As innovations in artificial intelligence continue to accelerate, the AgentGPT model is gaining recognition as a groundbreaking advancement in the field of AI. This transformative technology has the potential to redefine the way we approach natural language processing, improving efficiency and opening up new possibilities across a range of industries. In this essay, we’ll dive deep into the intricacies of AgentGPT, examining its architecture, training methods, real-world applications, ethical concerns, and the potential prospects and challenges it presents to the AI landscape.
Understanding AgentGPT
Table of Contents
- 1 Understanding AgentGPT
- 2 Optimizing and Training AgentGPT
- 3 Practical Applications of AgentGPT
- 4 Ethics and Responsible AI Development
- 4.0.1 Ethics and Responsible AI Development in AgentGPT Reworked AI
- 4.0.2 Ethical Concerns and Potential Misuse
- 4.0.3 Addressing Biases and Limitations
- 4.0.4 Transparency and Public Input in Regulatory Processes
- 4.0.5 Best Practices for Avoiding Unintended Consequences
- 4.0.6 Exploring the Potential of AgentGPT Reworkd AI
- 5 Future Prospects and Challenges for AgentGPT
Introduction to AgentGPT
AgentGPT is a recent development in the field of artificial intelligence (AI) that has emerged as a powerful natural language processing (NLP) model. Built on the foundational principles of the GPT (Generative Pre-trained Transformer) model, it has been tailored to suit the needs of open-ended applications, providing human-like communication and interaction.
This article discusses the fundamental concepts behind the AgentGPT model, including its architecture, key components, and essential features that distinguish it from other AI technologies.
Transformer Architecture
The underlying architecture of AgentGPT is based on the transformer model, a deep learning system that has revolutionized NLP in recent years. Transformers are unique in their ability to handle parallel computation effectively, owing to their reliance on the self-attention mechanism. This mechanism allows the model to focus on different parts of the input text to determine relationships among words, leading to a better understanding of the context.
Unlike traditional recurrent neural networks (RNNs) that sequentially process input data, transformers enable parallelization of computation through a series of stacked layers. Each layer consists of multi-head self-attention mechanisms and feed-forward networks that work together to effectively capture the syntactic and semantic structure of text. Consequently, transformer models like AgentGPT can process larger amounts of data more efficiently – a vital feature for handling complex NLP tasks.
GPT Design
AgentGPT is an extension of the GPT architecture, which is a generative and pre-trained model that focuses on unsupervised learning. GPT models are fine-tuned on vast amounts of text data, effectively “learning” the structure and nuances of human language, which is a key reason behind their remarkable performance in various NLP tasks.
The GPT model employs a unidirectional approach, processing input text from left to right. This design choice intends to predict the most likely next word in a sequence, producing coherent and contextually accurate text. As such, AgentGPT can engage in complex conversations and generate text that closely resembles human-generated content.
Role of Tokens
One of the primary components in the AgentGPT model is the concept of tokens. Tokens are the basic units of text representation used by the model to process and generate text efficiently. Typically, a token corresponds to a single word, subword, or character. However, in the case of AgentGPT, the model leverages much larger tokens that dynamically adjust to the input text.
The tokenization process allows the model to efficiently represent and manage input data, effectively handling the complexities of human language. The choice of token granularity is a crucial factor in the model’s performance.
Smaller tokens may increase the model’s ability to capture detailed nuances but may also require higher computational resources, while larger tokens may result in faster processing times but limit the model’s capacity to capture fine-grained language patterns. AgentGPT aims to strike an optimal balance between the two by using dynamic tokens suited to the particular context.
Differentiation from Other AI Technologies
AgentGPT stands out from other AI technologies in several ways. Firstly, it leverages the power of transformers, which offer parallel computation capabilities, making it more efficient in handling large volumes of text data. Secondly, it builds upon the generative and pre-trained nature of GPT models, giving it the unparalleled ability to adapt to various NLP tasks effectively. Lastly, the unique tokenization method used in AgentGPT allows for greater flexibility and adaptability, as the model adjusts tokens to suit the context of the input data.
Introduction
AgentGPT represents a powerful and versatile AI model for natural language processing, capable of revolutionizing the way humans and machines interact. By leveraging the GPT architecture and incorporating advanced mechanisms such as self-attention and dynamic tokenization, AgentGPT has become an essential tool for handling complex language tasks. Its adaptability and broad applicability make it perfect for applications such as conversational AI, language translation, and content generation. As the AI field continues to advance, models like AgentGPT will play an increasingly crucial role in driving innovation and improving human-machine communication.
Optimizing and Training AgentGPT
Optimization and Training of AgentGPT
To reach its full potential, AgentGPT must be effectively optimized and trained, allowing it to excel in a wide range of applications, including answering questions, accomplishing tasks, and generating content. This comprehensive guide will examine various optimization techniques, training methodologies, and data requirements essential for achieving the best performance from an AgentGPT model. Ensuring AgentGPT’s efficiency and accuracy will ultimately lead to even greater success in tackling complex language challenges and further enhancing our understanding of AI capabilities.
Optimization Techniques
Optimization is a critical aspect of developing an effective AgentGPT model. Various optimization techniques can be employed to improve its performance, reduce computational requirements, and minimize the risk of overfitting. Some of the widely used optimization techniques include:
- Gradient Descent Algorithms: Gradient descent and its variants, such as Stochastic Gradient Descent (SGD), Adam, and Adagrad, are commonly used to update the model’s weights based on the gradients of the error function. These methods help in achieving faster convergence and minimizing the loss function effectively.
- Learning Rate Scheduling: Adjusting the learning rate during the training process is crucial to control the trade-off between convergence speed and training stability. Techniques like step decay, exponential decay, and cosine annealing are useful for adaptively changing the learning rate as the training progresses.
- Regularization: Techniques like L1 and L2 regularization, dropout, and batch normalization can help reduce overfitting and improve the model’s generalizability by introducing constraints and penalties to the optimization process.
- Architecture Search: Efficiently searching for the optimal model architecture using methods like Neural Architecture Search (NAS) can help strike a balance between model complexity and performance.
Training Methodologies
Effective training methodologies can ensure that the AgentGPT model accurately understands and generates human-like text. Some key training methodologies include:
- Transfer Learning: Pre-training AgentGPT on a large corpus of text data helps it capture general language features, which can then be fine-tuned on domain-specific data for better task-specific performance.
- Curriculum Learning: Gradually exposing AgentGPT to increasingly complex tasks can significantly enhance its learning capability and robustness. This method involves sorting training samples based on their complexity and then training the model on simpler tasks before gradually increasing the difficulty.
- Data Augmentation: Generating new training samples by applying transformations that preserve the meaning of the original data can help improve AgentGPT’s performance, especially in low-data scenarios. Techniques like synonym replacement, back-translation, and paraphrasing can be employed for data augmentation.
- Active Learning: Iteratively selecting the most informative and challenging samples for training can greatly improve the model’s performance and reduce the amount of labeled data required.
Data Requirements
The quality and quantity of data used for training AgentGPT directly impact its performance. Essential data requirements for an effective AgentGPT model include:
- Diversity and Representativeness: The training data should be diverse and representative of the target task’s domain and distribution to ensure the model generalizes well.
- Quality and Relevance: High-quality and relevant training data help AgentGPT produce accurate and meaningful responses and minimize biases.
- Size of the Training Dataset: A larger and more comprehensive training dataset allows the model to learn more complex patterns and handle a wider range of tasks.
- Balanced Data: Ensuring a balanced dataset in terms of topics, styles, and difficulty levels can help AgentGPT become proficient in various tasks with minimal biases.
Conclusion
In conclusion, the effective optimization and training of AgentGPT are essential components in achieving optimal performance, efficiency, and accuracy across a wide range of tasks. By implementing the right optimization techniques and training methodologies, as well as ensuring that data requirements are met, industry experts can develop highly proficient AgentGPT models while minimizing computational demands. Continuous improvements in these strategies will pave the way for the development of even more powerful and efficient language models in the future.
Practical Applications of AgentGPT
Healthcare Applications
One industry that can greatly benefit from AgentGPT’s state-of-the-art language model is healthcare. With its advanced natural language processing capabilities, AgentGPT can analyze patient records, extract critical medical information, and process data to improve patient care. Furthermore, it sets the stage for numerous applications that can revolutionize healthcare practices.
For instance, AgentGPT can facilitate the creation of personalized care plans and recommendations. By inputting patient data, healthcare professionals can obtain treatment options based on a comprehensive understanding of the patient’s medical history. This approach not only saves time but also minimizes the risk of neglecting important details.
In addition, AgentGPT can generate easily understandable medical summaries or translate complex medical terms for patients, helping them grasp their diagnoses and treatment plans more effectively. The AI can also support researchers in conducting literature reviews and data analysis, leading to faster advancements in medical research.
Financial Applications
AgentGPT can provide value to the finance industry in several ways. In investment management, it can analyze financial reports, news articles, and market data to generate insights, thereby aiding investment professionals in making informed decisions. This AI-driven approach can lead to improved investment strategies and risk management.
In personal finance management, AgentGPT can act as a virtual financial advisor. By processing user inputs, it can provide personalized budgeting advice, savings recommendations, and debt management strategies tailored to an individual’s financial situation. Additionally, it can be used by banks and other financial institutions as a tool for customer service, responding to user queries and providing necessary information more efficiently than traditional service representatives.
Educational Applications
AgentGPT’s practical applications in education are vast. As an intelligent tutoring system, it can offer personalized learning experiences for students. By analyzing a student’s performance, strengths, and weaknesses, AgentGPT can provide targeted study materials and practice exercises, helping learners to overcome specific challenges.
Furthermore, AgentGPT can be employed as a virtual teaching assistant, easing the workload of instructors by responding to students’ questions and grading assignments. As an AI-powered writing assistant, it can also help students improve their writing skills by providing feedback on grammar, style, and clarity.
In higher education, AgentGPT’s advanced text-generation capabilities can aid research by summarizing articles or suggesting potential research topics and thesis statements. This reduces the time spent on administrative tasks, allowing scholars to focus on the creative aspects of research.
Customer Service Applications
AgentGPT has significant potential to revolutionize customer service across various industries. As a virtual assistant, it can engage with customers in natural language, understanding their needs, and providing helpful solutions. This not only reduces wait times for customers but also saves companies resources on personnel costs.
Integration of AgentGPT into customer relationship management systems can further boost customer satisfaction levels. By accessing customer data, the AI can deliver personalized support, addressing specific concerns more effectively. Furthermore, it can be employed to create tailored marketing materials, dynamically adjusting product recommendations or promotional offers based on a customer’s preferences and purchase history.
Exploring the Potential of AgentGPT Reworked AI
As an industry expert, understanding the applications and capabilities of AgentGPT Reworked AI is crucial in today’s dynamic landscape. The versatility and potential impact of this reworked AI can be seen in industries like healthcare, finance, education, and customer service. As integration progresses and prototypes and success stories emerge, the value of AgentGPT will continue to unfold, reshaping our approach to information generation and task automation across various sectors.
Ethics and Responsible AI Development
Ethics and Responsible AI Development in AgentGPT Reworked AI
Given the implications of employing AgentGPT Reworked AI across different industries, it is essential to consider the ethical aspects and responsible development of this technology. Rapid advancements in artificial intelligence (AI) and machine learning have raised several challenges, such as inherent biases, limitations, and potential unintended consequences. In this enlightening discussion, we will delve into the ethical issues surrounding AgentGPT, addressing best practices for ethical AI development and avoiding pitfalls in its utilization.
Ethical Concerns and Potential Misuse
One of the primary ethical concerns associated with AgentGPT Reworkd AI and similar models is their potential misuse. These powerful language models can potentially be used to generate high-quality, misleading, or harmful content. This includes generating fake news, automated disinformation campaigns, or even promoting extremist ideologies. Additionally, AI-generated content could be used to manipulate opinions, facilitate unauthorized access to sensitive information, or challenge intellectual property rights, thus raising legal and ethical challenges.
Addressing Biases and Limitations
A significant limitation of the AgentGPT Reworkd AI model is the potential presence of biases. Since AI models learn from extensive datasets consisting of text data from the internet, they might inadvertently adopt existing cultural, racial, or gender prejudices present in the training data.
Consequently, the generated text could further perpetuate these biases, leading to unfair treatment of or discrimination against certain groups. Training AI models on more diverse and inclusive datasets, removing inappropriate content, and using advanced techniques for bias detection and mitigation can help minimize biases and limitations.
Transparency and Public Input in Regulatory Processes
Transparent development processes and public input in AI regulations are key components of responsible AI development. Ensuring transparency implies a consistent sharing of information about the design, development, and functionality of AI models like AgentGPT Reworkd AI.
This allows users to better understand the capabilities and limitations of these models, which in turn fosters trust and well-informed decision-making. Encouraging public participation in regulatory processes is vital to develop inclusive and comprehensive policies regarding AI use.
By involving a wide range of stakeholders, including government, private sector, academia, and civil society, fair and balanced decisions can be made regarding AI development that take ethical considerations into account.
Best Practices for Avoiding Unintended Consequences
Implementing best practices is crucial to minimize unintended consequences and foster ethical AI development. AI researchers and developers need to collaborate to establish guidelines and assessment metrics for AI models that prioritize ethical considerations.
Developing clear policies on AI use and sharing, setting up AI ethics boards, and regularly auditing AI performance can help organizations mitigate risks. Moreover, fostering educational initiatives and developing ethical AI curricula can contribute to raising awareness of the challenges associated with AI use and ethical problem-solving.
Exploring the Potential of AgentGPT Reworkd AI
As an industry expert, it is essential to understand the powerful potential of language models like AgentGPT Reworkd AI and recognize the paramount importance of ethical concerns and responsible AI development. By implementing best practices and fostering collaborations among stakeholders, the AI community can work together to maximize the benefits of AI development while minimizing potential risks and unintended consequences. Transparency and public participation are key in mitigating potential misuse, biases, and limitations.
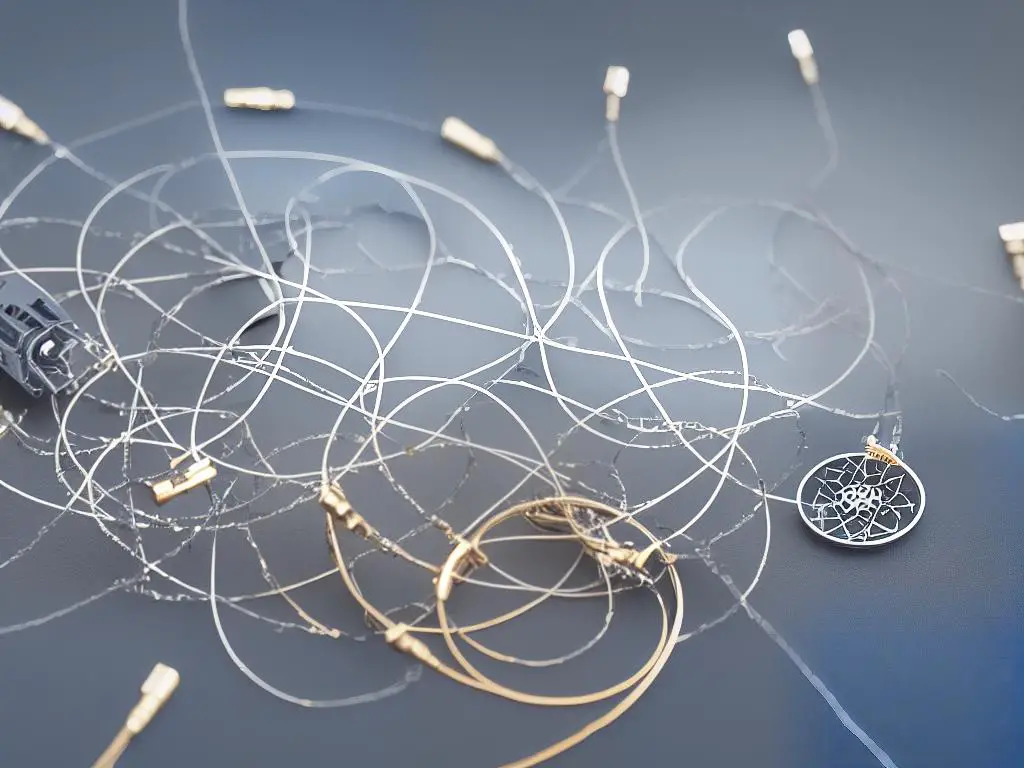
Future Prospects and Challenges for AgentGPT
Anticipated Advancements and Their Impact
Building on the solid foundation of ethical development, AgentGPT is a language model poised for continual improvements in natural language processing capabilities, user engagement, domain adaptation, and content generation.
As the model evolves, researchers expect to see key advancements that will allow AgentGPT to better understand context, emotions, and intentions, producing results that are more accurate and relevant to users’ needs. These exciting prospects have the potential to transform the landscape of AI applications and reinforce the significance of responsible AI development.
Challenges in the Development of AgentGPT
Despite the impressive advancements, there remain several challenges that researchers and developers need to address to further improve the AgentGPT model. Some of these challenges include:
- Ethical concerns: The AI’s ability to generate human-like text raises questions about how it may be used maliciously, such as in spreading misinformation, impersonation, or biased output. Addressing these concerns requires developing guidelines and ethical frameworks for the use and deployment of AgentGPT technology.
- Bias and discrimination: Like most AI systems, AgentGPT can unintentionally acquire and perpetuate human biases present in the training data. As the technology evolves, researchers need to work on mitigating these biases and ensuring that the generated content is fair, unbiased, and inclusive.
- Computational resources: The development and training of powerful AI models like AgentGPT require substantial computational resources. Access to these resources may be limited for some researchers, leading to disparities in advancements within the field.
Potential Breakthroughs and Collaborations
Collaboration between AI experts and professionals from various industries is crucial for realizing the full potential of AgentGPT technology. By working together, professionals can identify niche applications, optimize the technology accordingly, and use the solution to address complex industry challenges.
Bridging the gap between AI research and real-world applications involves forming partnerships between academia, research institutions, governments, and private industry partners. Recovery.ai, for example, has emerged as a leading platform for research and collaboration in AI, and GPT-based models have seen widespread adoption in various industries, from healthcare to financial services to creative writing.
Support Systems for Growth and Adoption
The growth and adoption of AgentGPT technology across numerous industries is dependent on several support systems, including:
- Infrastructure: The development and deployment of AI solutions require significant investment in hardware and software infrastructure. Regional and national governments, alongside private entities, play a critical role in facilitating and funding support.
- Education and training: The development and implementation of AI solutions require skilled professionals educated in the field. Governments and educational institutions must support and invest in AI-related education and reskilling programs to ensure a robust workforce capable of driving AI innovation.
- Regulations and policies: Fostering AI adoption across industries requires clear and supportive regulations, policies, and standards. Governments need to work closely with researchers and industry leaders to create conducive environments for AI development while addressing the ethical and societal concerns associated with its use.
Conclusion
As AgentGPT technology matures and evolves, we can expect it to be at the forefront of AI development, despite its challenges and the ongoing collaboration required to ensure ethical and responsible use. The continuous advancements in this field, propelled by cooperation between industries, academia, and governments, will no doubt lead to exciting new applications and more efficient outcomes for businesses and end-users alike.
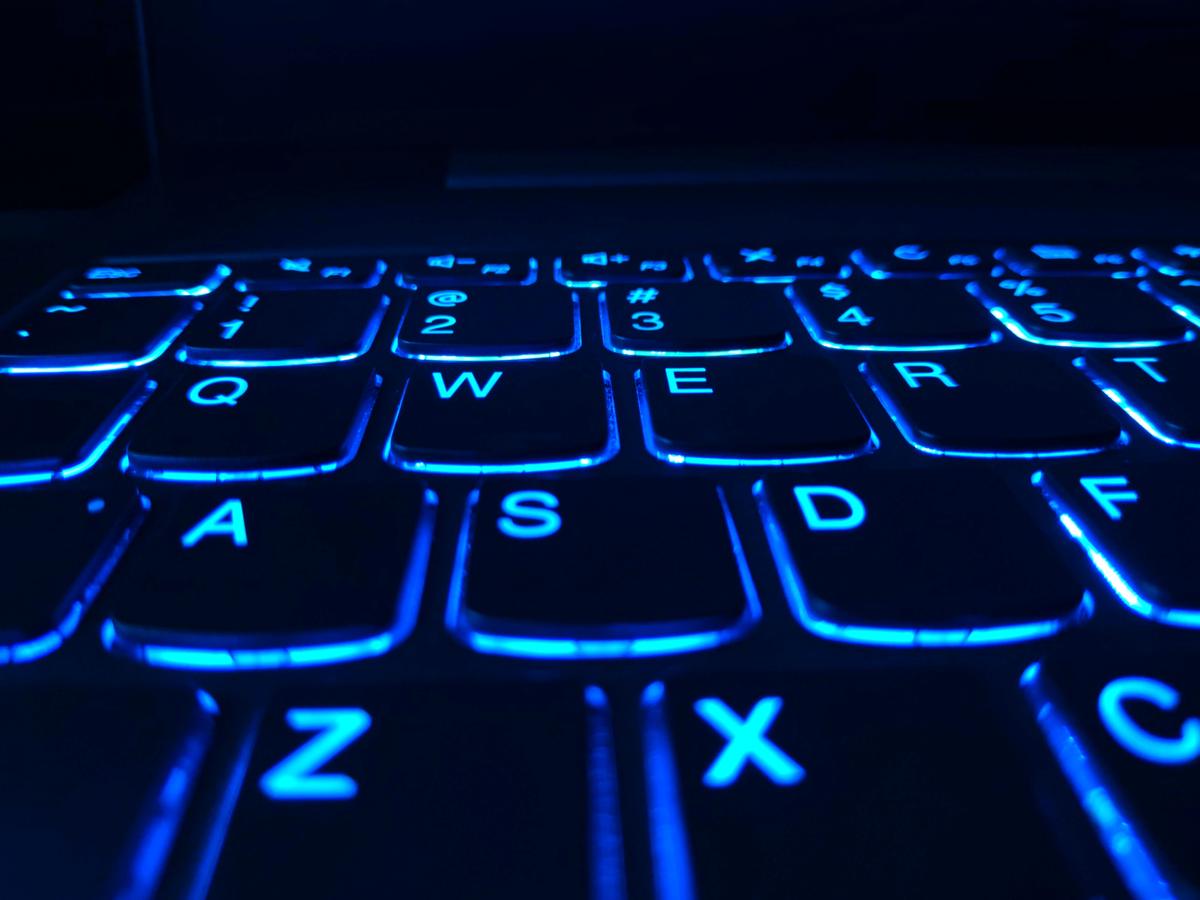
Throughout our exploration of AgentGPT, we have uncovered the ingenuity and potential of this powerful AI model. From its advanced architecture to its real-world applications and ethical considerations, AgentGPT represents a significant leap forward in natural language processing and AI development.
As we look to the future, the success of AgentGPT and similar models will hinge on our ability to advance research, address challenges, and forge collaborative partnerships across industries. By continuing to innovate responsibly and build on the foundation laid by AgentGPT, we can expect to see remarkable advancements that will reshape the way we live, work, and communicate.
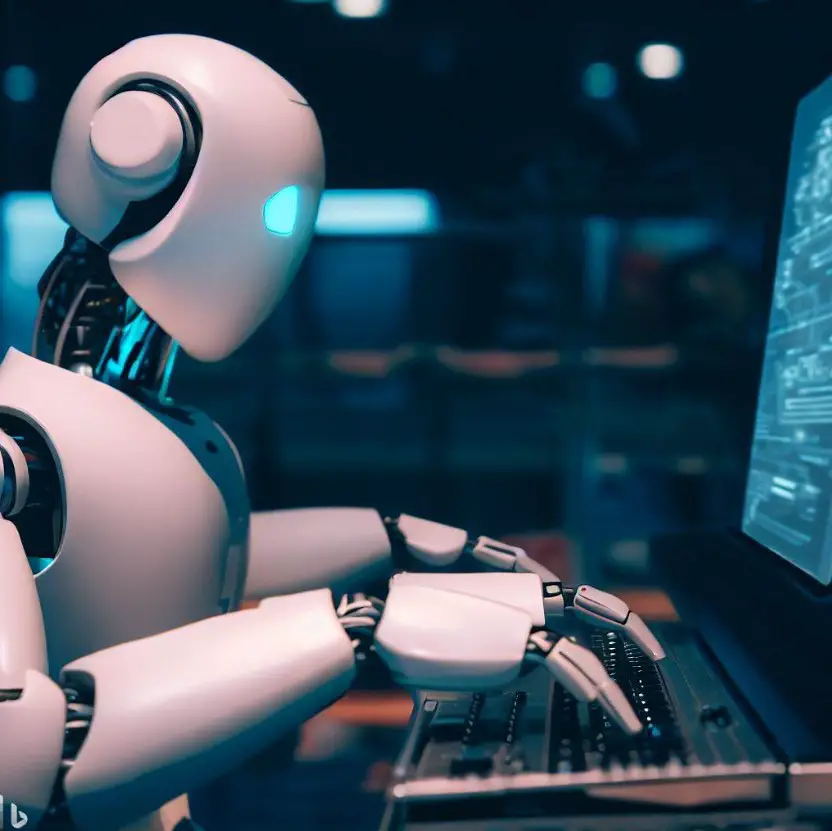
I’m Dave, a passionate advocate and follower of all things AI. I am captivated by the marvels of artificial intelligence and how it continues to revolutionize our world every single day.
My fascination extends across the entire AI spectrum, but I have a special place in my heart for AgentGPT and AutoGPT. I am consistently amazed by the power and versatility of these tools, and I believe they hold the key to transforming how we interact with information and each other.
As I continue my journey in the vast world of AI, I look forward to exploring the ever-evolving capabilities of these technologies and sharing my insights and learnings with all of you. So let’s dive deep into the realm of AI together, and discover the limitless possibilities it offers!
Interests: Artificial Intelligence, AgentGPT, AutoGPT, Machine Learning, Natural Language Processing, Deep Learning, Conversational AI.