As we stand on the cusp of a paradigm shift in artificial intelligence, one technology is leading the charge: Generative Pre-trained Transformer (GPT) models. In recent years, GPT has proved to be an exceptional advancement in the field of natural language processing, mirroring the incredible human-like ability in understanding and generating language.
This article delves into the intricacies of GPT technology, dissecting its architecture, training methods, applications, and ethical concerns to provide a comprehensive understanding of its potential and limitations in shaping the future of AI.
Overview of GPT Technology
Table of Contents
Generative Pre-trained Transformer (GPT) technology has emerged as a revolutionary development within the field of artificial intelligence (AI). This innovative method, primarily centered around natural language processing (NLP) tasks, has been developed by OpenAI, a research organization committed to advancing the study of AI. GPT’s primary objective is to enhance the understanding and generation of human-like text, driven by deep learning techniques and pre-trained models.
One of the reasons behind GPT’s massive potential is the use of transformers, which are a type of neural network architecture. The transformer concept was introduced in a paper by Vaswani et al. in 2017 and has since become a popular choice for a wide range of NLP tasks. Transformers are particularly useful in overcoming the limitations of sequential processing in recurrent neural networks, effectively improving tasks such as text comprehension, summarization, and translation.
Over time, the GPT technology has evolved through multiple iterations, with each version exhibiting improved proficiency and effectiveness in handling various NLP challenges. The first version, GPT, gave way to GPT-2, which boasted a higher amount of training data and parameters, consequently leading to better text generation capabilities. The most recent iteration, GPT-3, has been a game-changer in the AI community. It features an unprecedented 175 billion parameters and a diverse dataset, providing the model with an unparalleled understanding of context and underlying meaning within the text.
GPT technology’s significance extends beyond its impressive text generation capabilities. It has sparked a paradigm shift in the AI community, where large-scale pre-trained models have become increasingly popular. These models can be fine-tuned to achieve optimal performance, resulting in rapid and significant advancements within the field. Additionally, GPT-based models have the potential to be applied to a wide array of AI tasks, leading to innovations in fields such as healthcare, finance, and human-machine interaction.
Despite the growing popularity and adoption of GPT technology, it is crucial to acknowledge its limits, such as biased text generation, malicious use, potential lack of control over the generated text, and the high computational resources demanded for training and deploying large-scale models. However, these challenges have not hindered its impact on the artificial intelligence landscape and pushing the boundaries of natural language processing capabilities. Continuous research and development efforts in GPT technology could potentially revolutionize not just NLP, but the overall trajectory of AI.
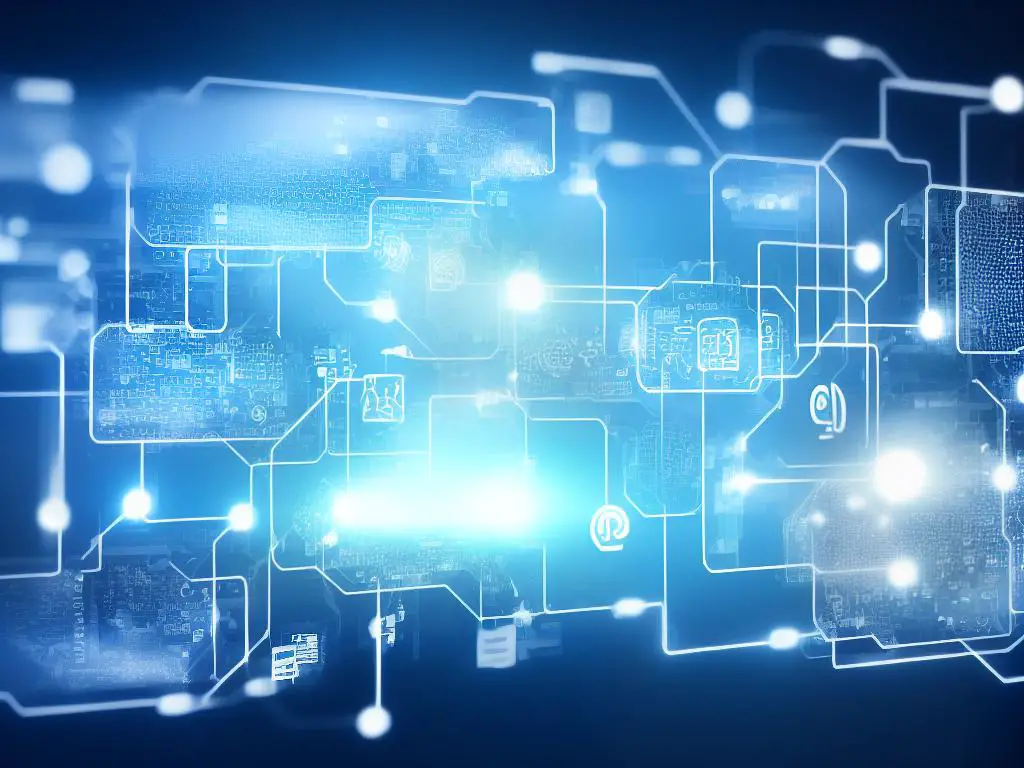
Components & Architecture
In order to achieve such impressive language understanding and generation, GPT (Generative Pre-trained Transformer) models rely on a well-designed architecture and essential components. One of the critical aspects of GPT architecture is the attention mechanism, specifically the self-attention mechanism, which enables models to determine the importance of different words in a text sequence. By calculating a score for every word pair in the input, the self-attention mechanism can discern the relevance of each word within a given context, significantly enhancing the model’s ability to produce coherent and contextually accurate text.
Another critical component of GPT models is positional encoding, a technique used to inject information about the position of each word in the input sequence. Since the attention mechanism is not sensitive to the order of the input words, positional encoding is essential in retaining the word order information. Positional encodings are added to the input embeddings to help the model understand the relationships between words, thereby enhancing its overall understanding of the language.
GPT models utilize a series of stacked encoder and decoder layers, each consisting of multi-head self-attention, normalization, and feed-forward sub-modules. These layers work together to identify and extract meaningful patterns and relationships within the data. As the input progresses through these layers, the model gains a deeper understanding of the grammar, syntax, and semantics of the language. This feature allows the GPT models to excel at various natural language processing tasks such as machine translation, text summarization, and question-answering.
The decoder in GPT architecture is responsible for generating text based on the insights gained from the encoder. It utilizes a masked self-attention mechanism, which prohibits the model from using future information while generating text. This allows the model to generate text in an autoregressive fashion, where it predicts one token at a time while conditioning on the previously generated tokens. The output of the decoder is passed through a final normalization layer and transformed into a probability distribution over the vocabulary for generating the next token.
The advancement of GPT models’ architecture, which incorporates multiple encoder and decoder layers, allows them to efficiently generalize language understanding and generate coherent text. Improvement in attention mechanisms and other model components play a crucial role in developing these state-of-the-art language models. Consequently, GPT technology has the potential to reshape the landscape of natural language processing, unlocking new possibilities for language-related applications.
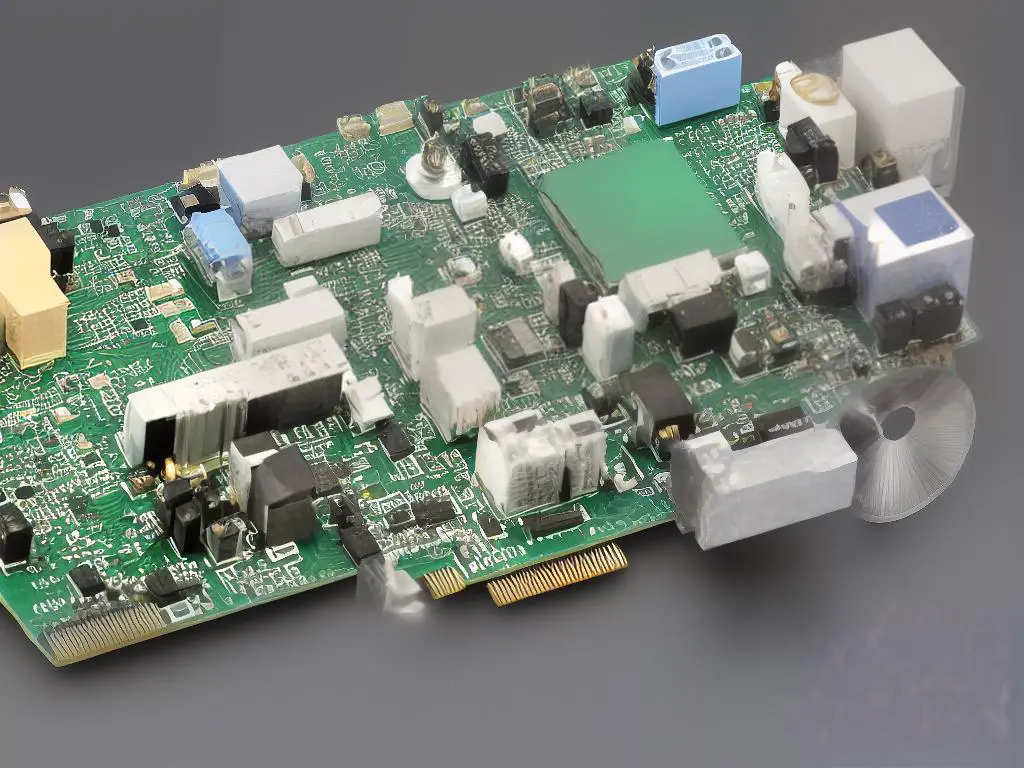
GPT-3: The Latest Evolution
Generative Pre-trained Transformer 3 (GPT-3) is the latest breakthrough in the GPT series of artificial intelligence models, offering numerous enhancements when compared to its predecessors. One of the most significant factors setting GPT-3 apart is its immense capacity, as it operates with approximately 175 billion parameters, a drastic increase from the 1.5 billion parameters found in GPT-2. This expansion has greatly elevated GPT-3’s text prediction and comprehension abilities to unprecedented levels.
The refined text generation capabilities of GPT-3 have made it exceedingly proficient at understanding context and generating natural-sounding text with impressive accuracy. This prowess in deciphering context enables the model to adapt its responses accordingly, bolstering its ability to convincingly emulate human-like written communication.
Additionally, GPT-3 possesses a remarkable facility for zero-shot or few-shot learning, which empowers it to process novel tasks without any prior training, thereby allowing for a more efficient and effective adaptation to a broader array of situations.
This extensive range of sophisticated capabilities has opened the door for GPT-3’s versatile application across numerous industries. In the realm of content creation, the technology holds the potential to revolutionize the way written materials are generated, whether it be news articles or advertising copy.
Moreover, GPT-3 may serve as a valuable asset in the development of virtual assistants, able to engage in coherent and context-driven conversation with users so as to vastly enhance user experience and incite customer loyalty in various applications such as customer support, sales, and marketing.
Beyond these industries, GPT-3 poses exciting opportunities within the fields of medicine, law, and finance. By streamlining mundane administrative duties and expediting the completion of tasks, this ground-breaking tool may free up valuable time for medical professionals to focus on providing high-quality patient care.
In the legal sector, GPT-3 can contribute to the simplification of the drafting of legal documents, the interpretation of complex legal language, and the management of research efforts. Lastly, in finance, the sophisticated text generation capabilities of GPT-3 may speed up and optimize the production of market analysis reports and financial summaries.
As GPT technology, particularly GPT-3, continues to evolve, its advancements have the potential to reshape our conceptions about the intersection between artificial intelligence and language processing. With an ever-growing suite of use cases and industries that are ripe for its application, this technology may significantly alter the way we approach and interact with various aspects of life, from daily communication to professional work environments.
Although the future of GPT technology remains uncertain, there is no denying that its revolutionary progression thus far has the potential to leave a profound and lasting impact on the world of AI and beyond.
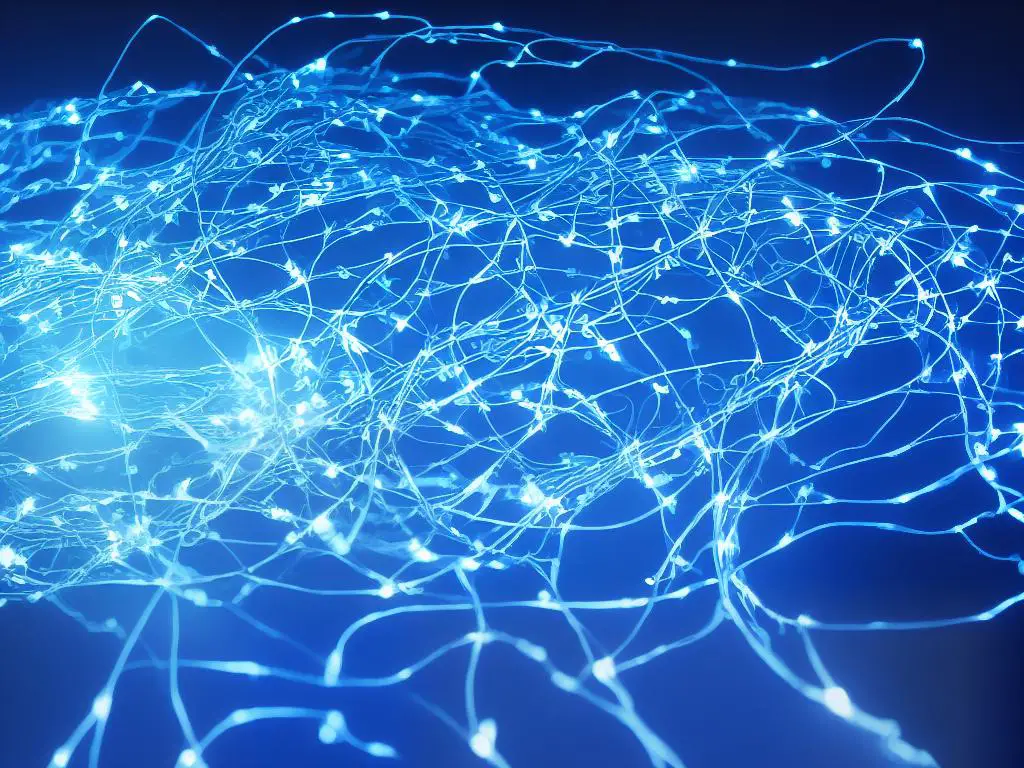
Training & Fine-tuning of AgentGPT
A key aspect that sets GPT technology apart is its reliance on unsupervised learning, allowing the models to adapt to new tasks without the need for labeled data. This approach greatly reduces the need for supervised fine-tuning, making GPT technology more versatile in addressing an extensive range of problems.
Unsupervised learning involves training the model on vast amounts of unannotated text data, enabling it to learn language structure, grammar rules, and context understanding. Consequently, GPT models can generate coherent and contextually accurate responses when interacting with users or performing various tasks.
Transfer learning is another essential aspect of GPT training, which takes advantage of pre-trained models to achieve better performance on new tasks. This approach allows the fine-tuning of GPT models using relatively smaller datasets than those required for training from scratch.
By leveraging the knowledge gained from a previously trained model, transfer learning reduces the need for extensive data and computational resources typically associated with training deep learning models. As a result, transfer learning enables GPT models to more efficiently adapt to specialized tasks in various domains.
The use of large-scale datasets in GPT training is crucial to ensure robust performance across multiple tasks. By training GPT models on vast amounts of diverse text data, they can learn to recognize patterns and relationships between different elements in the given data more effectively.
This improves the model’s ability to generalize its understanding of language across a wide range of tasks, increasing its accuracy and versatility. Furthermore, training on extensive datasets helps mitigate the risk of overfitting, in which the model begins to adapt too closely to the specific training data and loses the ability to make accurate predictions on novel input.
However, despite these strengths, there are notable weaknesses in the current GPT training methods. One significant challenge is the amount of computational resources required for training large GPT models. As these models grow in complexity, they demand extensive compute power, resulting in increased costs and environmental impact.
Additionally, the use of large-scale datasets in unsupervised learning can lead to the exacerbation of biases present in the data, which may adversely affect the model’s output.
As an industry expert exploring GPT technology, it is important to recognize the challenges faced during GPT training, such as the need for regularization techniques. These techniques, including dropout, weight decay, and gradient clipping, help maintain model performance while minimizing overfitting.
However, balancing the use of these techniques is a challenge for researchers, as both under-regularization and over-regularization can impact the overall model performance. Achieving a comprehensive understanding of the GPT training process requires acknowledging the significance of unsupervised learning, transfer learning, and large-scale datasets, while remaining aware of the technology’s current limitations and challenges.
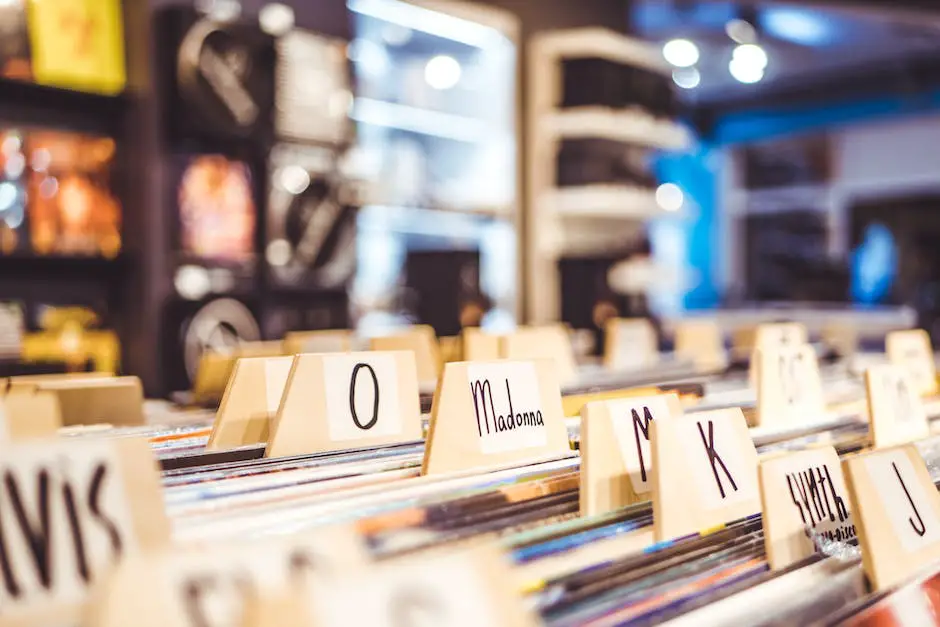
Applications & Use Cases for AgentGPT
Moving forward, it’s crucial to examine the practical applications of GPT technology across various industries such as advertising, media, and entertainment. GPT models have revolutionized content generation capabilities, enabling the automatic creation of social media posts, ad copy, and even long-form articles.
For example, both the Washington Post and the Associated Press utilize AI-powered systems to generate news articles and reports, resulting in more efficient content production. By leveraging GPT models for content generation, these industries can save time, reduce labor costs, and rapidly produce well-written texts tailored to specific audience segments.
Another area where GPT technology has made strides is code prediction and completion. Developers across the globe use coding platforms that utilize AI-based code prediction engines to provide code suggestions and auto-completion features, leading to a more streamlined development process.
With tools like GitHub’s Copilot, GPT models are now adept at understanding programming languages. This leads not only to the acceleration of software development but also helps in reducing the risk of coding errors and improving the overall code quality.
The question-answering function of GPT technology has revolutionized customer service and research. The ability of GPT models to understand human-generated text and provide accurate answers is proving to be an essential asset for chatbots used in customer support, human resources, and query resolution tools.
Companies such as Google, Facebook, and Amazon have employed these advanced AI algorithms for various purposes, from improving search engine responses to addressing customer queries on e-commerce platforms more efficiently.
In the area of language and communication, GPT technology has made significant advancements in machine translation. Deep learning models, such as GPT-3, have proven effective in recognizing and translating texts across multiple languages, leading to more accurate translation results.
This has seen widespread adoption of GPT models in the localization of software applications, websites, and content for a global audience. The technology enables businesses to cater to a diverse range of linguistic preferences, making products and services more accessible internationally.
One fascinating application of GPT technology lies in the gaming industry, where it is utilized to generate dynamic narratives for video games. By comprehending player choices and context, GPT models can adapt the storyline and dialogue to offer a more immersive and personalized experience.
Developers are investigating ways to integrate GPT models into gaming in order to create more life-like characters, transformative storytelling, and interactive game environments, signaling a bright future for this versatile technology.
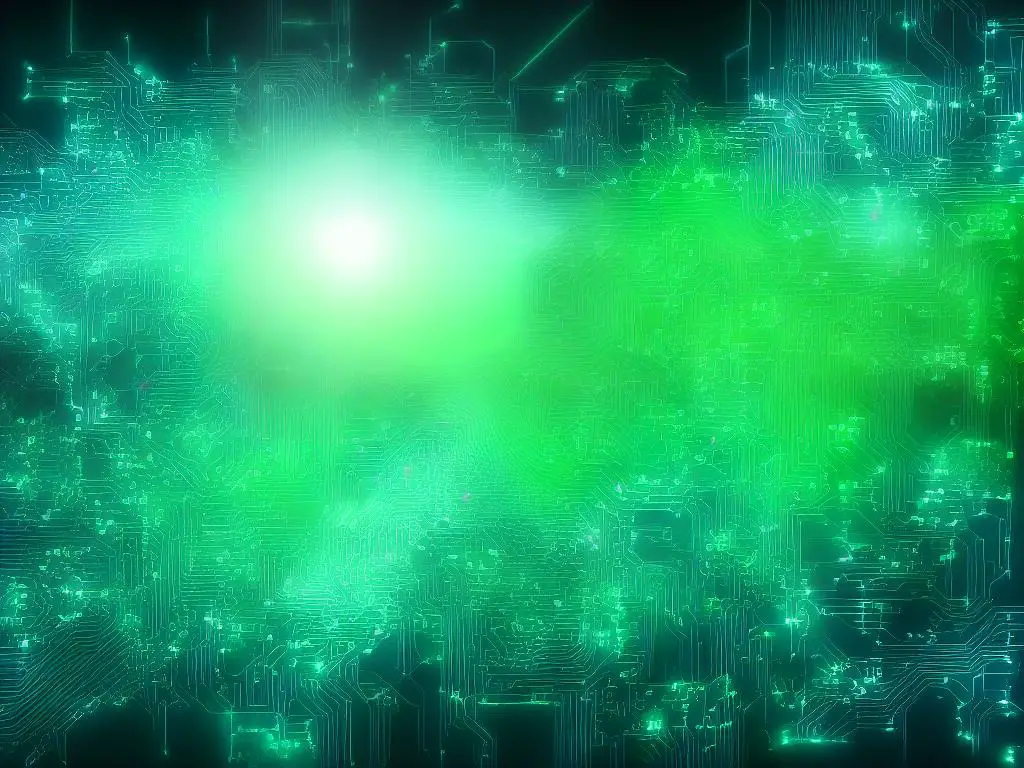
Potential Ethical Concerns & Limitations
However, a notable ethical concern associated with GPT technology is its potential to amplify biases present in its training data. As these models learn from text data on the internet, they may unintentionally adopt and perpetuate historical social, gender, or racial biases.
This could result in unfair language generation, discrimination, or exclusionary behavior. To address this issue, developers working on GPT technology are committed to researching methods for designing more equitable, transparent, and unbiased AI systems. Thorough testing and evaluation are essential to identify and correct any unintended biases in the way these models respond to various inputs.
Moreover, GPT technology also poses challenges when it comes to misinformation and malicious use. The advanced language understanding and generation capabilities of GPT systems may enable the creation of highly convincing fake news articles, hoaxes, or deepfake content.
This, in turn, can contribute to the spread of misinformation and heighten distrust in digital media. Some researchers are actively working on developing technological measures to detect AI-generated content and mitigate the risks of malicious misuse.
Data privacy constitutes another ethical concern for GPT technology. As these AI models scrape vast amounts of publicly available text data from the internet, there is a risk of incorporating private or sensitive information into the trained model. This raises concerns about the potential exposure of personal data hidden within the model’s predictions. Ensuring data privacy and protecting individuals’ information becomes paramount when designing and refining GPT technology.
An important limitation of GPT models is their inability to comprehend context and perform robust reasoning. Despite their impressive capabilities, these AI models might still generate incorrect or nonsensical responses due to gaps in their understanding of context and the intricate relationships between the various elements of a given text.
Researchers acknowledge this shortcoming and are working toward enhancing the deep learning architectures to improve context-awareness and reasoning capabilities for a more reliable and intelligent outcome.
In order to address the concerns and limitations surrounding GPT technology, developers and researchers are actively implementing more stringent guidelines for AI system behavior. Ensuring transparency and incorporating user feedback are vital in aligning these AI systems with human values.
Moreover, enhancing default behavior, allowing customization of AI values within societal norms, and seeking public input on system defaults and rules are some of the approaches being adopted to build trust and guarantee ethical deployment of GPT technology.
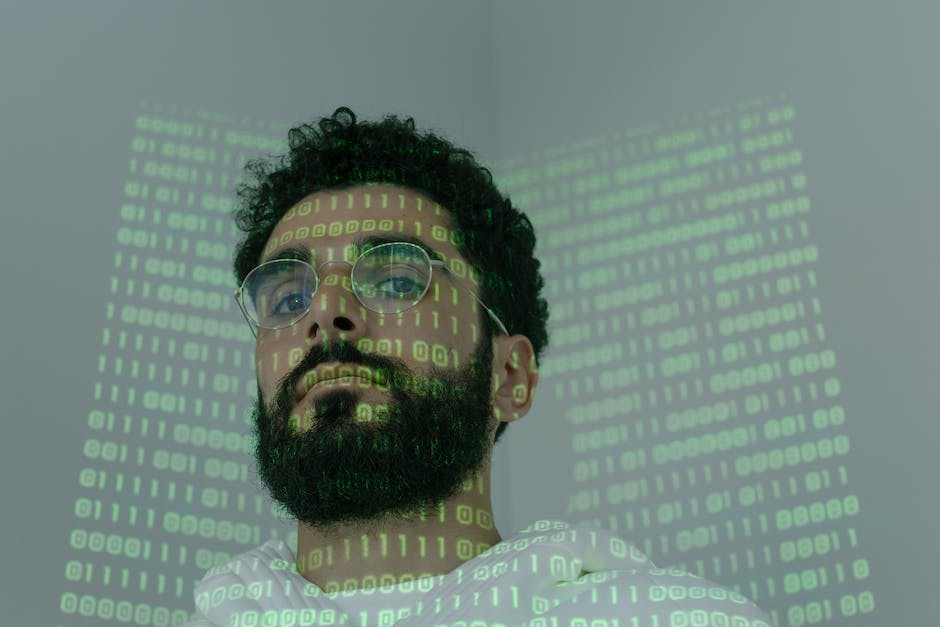
Future Developments & Research Directions
A key factor contributing to the future success of GPT technology is its ever-evolving capabilities. As demonstrated by GPT-3, these models have made significant strides in accuracy and natural language understanding. In the near future, cutting-edge research is expected to focus on achieving even greater levels of comprehension and context awareness.
This progress will drive the creation of more advanced GPT models capable of handling complicated tasks, responding to convoluted questions, and offering valuable insights across a wide range of domains.In parallel, addressing the challenges related to model size and computational resources remains a crucial aspect of advancing GPT technology.
As the models grow in size and scale, so does the need for efficient optimization methods and improved hardware compatibility. Researchers are actively exploring approaches to model compression and hardware optimization to ensure that GPT models integrate smoothly into diverse computational environments. These advancements will not only boost the performance of GPT models but also contribute to their scalability and accessibility.
Developing more efficient training methods is another area where GPT technology is expected to see significant breakthroughs. While unsupervised and self-supervised learning have shown remarkable results in language understanding, there is still considerable room for improvement. As a result, researchers are consistently working on refining training methodologies and leveraging diverse data sources to create more well-rounded and accurate models.
The adoption of transfer learning, curriculum learning, and other innovative techniques is projected to revolutionize the way GPT models are trained and fine-tuned.
Furthermore, exploring GPT technology’s potential to address broader artificial intelligence research objectives is also crucial for harnessing its full potential. Researchers are increasingly interested in understanding how GPT models can contribute to advancements in areas like reinforcement learning, computer vision, and natural language generation.
Integrating these techniques with GPT technology will enable the development of hybrid models that are capable of solving multiple challenges simultaneously, paving the way for a new generation of AI applications.
Finally, another significant direction for GPT technology development is the focus on ethics and safety. As these models learn from vast amounts of data, they may inadvertently acquire biases, offensive content, or other unwanted information.
Researchers need to develop methods for ensuring the ethical use of GPT technology, mitigating potential harm, and safeguarding users’ privacy. By addressing these critical safety concerns, GPT technology researchers will be able to guide the development and application of these models while setting a higher standard for ethical AI practices.
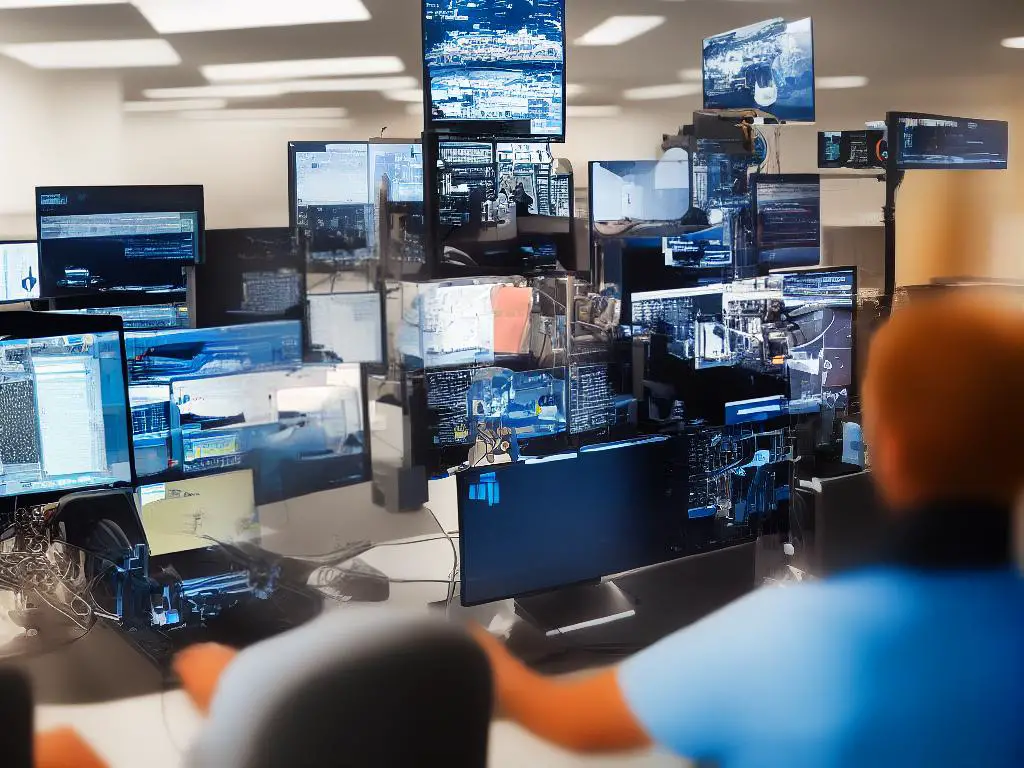
Ultimately, GPT technology holds exceptional promise in revolutionizing the way we interact with machines and how they understand our unique language abilities.
As we ponder on its remarkable achievements, limitations, and ethical concerns, it becomes increasingly important to direct our collective expertise towards the responsible development of GPT models.
By doing so, we ensure that these powerful language processing tools can augment human capabilities, transform industries, and catalyze a new era of artificial intelligence that benefits all of humanity.
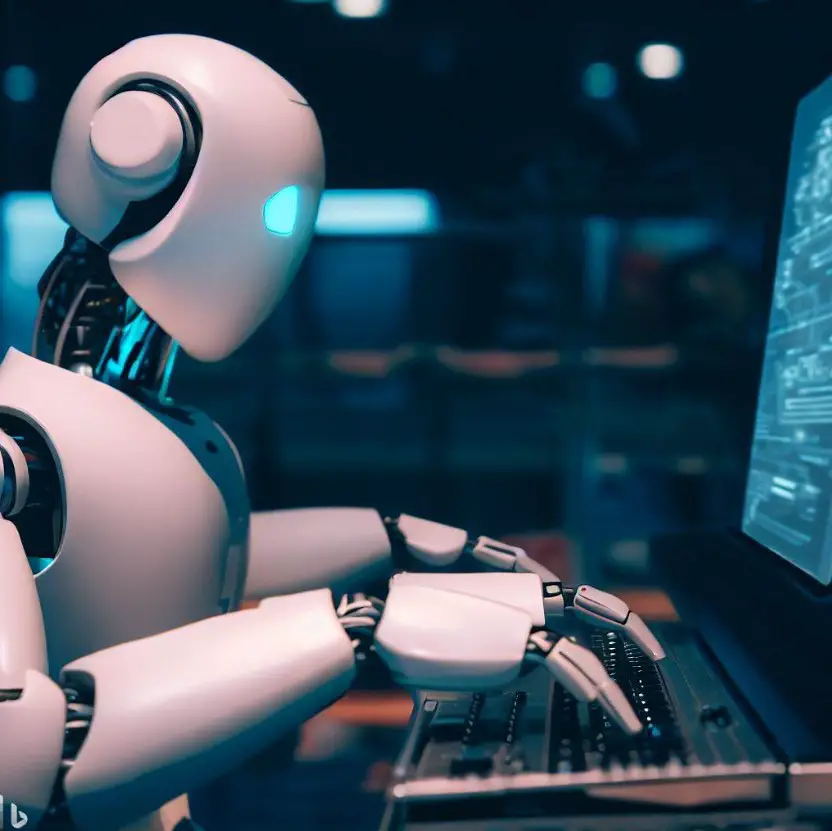
I’m Dave, a passionate advocate and follower of all things AI. I am captivated by the marvels of artificial intelligence and how it continues to revolutionize our world every single day.
My fascination extends across the entire AI spectrum, but I have a special place in my heart for AgentGPT and AutoGPT. I am consistently amazed by the power and versatility of these tools, and I believe they hold the key to transforming how we interact with information and each other.
As I continue my journey in the vast world of AI, I look forward to exploring the ever-evolving capabilities of these technologies and sharing my insights and learnings with all of you. So let’s dive deep into the realm of AI together, and discover the limitless possibilities it offers!
Interests: Artificial Intelligence, AgentGPT, AutoGPT, Machine Learning, Natural Language Processing, Deep Learning, Conversational AI.