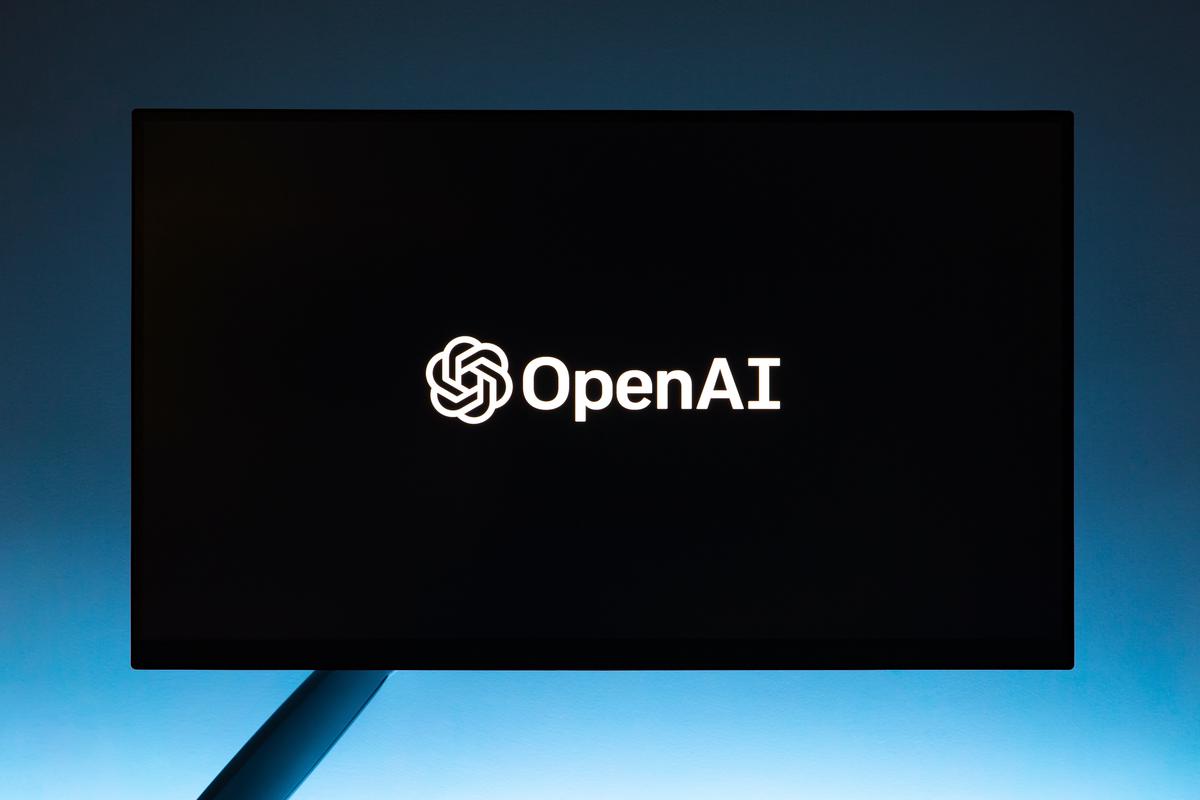
Photo by andrewtneel on Unsplash
GPT Generations and Evolutions
The Evolution of OpenAI GPT: Generations and Challenges
The evolution of OpenAI GPT has witnessed tremendous progress through its generations, culminating in the creation of powerful and versatile AI language models.
GPT-2
The second-generation model, GPT-2, marked a significant leap forward from the original GPT with its ability to generate coherent and contextually relevant text.
Featuring 1.5 billion parameters and trained on 40GB of data, GPT-2 gained widespread attention for its impressive text generation capabilities. However, this also raised ethical concerns about potential negative implications, such as generating convincing fake news articles or deep fakes.
GPT-3
With GPT-3, OpenAI has made further advancements in terms of scalability and capabilities. As the largest language model currently available, GPT-3 boasts 175 billion parameters and is trained on 45 terabytes of diverse data.
This massive scale enables the model to not only produce human-like text, but also understand complex contexts and provide meaningful responses. GPT-3 can perform tasks ranging from translation and content generation to computer programming code completion.
Furthermore, the evolution from GPT-2 to GPT-3 introduced the shift from unsupervised fine-tuning to few-shot learning, enabling the model to quickly adapt to specific tasks with only a limited amount of data samples.
Potential Applications and Challenges
Each successive generation of OpenAI’s GPT models comes with its own potential applications and challenges. Both GPT-2 and GPT-3 have proven to be incredibly powerful text generators, yet unique challenges arise, such as energy consumption during training and possible unintended biases.
Moreover, concerns regarding the misuse of AI-generated content necessitate responsible deployment. OpenAI has begun addressing these issues by sharing research with the community, as well as seeking public input to foster the development of safe and beneficial AI systems. Future enhancements in GPT generations may thus focus on tackling these challenges while simultaneously improving the model’s capabilities and efficiency.
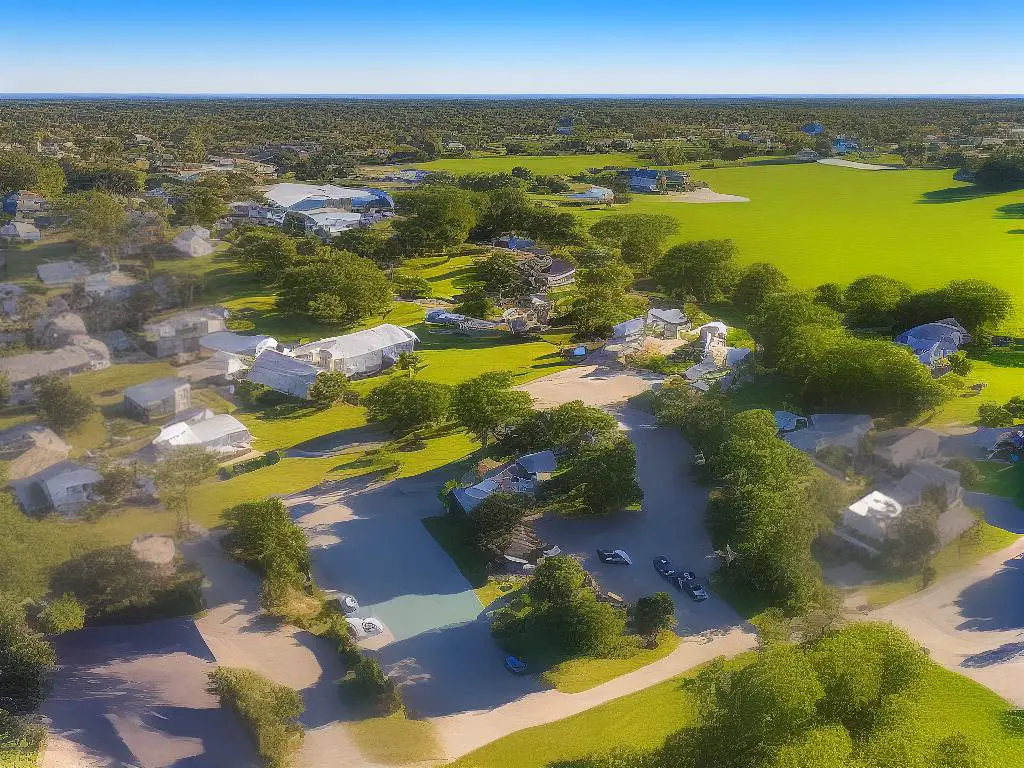
Understanding Transformer Architecture
The Transformer Architecture in Modern NLP Models
The transformer architecture, first introduced by Vaswani et al., serves as the foundation for contemporary natural language processing (NLP) models, including the successful OpenAI Generative Pre-trained Transformer (GPT) series.
The self-attention mechanism lies at the heart of this architecture, enabling the model to efficiently merge diverse context information and discern long-range dependencies within text. Alongside the self-attention mechanism, the transformer architecture employs multi-head attention, which allows it to capture multiple semantic representations at varying scales and assign appropriate weights during the encoding and decoding phases.
The Encoder and Decoder Components of Transformer Architecture
There are two essential components to transformer architecture: an encoder and a decoder. The encoder is responsible for processing the input sequence and generating an intermediate representation, while the decoder module produces an output sequence using the encoded representation. It’s notable that in the GPT models, only the decoder portion of the transformer is used.
The GPT models are designed for unidirectional, or causal, language modeling, meaning that the model can only generate a token by conditioning on the previously generated tokens. This design choice makes GPT models more suitable for tasks where predicting the upcoming tokens or completing given sentences is essential.
Effective Scaling and Training Strategies for GPT Models
The significant success of the GPT models in handling various natural language understanding and generation tasks can be attributed to their effective scaling and the transformer architecture. Increasing the number of layers and attention heads in models like GPT-2 and GPT-3 have showcased their remarkable capabilities.
Furthermore, the pre-training and fine-tuning strategy employed in GPT models enables them to learn an extensive range of language patterns during pre-training, while fine-tuning allows them to specialize in specific tasks. The union of the transformer architecture and a robust training strategy has established the GPT series as the state-of-the-art choice for numerous NLP applications.
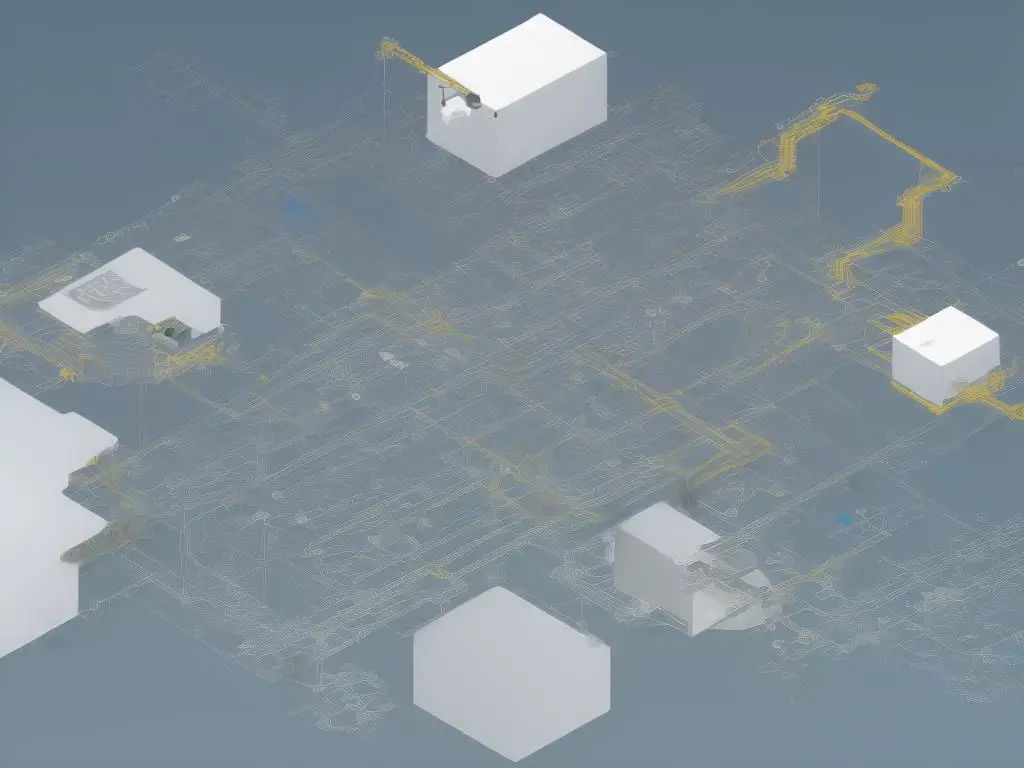
Language Modeling and NLP
Language modeling forms the core of natural language processing (NLP), aiming to accurately represent and predict the nuances and distributions of human language. In the context of OpenAI’s Generative Pre-trained Transformer (GPT), language models lay the groundwork for understanding and generating human-like text.
The extensive training of GPT on large amounts of text data allows the model to learn patterns, grammar, sentence structures, and context.
With the support of the Transformer architecture and deep learning techniques, GPT can efficiently process and analyze textual data, empowering it to predict subsequent words in a sentence while also generating coherent paragraphs. These capabilities contribute to the seamless connection between the GPT models and their language modeling goals.
Attention Mechanism
The effectiveness of GPT lies in its attention mechanism and use of self-attention, which allows the model to focus on different parts of the input when making predictions. This mechanism provides the model with the capacity to discern contextual relationships between words in a sentence, even if they are spaced apart.
Moreover, GPT employs a multi-headed self-attention approach, enabling it to compute multiple attention representations simultaneously. By capturing different contexts and representations of the input, GPT significantly improves its capability in various NLP tasks such as machine translation, summarization, and question-answering.
Fine-tuning
Fine-tuning GPT enables it to excel in specific NLP tasks by adjusting the model’s weights according to the specialized dataset. This process allows GPT to effectively capture nuances and specific knowledge needed for the task at hand. In addition, tokenization techniques, which are employed to separate input into meaningful language representations, play a vital role in improving GPT’s efficiency.
By utilizing subword tokenization methods, GPT reduces the number of tokens it needs to process, leading to better memory usage and computational efficiency. As a result, GPT’s language modeling capabilities greatly impact the frontier of NLP, contributing to the development of advanced AI applications capable of understanding, interpreting, and communicating using human-like language.
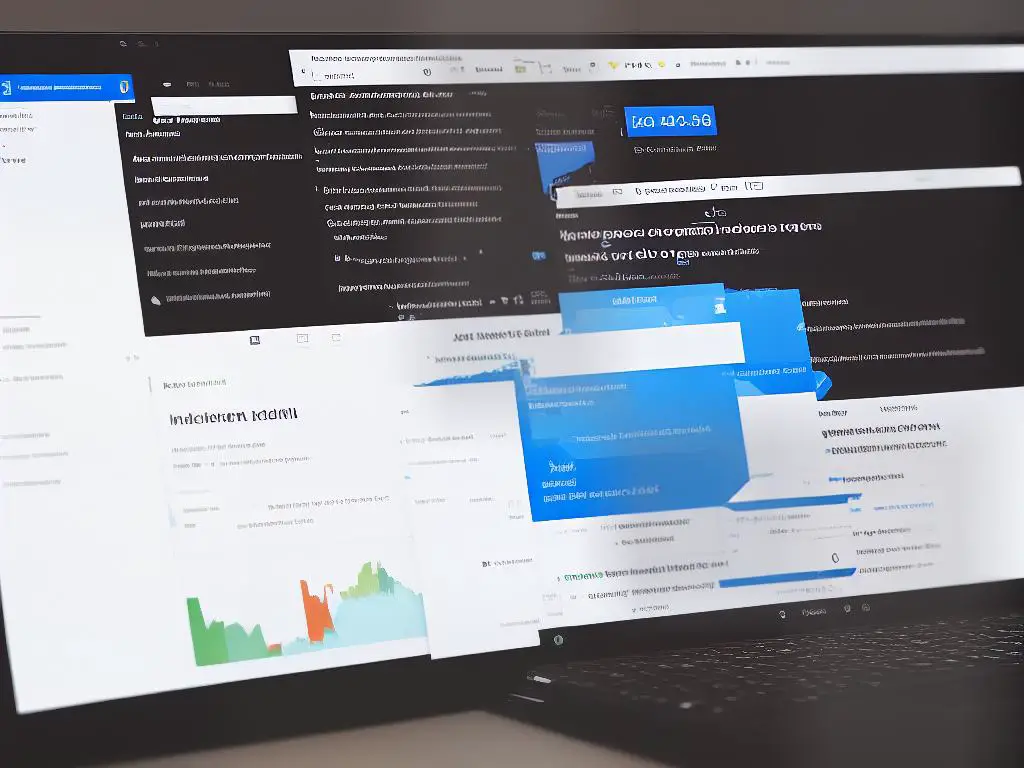
Training Strategies and Datasets
A fundamental aspect of developing powerful language models like OpenAI’s Generative Pre-trained Transformer (GPT) is the thorough data preparation and rigorous training process that goes hand-in-hand with the fine-tuning mentioned earlier. Selecting diverse, rich datasets for pretraining and fine-tuning enables the model to master various linguistic patterns and adapt its performance to a wide array of tasks.
Notable examples of datasets used for GPT models include Books1 and Books2, which incorporate text from thousands of books, and WebText, featuring web pages from numerous online sources. By leveraging such extensive datasets, language models can effectively learn linguistic structures, contextual understanding, and specialized knowledge across an array of domains.
Training strategies for GPT models are designed to optimize their performance and enhance their generalization capabilities. One such strategy is unsupervised pretraining, which exposes the model to vast multilingual data to acquire foundational language skills.
This initial step is followed by supervised fine-tuning, a process that narrows the model’s focus onto specific target tasks with labeled datasets. Techniques like batch normalization, gradient clipping, and learning rate schedules are employed to stabilize the training process and prevent issues such as gradient explosion or vanishing. Moreover, adopting strategies such as transfer learning and leveraging transformer architectures have proven effective for large-scale language models like GPT.
Researchers constantly strive to enhance language models by fine-tuning various aspects, such as model architecture and data preprocessing techniques. The progression from GPT-2 to GPT-3, for example, saw a notable increase in the number of parameters from 1.5 billion to a staggering 175 billion.
This has significantly boosted the capabilities of GPT-3. Crucial changes include improved data cleaning and tokenization, which contribute to the reduction of noise and the better preparation of textual data for training. As the field of natural language processing advances, GPT models and other language models will continue to exhibit improved performance and capabilities.
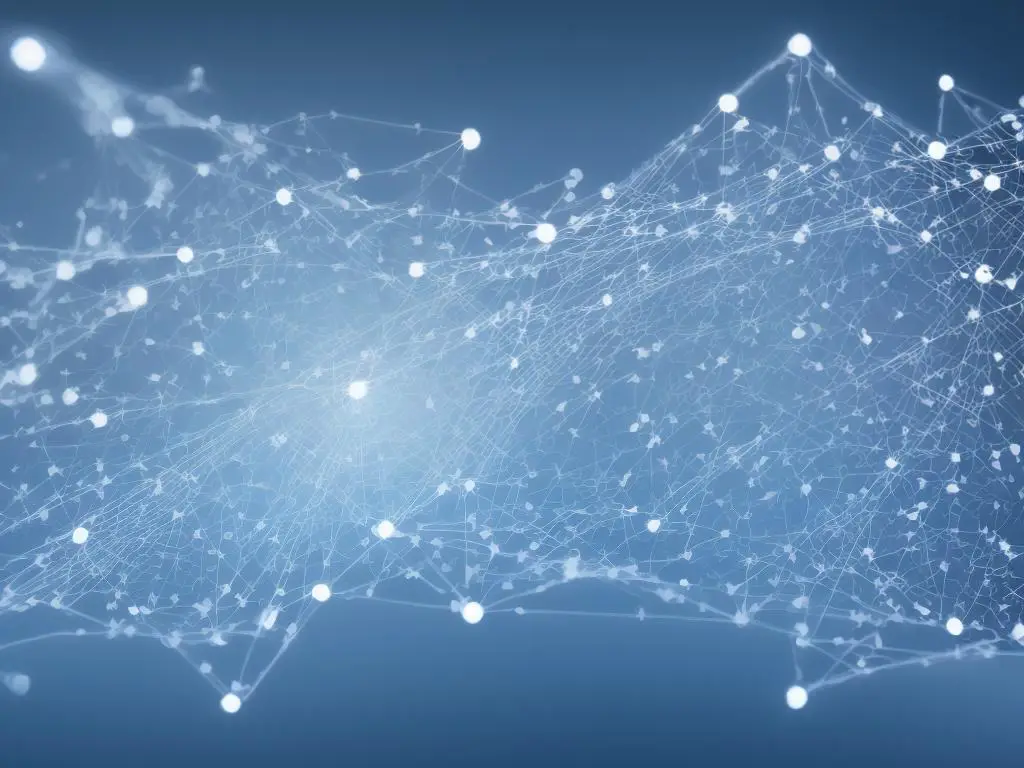
Fine-tuning and Applications
The fine-tuning process is essential for adapting pre-trained GPT models to specific tasks or domains, allowing them to excel in text classification, sentiment analysis, summarization, translation, and more. Fine-tuning involves training the model further using a smaller, task-specific dataset, which equips it with context-specific knowledge.
This leads to more accurate, relevant, and coherent responses from the models. As these fine-tuning techniques are refined, GPT models will become even more effective in catering to the specialized needs of individual tasks and industries.
Across various industries, the applicability of GPT models has been immense. In healthcare, for example, these models can aid medical professionals by providing diagnostic suggestions, navigating through complex medical literature, or synthesizing research articles. In the realm of finance, GPT models can support tasks like market analysis, pattern identification, and even help draft reports on financial trends and stocks. In the legal sector, these models can assist in drafting legal documents, analyzing contracts, or identifying potential compliance issues.
Furthermore, GPT models have also found utility in creative writing, where they can be used for generating story ideas, writing prompts, or even assisting in drafting full-length novels.
The power of GPT models lies in their adaptability to various contexts and industries, making them invaluable assets in today’s data-driven world. As technological advancements continue, the potential for fine-tuning and repurposing GPT models in previously unexplored fields only grows, solidifying their indispensable role across diverse domains.
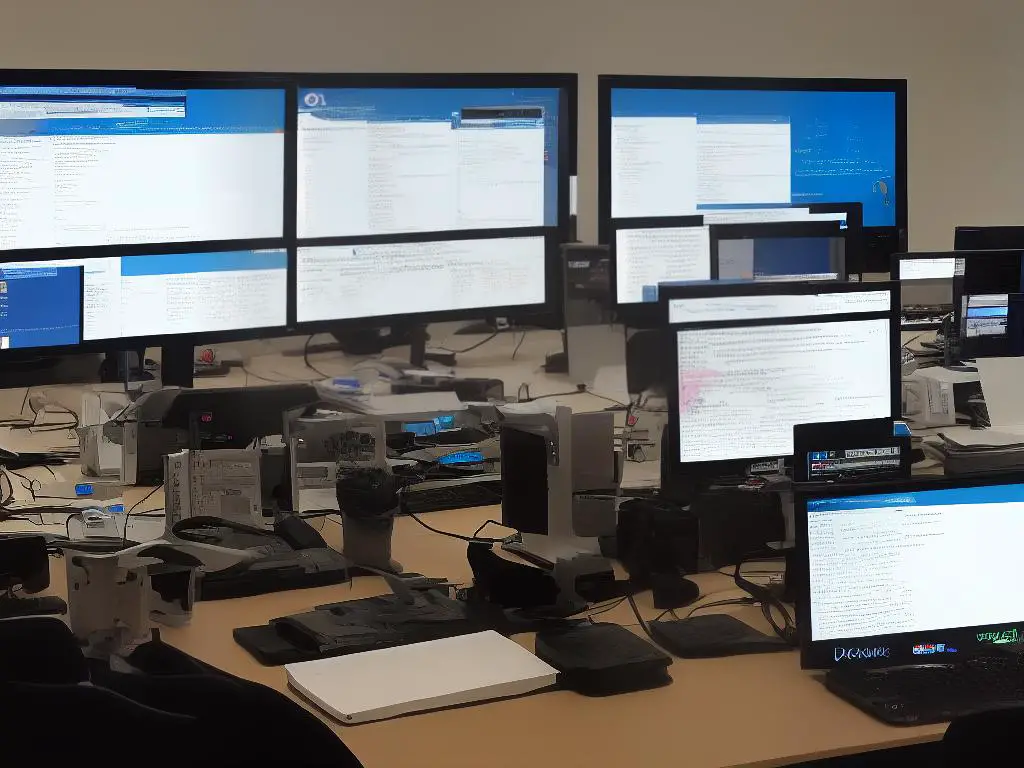
Limitations and Ethical Concerns
However, GPT models do have significant limitations, particularly in regard to output biases that can raise ethical concerns. Since these models are trained on vast quantities of internet text, they may inadvertently learn and perpetuate biases found within the source data, such as gender, racial, and political biases, among others specific to different contexts.
As GPT models become increasingly integrated into applications like chatbots and content generation, it’s crucial that we address and mitigate these biases to ensure users aren’t misled or influenced by prejudiced AI-generated content.
Another concern surrounding GPT is the propagation of false information or manipulation. As GPT becomes more advanced and widely implemented, there is a risk of using the technology to create convincing but misleading content that can potentially influence public opinion, spread misinformation, or even fuel disinformation campaigns.
To address this, it is vital to invest in research efforts that focus on detecting AI-generated content and mitigating such risks from being abused. In addition, educating users on the limits of AI-generated content will be crucial in fostering critical thinking and skepticism when consuming content generated by GPT.
As GPT models are becoming more powerful and have a growing influence on various sectors, a critical aspect to consider is the societal impact of such applications. Concerns have been raised about the potential job displacement these models might cause as a result of automating tasks previously done by humans, particularly in the realm of content generation.
Additionally, there is a risk of unintended consequences that may arise from using GPT in decision-making, filtering, or behavior prediction applications where biases and inaccuracy can have more profound implications on individuals and communities. It is imperative to assess and address these ethical concerns as we integrate GPT and similar AI models into our daily lives to ensure a fair, responsible, and beneficial application of the technology for all.
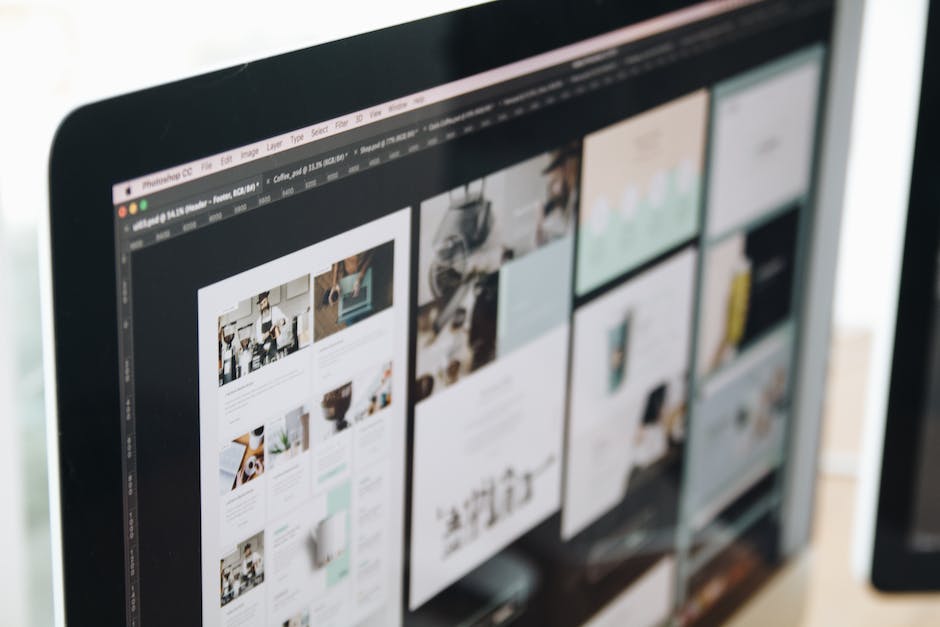
Throughout this article, we’ve embarked on a journey through OpenAI GPT’s intricate world, discussing its growth, architecture, training, and applications. As we’ve discovered, this technology offers tremendous potential across numerous industries, but it’s crucial that we remain aware of its limitations and ethical implications. With continued research and development, OpenAI GPT has the power to transform the way we interact with and understand artificial intelligence.
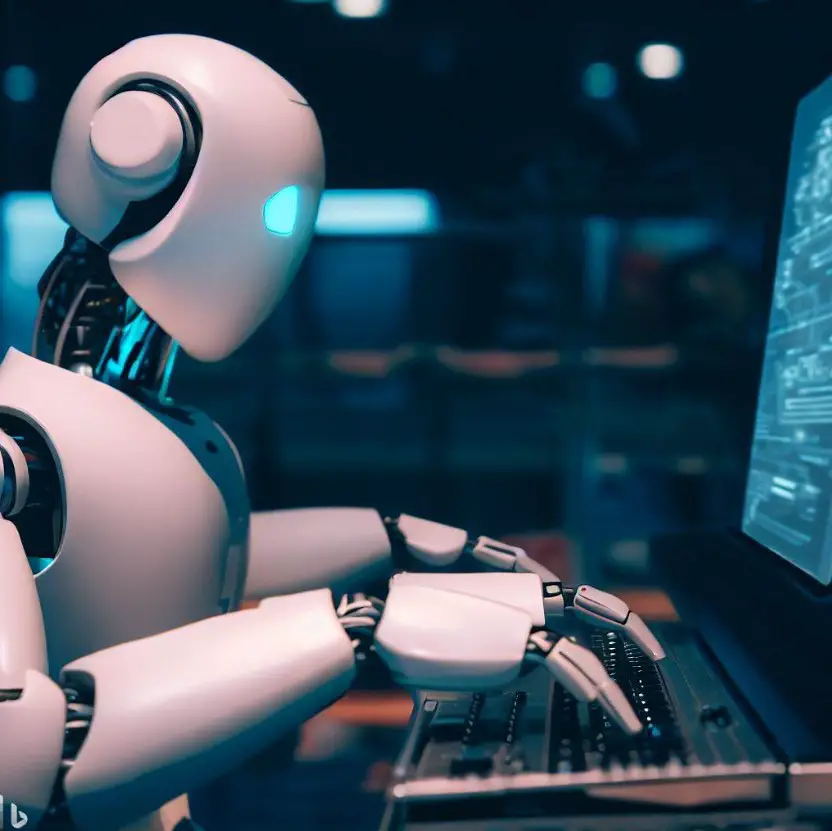
I’m Dave, a passionate advocate and follower of all things AI. I am captivated by the marvels of artificial intelligence and how it continues to revolutionize our world every single day.
My fascination extends across the entire AI spectrum, but I have a special place in my heart for AgentGPT and AutoGPT. I am consistently amazed by the power and versatility of these tools, and I believe they hold the key to transforming how we interact with information and each other.
As I continue my journey in the vast world of AI, I look forward to exploring the ever-evolving capabilities of these technologies and sharing my insights and learnings with all of you. So let’s dive deep into the realm of AI together, and discover the limitless possibilities it offers!
Interests: Artificial Intelligence, AgentGPT, AutoGPT, Machine Learning, Natural Language Processing, Deep Learning, Conversational AI.