Ever evolving and continuously advancing, the field of Artificial Intelligence (AI) has seen a radical transformation over the decades, particularly in relation to the development of AI chatbots. Shedding light on the origins of this field, from its rudimentary stages to its current, progressive state, this exploration will delve into the intricate processes and technologies behind chatbots, more specifically, generative pretrained transformers (GPT).
The aim is to provide professionals with an understanding of the sophisticated workings of AI chatbots, their bears on AI devices, their importance in today’s digital landscape, and future implications. By understanding how AI leverages natural language processing to improve human-like interactions and the extensive applications of GPT in various industries, readers can anticipate future opportunities and challenges in this rapidly evolving field.
Origins and Development of AI Chatbots
Table of Contents
Tracing the Roots of Artificial Intelligence: The Evolution of Chatbot Technology
For many people, artificial intelligence (AI) seems to be a recent phenomenon. However, the concept of AI – of machines that can think, learn, and adapt – has been in our societal consciousness for centuries. The idea of non-human intelligence can be traced as far back as ancient mythology, where animated statues were thought to possess knowledge and wisdom. But the concept of AI as we know it – computer systems designed to mimic human intelligence – began to take shape in the mid-20th century.
In 1950, the pioneering British mathematician and cryptographer, Alan Turing, proposed the idea of a “Universal Machine” that could simulate any human intelligence, laying down a significant marker in AI’s evolution. This was later developed into what we now widely recognize as the Turing Test, a method to assess a machine’s capability to exhibit intelligent behavior equivalent to, or indistinguishable from, that of a human.
In a more practical stride towards creating these intelligent machines, researchers at MIT in the 1960s began developing ELIZA, a computer program that was one of the earliest attempts at simulating human conversation. Codenamed as a “chatterbot”, ELIZA was essentially a script that could mimic conversation with a psychotherapist.
However, today’s chatbots have come a long way from ELIZA. They have been enriched by significant advances in AI and natural language processing (NLP) technologies. These advancements have allowed chatbots to not only respond based on a script but to understand and generate responses to human language in a more sophisticated manner.
Modern chatbots employ machine learning techniques that help them learn and evolve. They can now identify intents, capture entities, use sentiment analysis, and engage in a more human-like dialog. Our current generation of chatbots is significantly more advanced, being capable of understanding natural language inputs, handling complex conversations, and learning from past interactions.
The evolution of AI and chatbots is not isolated but intertwined with the evolution of computation power, algorithmic advancements, and availability of large-scale, high-dimensional data. Combining these technological advances with massive computational power and unprecedented amounts of data, chatbots have learned to understand and respond to human queries more accurately and contextually.
Deep Learning takes AI a step closer to achieving Turing’s vision of a machine that can perfectly simulate human intelligence.
While chatbot technology is advancing rapidly, it’s important to understand its roots – from the ancient concepts of non-human intelligence to the profound contributions of Turing, the rudimentary chatterbots of the past, and the cutting-edge AI-driven chatbots of the present. As we stand at the brink of a significant paradigm shift in AI, appreciating this rich history can inform our understanding of this technology and the exciting future it promises.
Understanding GPT or Generative Pretrained Transformers
Venturing deeper into the realm of artificial intelligence, chatbot technology’s vanguard is undoubtedly the innovative model of the Generative Pretrained Transformers (GPTs), particularly OpenAI’s cutting-edge GPT-3. As transformative as the leaps from Turing’s Universal Machine to ELIZA, these advanced models are shaping the progressive landscape of AI-powered conversational interfaces and advancing the trajectory of AI far beyond the original applications envisioned by early pioneers.
Generative Pretrained Transformers fundamentally redefine the function of chatbots by employing a more dynamic and responsive approach. Unlike rudimentary chatbots which relied primarily on pre-programmed responses, GPTs break new ground with their capability to generate responses on-the-fly with striking contextual understanding and grammatical accuracy. The potency of these models, and their unmatched realism, owes much to the concepts underlying their design.
The architecture is based on a transformer model that leverages self-attention mechanisms. Typically, a transformer model integrates an encoder, able to digest the input and understand its context, with a decoder, skilled in crafting a coherent and relevant output. The approach trades the sequential nature of recurrent neural networks, like LSTM, for a more parallelizable model. This inherently efficient construction drastically reduces computational burden while maintaining – even enhancing – its capability of preserving long-term dependencies in text.
The soul of a GPT lies within its unsupervised learning approach – a training regimen that goes beyond the traditional supervised learning techniques. Using massive datasets, GPT models learn from intricate patterns and nuanced contexts in language. They craft themselves into convincing zeitgeists of linguistic ability, capable of producing human-like text that is often indistinguishable from the words of a human author.
The concept of transferrable learning brings an added layer of versatility to these models. In this, the models are pretrained on a large corpora of text data, thus absorbing a robust understanding of language. They are then fine-tuned to specific tasks. This dual-pronged approach yields superior performance than training each task from scratch. Further, it enables GPTs to adapt flexibly to a multitude of tasks. Thus, similar to a human who learns a generic skill and applies it across various domains, a GPT utilizes learnings from its pretraining to numerous conversational scenarios.
Nonetheless, achieving these remarkable feats is dependent on a vast volume of quality data and a substantial computational power, echoing the sentiment that more sophisticated chatbots are an amalgam of data, algorithms, and computational prowess.
Nevertheless, GPTs are not immune to setbacks. Training these models is an energy-intensive task, raising valid concerns about their environmental viability. Further, they possess a tendency to produce content that is brilliantly eloquent, but without intrinsic understanding, echoing human wit and wisdom yet fundamentally lacking those qualities.
Generative Pretrained Transformers and their ilk, thus, represent a grand stride forward in the journey of chatbot evolution, even as they illuminate new challenges on the horizon. As we continue exploring the uncharted territories of artificial intelligence, these models act as powerful torchbearers, underscoring the intrinsic interconnectedness of computational power, algorithmic dexterity, quality data, and the irreversible march towards more intelligent machines.
Artificial Intelligence and Natural Language Processing
Pivoting away from a holistic historical exploration of AI and chatbot technology, we now delve into another fascinating development on this relentless quest for better machine-human interaction: Generative Pretrained Transformers (GPTs). Unlike traditional chatbots, the GPT models adopt a more metamorphic stance.
The architecture of a GPT is designed to allow relevant data from previous tasks to influence its approach to subsequent ones. This not only serves to improve task execution but also opens the door to a level of situational adaptation not seen before in AI systems. It is the equivalent of furnishing a Shakespearean actor with immersion and improvisation skills, making their performances not only word-perfect but tonally appropriate to a vast array of scenarios. An ability that is intricate to natural language processing!
Of course, like a skillful actor, a GPT must also prepare for its performance, relying heavily on unsupervised learning as opposed to meticulously labeled data sets. After rigorous training, these GPT models prove to be more versatile than their counterparts, able to transfer learning from one domain to another with surprising accuracy.
However, it would be an egregious error to blind ourselves to the resource demand of GPTs, both in data and computational power. This consequence stirs concerns regarding potential environmental impacts and exorbitant computational costs. Logistically, this places an invisible ceiling over advancements in this sector, emphasizing the importance of continuous attempts to optimize algorithms for efficiency.
That said, while the limitations of GPTs are clear, their role in propelling AI evolution forward cannot be dismissed. They have coerced the conversation around AI from a binary focus on rule-based or statistical models to a more nuanced discussion on the power of training across domains.
Yet, the summit of artificial intelligence, where a computer can truly understand and interact with humans in all our complexity, sentiments, and unpredictability, remains a tantalizing mirage in the distance. It is the delphic combination of semantics, pragmatics, and world knowledge that sets humanity apart. This audacious goal will undoubtedly shape the continuing conversation around AI and natural language processing and in turn, determine the challenges, barriers, and possibilities we encounter along the way. The interplay between AI and natural language processing is a dance of intricacies and nuances, a testament to the creativity and tenacity of the human spirit in emulating itself in binary form.
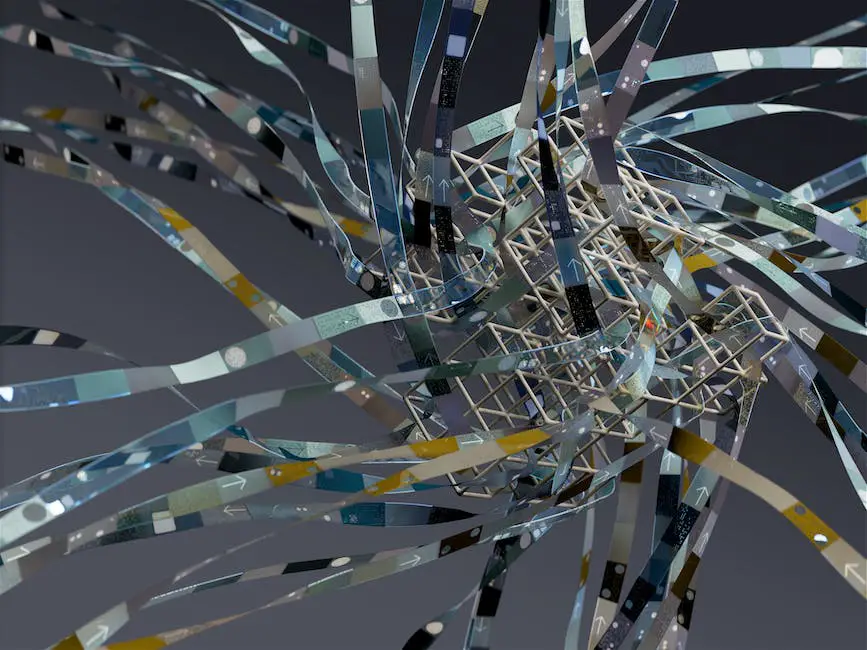
Real-life Application of GPT Chatbots
With the emergence of Generative Pretrained Transformers (GPT), we must examine how these sophisticated machine learning models have effectively reshaped chatbot applications in contemporary times. Developed by OpenAI, GPT models include GPT-1, GPT-2, and as of recent, the even larger and more robust GPT-3, each iteration highlighting advancements in scalability and performance that render these models increasingly humanlike in their responses.
GPT-enabled chatbots today have been implemented across diverse sectors, demonstrating the expansive scope of their role. In the realm of customer service, for instance, these chatbots have proven themselves as effective tools in handling client interactions, resolving inquiries in real time, and offering personalized recommendations based on the surplus of data they have been trained on. This exceptionally personal level of service serves to enhance customer experience substantially while reducing the burden on human customer service teams.
In the healthcare industry, GPT-enabled chatbots represent a potential revolution in patient care, with the ability to provide immediate responses to medical queries, assist in symptom triage, and provide valuable resources or information. The promise lies not only in cumulative data or speed but in the AI’s ability to exhibit empathy through carefully calibrated responses, a testament to the model’s intricate understanding of human language and sentiment.
Education also stands to benefit from these advanced chatbots. With the capacity to tutor in multiple subjects, GPT-enabled chatbots can furnish detailed explanations, correct student errors, or offer targeted practice problems. These chatbots may supplement traditional instruction and provide comprehensive learning tools available around the clock.
GPT chatbots are also paving the way in content creation. They can generate ideas, draft articles, and even pen entire stories. These bots no longer simply string together keywords but now produce content that is coherent, engaging, and humanlike in its creativity.
However, despite these promising applications, the use of GPT in chatbots is not void of challenges. Foremost among them is the risk of misuse, especially with regards to generating deceptive or offensive content. Ensuring ethical use of this technology will be crucial as it becomes more ubiquitous.
Moreover, the quality of responses from GPT chatbots is largely contingent on the quality and diversity of the input data. The current lack of effective mechanisms to guide these chatbots mid-conversation means there is still room for improvement in achieving a truly conversational AI. Verification and validation also pose challenges for these models due to their black-box nature.
As we step forward into an era where GPT chatbots become increasingly intertwined with our daily lives, continuous scrutiny will be required to balance the remarkable capabilities they bestow upon us with the ethical and social implications that they present. This exploration and evaluation will be a testament not just to the potential of AI, but the ability of society to employ these advancements towards the sustainable betterment of all.
Predicting Future Potential and Challenges
Building on the architectural prowess of Generative Pretrained Transformers (GPTs), there’s been a phenomenal progression in their scalability and performance. With each subsequent iteration from GPT-1 to GPT-3, there has been a marked increase in the capacity of these models. They’ve grown in size, encompassing billions of parameters that underpin their burgeoning proficiency in language tasks.
The applicability of these GPT models is fully realized when implemented into chatbots, particularly in industries such as customer service. In these arenas, GPT-powered chatbots have shown remarkable potential in interpreting customer queries, providing accurate responses, and optimizing businesses’ interaction with their clientele. With time, they’re expected to handle even complex consumer behaviors, disputes, and resolutions, thus reshaping customer service paradigms.
Looking beyond customer service, the healthcare industry presents ample opportunities for the deployment of these chatbots. GPT-enabled chatbots can revolutionize patient interactions, symptom checking, health consultations, and even mental health services. Potential applications extend further to education, where they promote personalized learning by catering to the needs, pace, and comprehension level of the varied student body.
Notwithstanding its obvious merits, the use of GPT technology in chatbots is not without challenges. Misuse of these chatbots for nefarious purposes raises grave ethical considerations. An AI technology that can potentially mimic human-like text generation can be exploited to generate fake news, spew hate speech, or deceive unsuspecting individuals. The quality of data used to train these chatbots can also bear significant impact on their outputs, underscoring the importance of robust data collection and management processes.
Moreover, the inherent limitations in conversational AI pose significant hurdles. While GPT chatbots can respond appropriately to queries most of the time, they can still falter when met with ambiguity, abstract concepts, or cultural nuances. They are still to master the art of context retention and understanding the intent behind user inputs.
Another concern lies in the verification and validation of these chatbots. Given the wide range of outputs they can potentially generate, how should we go about assessing their correctness or appropriateness? Traditional test cases might be rendered obsolete, and new validation strategies ought to be devised.
Lastly, it falls upon the researchers, developers, and stakeholders to continuously scrutinize and evaluate GPT chatbots for their ethical and social implications. This ongoing supervision forms an integral part of ensuring that these advanced AI models serve humanity beneficially and responsibly. When properly harnessed, there is much hope that GPT chatbots can significantly bolster our advancements in the field of artificial intelligence. Tomorrow awaits this exciting transformation, with bated breath.
Ethical and Social Implications of AI Chatbots
As the narrative unfolds, one becomes conscious of the palpable ethical and social implications that inevitably accompany the deployment of AI chatbots. While portraying the undeniable utility of AI chatbots and the transformations happening due to advancements like Generative Pretrained Transformers (GPTs), some pressing concerns deserve equal attention. These points of contention, stressing the ethical and social aspects, uncover the potential real-life effects and long-term impacts of such digital solutions.
On the ethical front, data privacy emerges as a predominant concern. Chatbot applications inherently require interaction with an array of data, often of a highly personal nature. Regardless of the encryption or anonymization methods employed, the extent to which these chatbots require access to private data can be disconcerting, especially as data breaches and leaks become increasingly common. This fact is often deepens with the integration of GPT-enabled chatbots, given their robust data requirements due to the vast neural network structures.
The phenomenon of AI deception or AI fakery is another ethical dilemma. Fundamentally, chatbots mimic human conversation, and with advancements in Natural Language Processing and machine learning techniques, it might be increasingly difficult for users to distinguish between a bot and a human. Deception, as acceptable as it may seem in the context of providing seamless service, poses challenging questions about the integrity and transparency of AI systems.
The deployment of AI chatbots, especially in crucial sectors like healthcare, raises concerns about accountability. To illustrate these concerns, consider a scenario where a GPT-powered health-chatbot misinterprets symptoms and provides inaccurate or harmful suggestions. The identification of who should be held accountable – the algorithm, the developer, or the entity employing the chatbot – remains a grey area.
The ethics of AI chatbots also envelop the elements of misuse or manipulation. The potential of these systems to be wielded with malicious intent is not insignificant, especially considering complex GPT models’ capacity to generate human-like textual outputs. The possibilities range from the spread of disinformation or propaganda to the manipulation of user behavior and opinion.
Correspondingly, the social implications of deploying AI chatbots are equally far-reaching. The fear of job displacement is a prominent social concern. With GPT-enabled chatbots constantly improving their conversational capabilities, customer service representatives, retail assistants, and even content creators could find their roles threatened. The balance between technological innovation and human employment needs to be delicately managed.
Observe also the role of AI chatbots in further accentuating the digital divide. The widespread use of chatbot applications, particularly in essential services, may disadvantage individuals without sufficient digital literacy or access to digital platforms.
Meanwhile, cultural and linguistic inclusivity remains a challenge. Despite the advancements, chatbots, including those powered by GPT models, frequently struggle to comprehend and return responses in a culturally nuanced manner. As such, AI chatbots can inadvertently contribute to a certain degree of cultural homogenization, deprioritizing the diversity that defines human societies.
As the AI chatbot landscape continues to evolve, we cannot undermine these ethical and social implications. The need of the hour is a commitment towards robust regulatory frameworks, rigorous algorithmic audits, and an ethos of building AI with social consciousness. The creation of any technology should not outpace the contemplation of its broader implications on society. AI chatbots and tools like Generative Pretrained Transformers stand as another testament to this axiom. Encapsulating the essence of this narrative requires not simply celebrating the evolution of technology but also fostering an understanding of its intricacies and reverberations through the society we share.
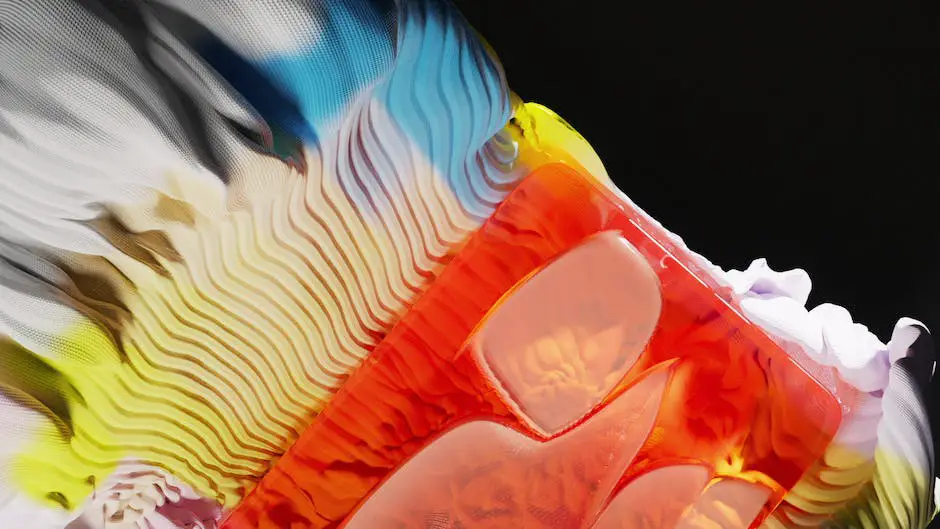
As we embrace the new age of AI and GPT chatbots, it is essential to observe responsible and ethical usage, taking into consideration crucial aspects like data privacy, user consent and potential social implications. This responsibility is not limited to developers and researchers but spans to regulators and end-users too.
While we marvel at the advancement and wide applications of AI chatbots, we ought to simultaneously be conscious of the risks involved and act responsibly to foster a healthy balance between digital progression and ethical considerations.
Future advancements in AI chatbots will undoubtedly bring myriad opportunities, but alongside it will come new challenges that we must be ready to confront and handle with care. The journey of exploring, grappling with, and triumphing over these challenges in AI chatbot technology remains an ongoing, immersive adventure in the realm of Artificial Intelligence.
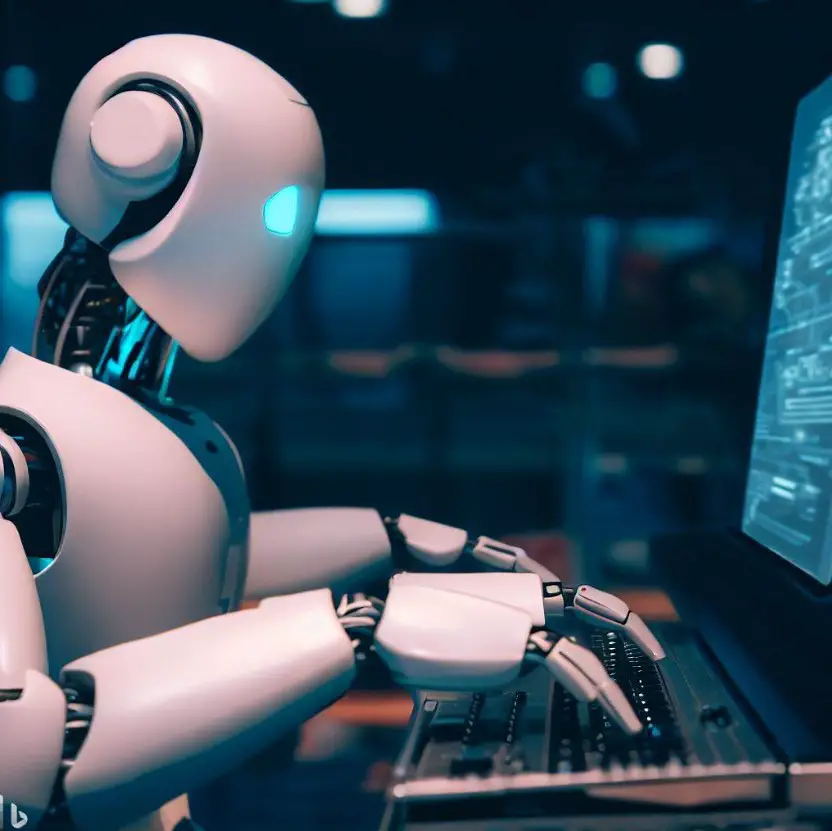
I’m Dave, a passionate advocate and follower of all things AI. I am captivated by the marvels of artificial intelligence and how it continues to revolutionize our world every single day.
My fascination extends across the entire AI spectrum, but I have a special place in my heart for AgentGPT and AutoGPT. I am consistently amazed by the power and versatility of these tools, and I believe they hold the key to transforming how we interact with information and each other.
As I continue my journey in the vast world of AI, I look forward to exploring the ever-evolving capabilities of these technologies and sharing my insights and learnings with all of you. So let’s dive deep into the realm of AI together, and discover the limitless possibilities it offers!
Interests: Artificial Intelligence, AgentGPT, AutoGPT, Machine Learning, Natural Language Processing, Deep Learning, Conversational AI.