As the world continues to embrace technological advancements, the manufacturing industry is experiencing a significant transformation with the integration of artificial intelligence (AI). This revolutionary shift is paving the way for improved efficiency, cost reduction, and enhanced safety measures within various manufacturing processes. By exploring the different AI technologies, their benefits, associated challenges and risks, as well as the concept of Industry 4.0 and potential future trends, we can gain a comprehensive understanding of the role AI is playing in shaping the future of manufacturing.
AI Technologies in Manufacturing
Table of Contents
Introduction to AI Technologies in Manufacturing
As AI technologies continue to advance, they are becoming increasingly relevant in the manufacturing industry. From optimizing production processes to improving product quality, AI technologies offer various benefits that help businesses save time, reduce costs, and increase efficiency. In this article, we will discuss the key AI technologies influencing the manufacturing sector: machine learning, computer vision, robotics, and natural language processing. We will also explore their specific applications in the industry, such as predictive maintenance, quality control, and automated material handling.
Machine Learning in Manufacturing
Machine learning is a subset of artificial intelligence that focuses on enabling computers to learn from data and improve their performance without being explicitly programmed. In the context of manufacturing, machine learning can be applied to a wide range of tasks, including:
- Predictive maintenance: Machine learning algorithms can analyze vast amounts of data generated by sensors in machinery and equipment to detect anomalies, identify patterns, and predict when failures are likely to occur. This enables manufacturers to schedule maintenance activities more efficiently, reduce downtime, and minimize the likelihood of unexpected failures.
- Process optimization: By analyzing historical process data and real-time sensor data, machine learning can identify optimal process parameters, such as temperature, pressure, and flowrate, to maximize product quality and minimize waste.
- Demand forecasting: Machine learning can help manufacturers better understand customer demand patterns, allowing them to optimize production schedules, manage inventories, and ultimately, improve customer satisfaction.
Computer Vision in Manufacturing
Computer vision is an AI subfield that focuses on enabling computers to process, analyze, and interpret visual information from the real world. In manufacturing, computer vision can be used for the following purposes:
- Quality control: Automated inspection systems powered by computer vision can detect defects and irregularities in products with high accuracy, ensuring high quality and minimizing wastage.
- Robotics and automation: Computer vision enables robots and other automated systems to navigate and interact with their environment, improving efficiency and productivity in various production tasks, such as assembly and material handling.
- Safety monitoring: Computer vision can be used to monitor production facilities for potential safety hazards, such as leaks, fires, or unauthorized personnel, helping to prevent accidents and protect employees.
Robotics in Manufacturing
Robotics is the interdisciplinary branch of AI that involves the design, construction, operation, and application of robots and other automated systems. In manufacturing, robotics plays a crucial role in automating repetitive, labor-intensive, and hazardous tasks, thus increasing productivity and reducing the risk of injury. Robotics can be used in various applications, such as:
- Material handling: Robots with advanced gripping capabilities can efficiently transport parts, materials, and products within the manufacturing facility, improving the overall efficiency of the production process.
- Assembly: Industrial robots can be used to automate complex assembly tasks, significantly increasing precision, productivity, and consistency while reducing labor costs.
- Welding, painting, and machining: Specialized robots can perform intricate tasks like welding, painting, and machining with high precision and speed, ensuring exceptional product quality.
Natural Language Processing in Manufacturing
Natural language processing (NLP) is an AI subfield that focuses on enabling computers to understand, interpret, and generate human language. In the manufacturing industry, NLP can facilitate more effective communication between humans and machines, improving overall efficiency. Some applications of NLP in manufacturing include:
- Maintenance and troubleshooting: Natural language interfaces can help technicians access the relevant information and instructions to perform maintenance tasks or troubleshoot issues in machinery more efficiently.
- Supply chain management: NLP can be used to analyze textual data, such as emails and documents, to identify potential supply chain disruptions or risks, helping manufacturers take proactive measures to ensure smooth operations.
- Knowledge management: NLP can be used to extract information from unstructured data sources, such as documents, emails, and reports, making it easier for employees to find relevant information and make data-driven decisions.
Conclusion
In conclusion, AI technologies are revolutionizing the manufacturing industry by offering various benefits, from predictive maintenance and quality control to automation and improved communication. By embracing these technologies, manufacturers can enhance their operations, reduce costs, and meet customer demands more effectively, ensuring a competitive edge in this dynamic and fast-changing sector.
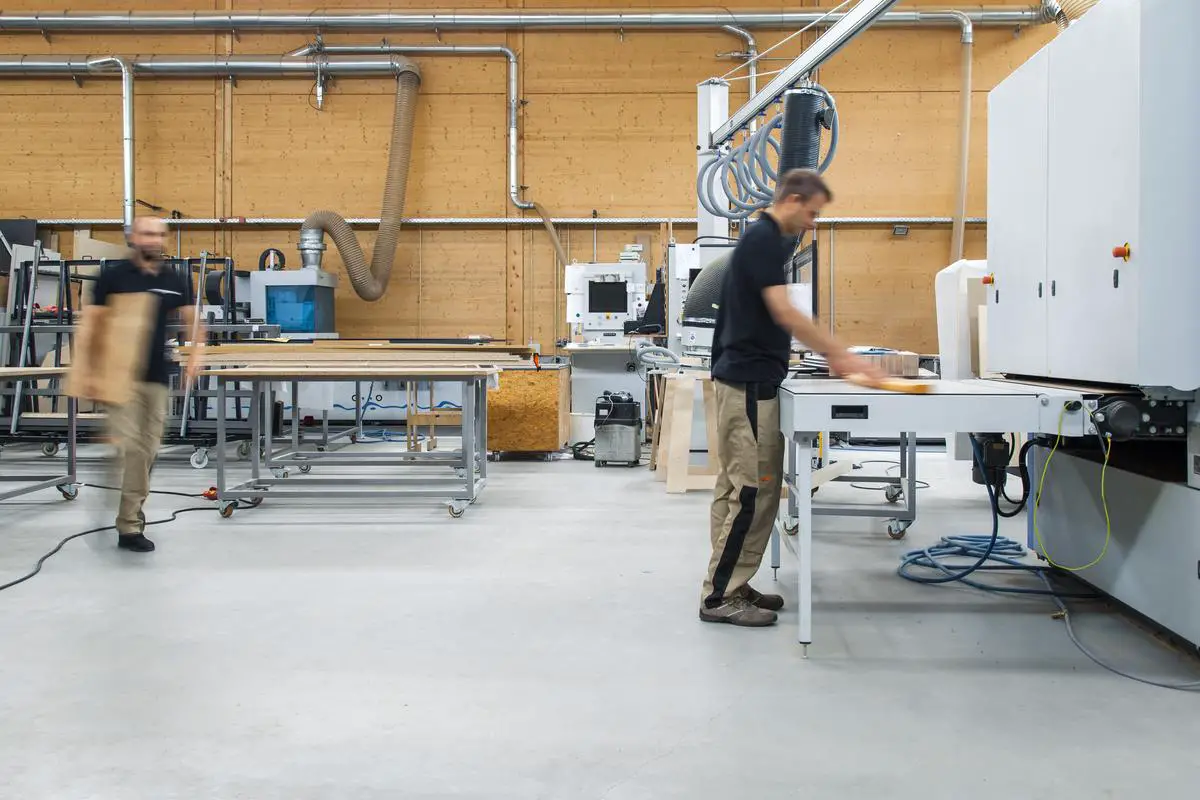
Photo by pickawood on Unsplash
Benefits of AI in Manufacturing
Building upon these innovations, the integration of artificial intelligence (AI) into manufacturing processes has been a game-changer for the industry, providing numerous benefits across the board. As technology continues to advance, the adoption of AI-driven techniques is becoming increasingly popular, with companies seeking to capitalize on the potential advantages it offers.
The following sections will delve into the key aspects and advantages of implementing AI in manufacturing processes, and how they collectively contribute to creating a smarter and more efficient production environment.
Improved Efficiency and Productivity
One of the most prominent benefits of incorporating AI into manufacturing processes is the marked improvement in efficiency and productivity. AI-powered automation enables machines to perform tasks more rapidly and accurately than their human counterparts. This increase in efficiency results in higher production levels and reduced lead times, ultimately improving the overall performance of the manufacturing plant. Moreover, with machine learning capabilities, AI-driven systems can analyze vast amounts of data and make data-driven decisions in real-time, further streamlining the production process.
Reduced Production Costs
By automating various aspects of the manufacturing process, AI can significantly reduce overhead costs. For instance, the implementation of AI-driven machinery often leads to a decrease in labor expenses, as fewer workers are required to manage and operate the equipment. Additionally, AI can optimize energy consumption, leading to lower energy bills for the manufacturing facility. Furthermore, AI-powered predictive maintenance tools can detect potential equipment failures early on, allowing manufacturers to address issues before they result in costly downtime.
Improved Product Quality
Another advantage of implementing AI in manufacturing processes is the notable enhancement of product quality. AI-driven systems can analyze data collected during the production process, identifying inconsistencies and deviations from set parameters. By spotting these irregularities, manufacturers can quickly address any quality issues, resulting in less product waste, reduced costs, and improved customer satisfaction.
Enhanced Worker Safety
The incorporation of AI in manufacturing processes has also had a profound impact on worker safety. By automating hazardous tasks or utilizing AI-driven tools to guide workers through dangerous tasks, the risk of workplace injuries is significantly reduced. Moreover, AI-powered wearable devices can monitor workers’ biometrics and detect signs of fatigue or stress, alerting both employees and supervisors to potential safety concerns.
Real-Life Examples and Case Studies
Many case studies exist to demonstrate the benefits of AI implementation in the manufacturing industry. For example, Siemens, a global powerhouse in the fields of industry, energy, and healthcare, has developed a self-optimizing gas turbine system using AI. This system monitors itself, diagnoses issues, and adjusts its settings accordingly, significantly reducing maintenance costs and increasing overall efficiency.
In another case, General Motors utilizes AI-powered robotics in their manufacturing plants. These robotic arms are designed with embedded cameras and machine learning capabilities, enabling them to perform a wide range of tasks, such as welding and assembly. As a result, productivity has increased, and workplace injuries have significantly decreased.
The extraordinary potential of artificial intelligence (AI) in manufacturing
Artificial intelligence (AI) has been making waves in the manufacturing industry, thanks to its remarkable ability to improve efficiency, reduce costs, enhance product quality, and promote worker safety. As an educator or teacher interested in developing proficiency in AI applications in manufacturing processes, you have a unique opportunity to help shape the future of the industry and empower the next generation of innovators to thrive in a rapidly evolving market.
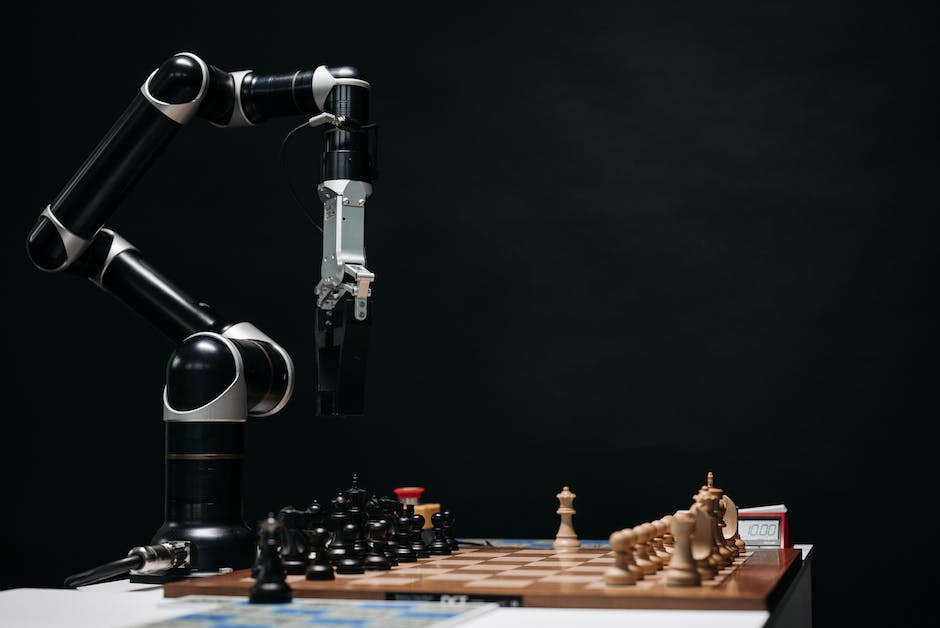
Challenges and Risks in AI Adoption
Understanding challenges and risks in AI-powered manufacturing
Although the benefits of AI in manufacturing are significant, it is also essential to consider the potential challenges and risks associated with AI adoption, such as job displacement, data security, and ethical concerns.
In this article, we will delve into these critical issues as well as the difficulties in integrating AI systems with existing infrastructure, emphasizing the importance of employee retraining as part of the transition to AI-driven manufacturing processes. By recognizing and addressing these challenges, educators and teachers can provide comprehensive guidance and skills development to students, equipping them for success in an AI-enhanced manufacturing landscape.
Job Displacement
Arguably one of the biggest concerns around AI adoption in manufacturing is the potential displacement of human jobs. The rise of AI-driven systems and robotics could lead to the automation of many tasks traditionally carried out by workers, particularly those involving routine and repetitive activities. This can lead to significant job losses, increasing unemployment rates, and exacerbating socioeconomic inequalities. To address this concern, companies and governments alike must consider strategies to transition workers to new positions and provide them with the skills necessary to succeed in an increasingly automated world.
Data Security
As AI systems rely heavily on data to function, it is essential to address the issue of data security. Enormous quantities of sensitive information, such as employee data, intellectual property, and trade secrets, may be at risk if proper measures are not put in place to protect this valuable resource. Cybersecurity threats, including cyber attacks, data breaches, and espionage, are becoming more sophisticated, requiring organizations to constantly review and update their security measures. This includes investing in robust data protection systems and conducting regular security audits to ensure the integrity of data.
Ethical Concerns
The use of AI-driven technologies in manufacturing also raises ethical questions around transparency, accountability, and fairness. Ensuring that AI algorithms function in a fair and unbiased manner can be challenging, particularly given that they are often designed based on vast amounts of data that may contain underlying biases. As such, organizations must be vigilant in identifying and addressing potential bias in their AI systems, as well as ensuring that they adhere to applicable regulations and ethical standards.
Integrating AI Systems with Existing Infrastructure
The integration of AI into established manufacturing infrastructures can be a complex and costly process, particularly given the need to retrofit or replace existing equipment to accommodate new technologies. Organizations must carefully consider the financial, logistical, and operational implications of embracing AI and plan for the long-term impacts of such investments. This may require extensive collaboration between AI experts, engineers, facility managers, and business leaders to ensure a smooth transition.
Employee Retraining
The implementation of AI in manufacturing requires a significant shift in the skills necessary for employees to excel in their roles. As AI systems take over repetitive tasks, the demand for human creativity, problem-solving, and decision-making grows.
Organizations must invest in retraining initiatives to equip their workforce with the skills necessary to work alongside AI technologies, including upskilling and reskilling programs in areas such as data analysis, programming, and machine learning. In doing so, businesses can ensure they have the human capital needed to capitalize on the vast potential of AI-driven manufacturing.
Introduction
With the emergence of AI in manufacturing processes, a new era of industrial transformation is upon us. This technological revolution, known as Industry 4.0, promises vast improvements in efficiency, productivity, and innovation for manufacturers.
As educators and teachers, it is crucial to become proficient in AI and its applications within the manufacturing landscape. By understanding and addressing the challenges and risks that lie ahead, such as job displacement, data security, ethical concerns, integration with existing infrastructure, and employee retraining, we can ensure the responsible and efficient adoption of AI in manufacturing processes.
Industry 4.0 and Smart Factories
Industry 4.0 and Smart Factories
Building upon this notion, Industry 4.0 and smart factories serve as prime examples of AI-driven advancements in the manufacturing sector. Through the integration of innovative technologies such as the Internet of Things (IoT), big data analytics, and robotics, highly connected, automated, and data-driven smart factories have emerged. For educators and teachers looking to become proficient in AI within manufacturing processes, these developments present a wealth of opportunities for knowledge acquisition and practical application, as well as various challenges and obstacles to be navigated.
The Rise of Industry 4.0
Industry 4.0 is characterized by the integration of digital systems in traditional manufacturing processes, resulting in increased efficiency and autonomy. The term “Industry 4.0” was coined in 2011 by the German government to describe the ongoing technological advancements in industrial production.
These advances include the integration of the cyber-physical systems (CPS), which enable machines and humans to interact through digital networks in real-time. By leveraging AI, machine learning, and big data, Industry 4.0 aims to improve production processes, reduce waste, and increase overall operational efficiency.
AI and Machine Learning in Industry 4.0
AI and machine learning play a critical role in the development of smart factories within Industry 4.0. With the help of AI-powered systems, manufacturers can analyze data from production lines to predict equipment failures, detect patterns, and optimize production processes. Machine learning algorithms can also enhance quality control by analyzing images or video feeds to detect defects, minimizing human involvement in quality assurance tasks.
Moreover, AI-driven technologies facilitate advanced robotics solutions that can work autonomously or in collaboration with humans. These solutions enable improved productivity, optimized utilization of resources, and increased safety for employees on the factory floor.
Internet of Things (IoT) and Big Data Analytics
The Internet of Things (IoT) is another essential aspect of Industry 4.0, enabling smart factories to connect devices, sensors, and machines through a centralized network. These connected devices generate a vast amount of data during production, which can be analyzed using big data analytics to discover new insights, optimize processes, and improve decision-making.
Big data analytics in smart factories can be used to monitor and manage resources, identify bottlenecks, improve energy efficiency, and optimize supply chain operations. Furthermore, the combination of IoT and big data analytics offers increased visibility into the overall manufacturing process, enabling real-time monitoring and agile adjustments to production plans.
Workforce Implications and the Role of Educators
While Industry 4.0 and smart factories offer numerous benefits, they also pose challenges for the workforce. Automation of manual tasks and increased demand for skilled employees proficient in AI, IoT, and analytics require a shift in education and training within the manufacturing sector.
Educators and teachers play a critical role in preparing the workforce for smart factories by developing curricula focused on AI, data analytics, and IoT technologies. Skill development and continuous learning are essential to ensure employees can adapt to the rapidly changing manufacturing landscape.
By staying up to date on advancements within Industry 4.0, educators can provide valuable insights and knowledge to their students, ensuring they are well-equipped to contribute to the evolution of smart factories.
Industry 4.0 and Smart Factories
As the convergence of AI, IoT, and big data analytics, Industry 4.0 and Smart Factories are transforming traditional manufacturing into an advanced, interconnected, and data-driven ecosystem. As an educator and teacher in this field, it’s crucial to invest in continuous learning and adapt curricula to address emerging technologies. This will help prepare the workforce for the challenges and opportunities presented by AI in manufacturing processes.
Future Trends in AI for Manufacturing
Exploring AI in Manufacturing: Trends and Innovations
Artificial Intelligence is making significant strides in the field of manufacturing, leading to increased efficiency, cost savings, and improved production quality. As industry trends continue evolving, it’s essential to stay informed on the latest developments and innovations.
Let’s delve into some of the most exciting trends like AI-powered collaborative robots, generative design, and the potential implementation of artificial general intelligence (AGI) within the industry, to ensure the workforce is prepared for the rapidly changing landscape of manufacturing.
AI-Powered Collaborative Robots
Collaborative robots, or “cobots,” have experienced significant growth in recent years and are transforming manufacturing as we know it today. These intelligent machines are designed to work alongside human operators safely and effectively, complementing their abilities rather than replacing them. With AI integration, cobots are becoming even more efficient and can autonomously learn and adapt to various tasks and changing environments.
One of the most exciting applications of AI-powered cobots is the ability to teach them new tasks through demonstration. By utilizing machine learning algorithms, cobots can observe humans performing a specific task and then replicate that action on their own, greatly reducing the amount of time and resources used for programming. In this sense, AI-powered cobots have the potential to revolutionize manufacturing processes by taking on more complex jobs, improving overall productivity, and minimizing human errors.
Generative Design
Generative design is another significant trend that has the potential to reshape the manufacturing landscape. By harnessing the power of AI, generative design allows engineers to explore thousands of potential design solutions based on predetermined constraints and requirements automatically. This advanced design process helps optimize the initial design stage by generating innovative and highly efficient designs that human intuition might not be able to achieve.
By utilizing generative design within manufacturing processes, companies can drastically improve their product designs, reduce material waste, and improve overall production efficiency. Furthermore, generative design can be integrated with additive manufacturing (3D printing) technology to enable the production of complex geometric shapes that would be otherwise impossible to create using traditional manufacturing methods.
Artificial General Intelligence in Manufacturing
Artificial general intelligence (AGI), also known as “strong AI,” is a theoretical form of artificial intelligence that could perform any intellectual task that a human being can do. Although AGI has not yet been achieved, its potential impact on manufacturing could be transformative.
The implementation of AGI in manufacturing processes could lead to a completely autonomous production system, where machines would have the capability to design, plan, and optimize every aspect of the manufacturing process without any human intervention. This level of automation could result in significant cost savings, more efficient operations, and increased overall safety within the industry.
However, the pursuit of AGI also raises potential concerns and ethical questions about the role of human labor in an increasingly automated world. Industry leaders and policymakers must consider these implications as they plan for the future of manufacturing.
Conclusion
In conclusion, the future of artificial intelligence in manufacturing is marked by exciting innovations and trends. AI-powered collaborative robots, generative design, and the potential implementation of artificial general intelligence all hold the potential to revolutionize the industry. As these technologies continue to advance, it is crucial for educators, teachers, and professionals in the manufacturing sector to stay informed about these developments, embrace the changes, and prepare for a future shaped by artificial intelligence.
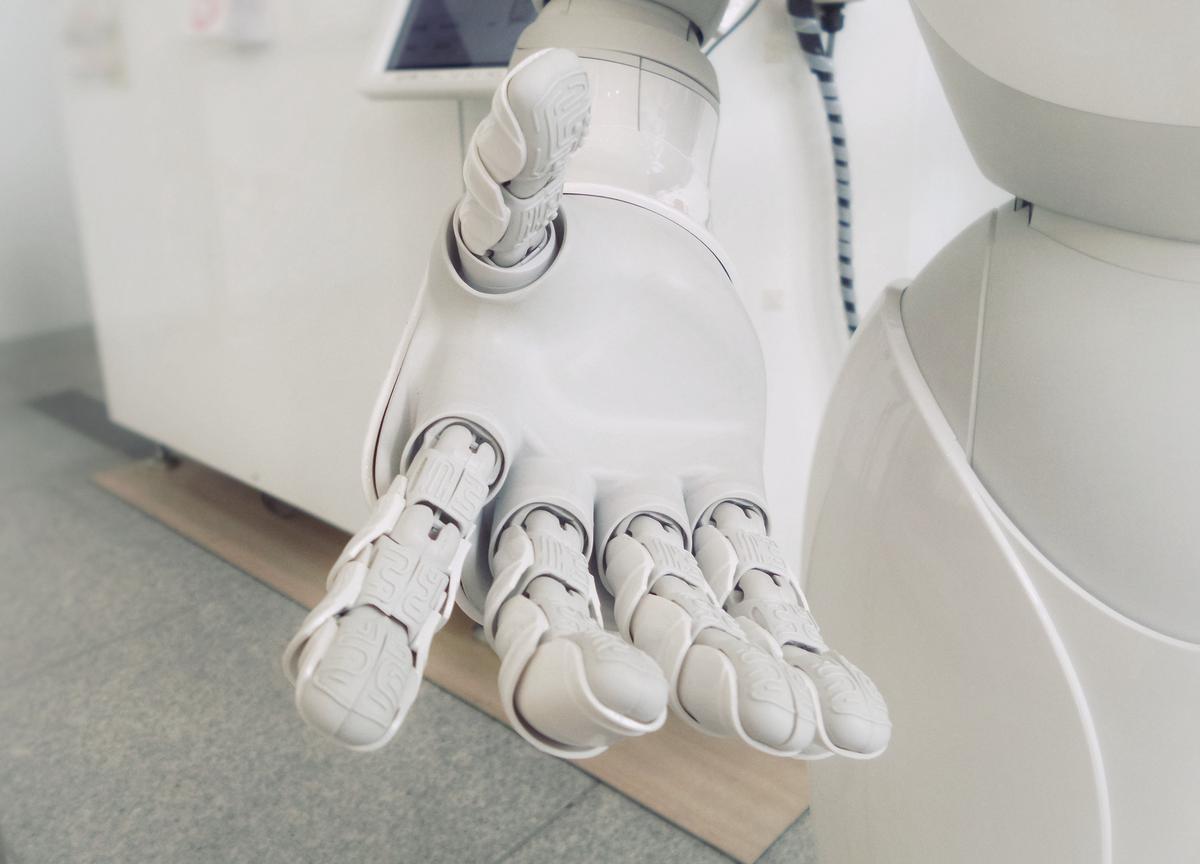
Photo by possessedphotography on Unsplash
Throughout this analysis, it is evident that AI is progressively becoming an integral part of manufacturing, driving various innovations and optimizations within the industry.
From harnessing the power of machine learning and computer vision to adopting smart factory concepts and Industry 4.0, AI technologies are progressively enhancing efficiency and product quality while addressing critical challenges.
As we navigate the continually evolving AI landscape, it is crucial for businesses and individuals to recognize the potential risks, prepare for workforce changes, and embrace the innovative opportunities that AI holds in reshaping the future of manufacturing.
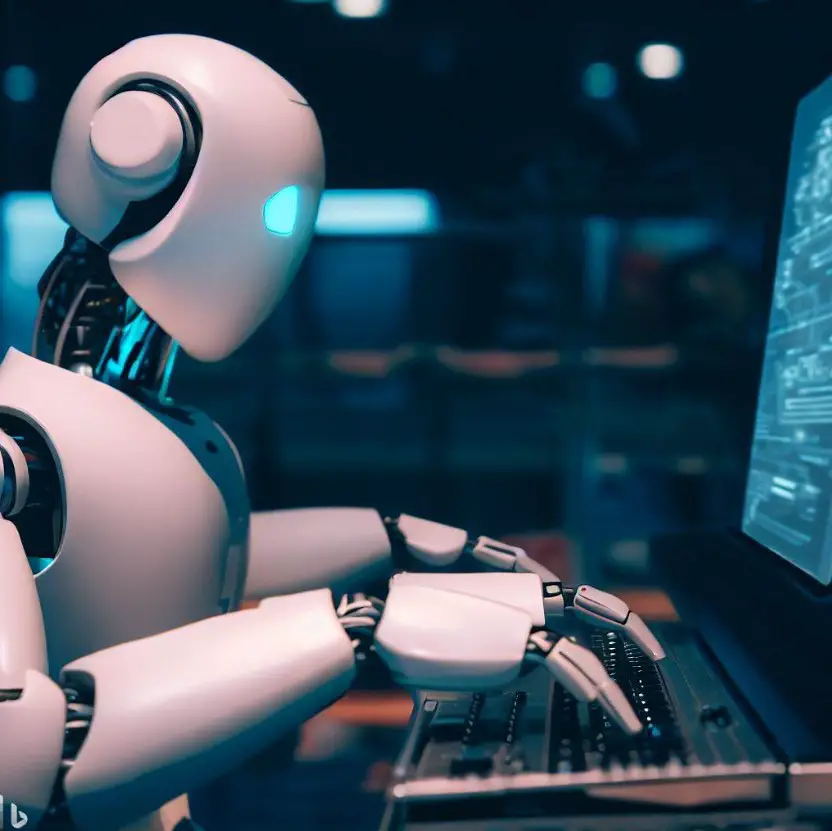
I’m Dave, a passionate advocate and follower of all things AI. I am captivated by the marvels of artificial intelligence and how it continues to revolutionize our world every single day.
My fascination extends across the entire AI spectrum, but I have a special place in my heart for AgentGPT and AutoGPT. I am consistently amazed by the power and versatility of these tools, and I believe they hold the key to transforming how we interact with information and each other.
As I continue my journey in the vast world of AI, I look forward to exploring the ever-evolving capabilities of these technologies and sharing my insights and learnings with all of you. So let’s dive deep into the realm of AI together, and discover the limitless possibilities it offers!
Interests: Artificial Intelligence, AgentGPT, AutoGPT, Machine Learning, Natural Language Processing, Deep Learning, Conversational AI.