In this rapidly evolving digital age, the phenomenon of social media has become an integral part of our lives. With billions of users connected across various platforms, it is now more important than ever for enthusiasts and hobbyists to explore the potential implications of artificial intelligence (AI) in social media analysis.
This essay aims to provide a comprehensive understanding of the essential aspects, techniques, and tools necessary for developing expertise in AI-driven techniques for social media analysis.
Understanding AI and Social Media
Table of Contents
Artificial intelligence (AI) has become an essential tool in a broad range of industries, particularly in the realm of social media analysis. By leveraging various AI methodologies, including machine learning (ML), natural language processing (NLP), and deep learning, researchers and analysts can address complex challenges in the field of social media.
With the help of AI algorithms, accurate predictions, sentiment analysis, and customer engagement patterns can be deciphered, providing valuable insights into user behavior and preferences.
One key application of AI in social media analysis is sentiment analysis, which determines the emotional tone and intent behind user-generated content.
By utilizing NLP techniques to parse text, AI can detect users’ emotions (positive, negative, or neutral) and identify key opinion trends. This can be particularly useful for companies seeking to understand and respond to their customers’ views regarding products, services, or overall brand perception.
Another valuable application of AI in social media analysis is the identification and tracking of influential users and content. Through ML algorithms, it is possible to recognize users who consistently have a higher impact on specific topics and discussions. Brands can then leverage this information to target and collaborate with these influencers, enhancing their marketing campaigns and maximizing their reach. Moreover, AI can help identify viral content, allowing brands to capitalize on trending topics and engage with their audience in a timely manner.
AI can also assist brands in optimizing their social media posting schedules and content strategies. By analyzing user engagement levels and preferences across multiple platforms, AI algorithms can identify the most effective posting times and content types that drive maximum engagement. This valuable information allows businesses to plan their content calendars more efficiently, resulting in increased visibility and better audience retention.
In conclusion, AI technology offers significant advantages in the realm of social media analysis, making it an indispensable tool in today’s digital world. By uncovering valuable insights that may have otherwise been overlooked, AI allows organizations to gain a deeper understanding of their audiences, refine their messaging, and ultimately enhance their overall social media presence.
As AI technology continues to advance, so too will the potential for more sophisticated social media analysis, paving the way for businesses to create more targeted and effective strategies.
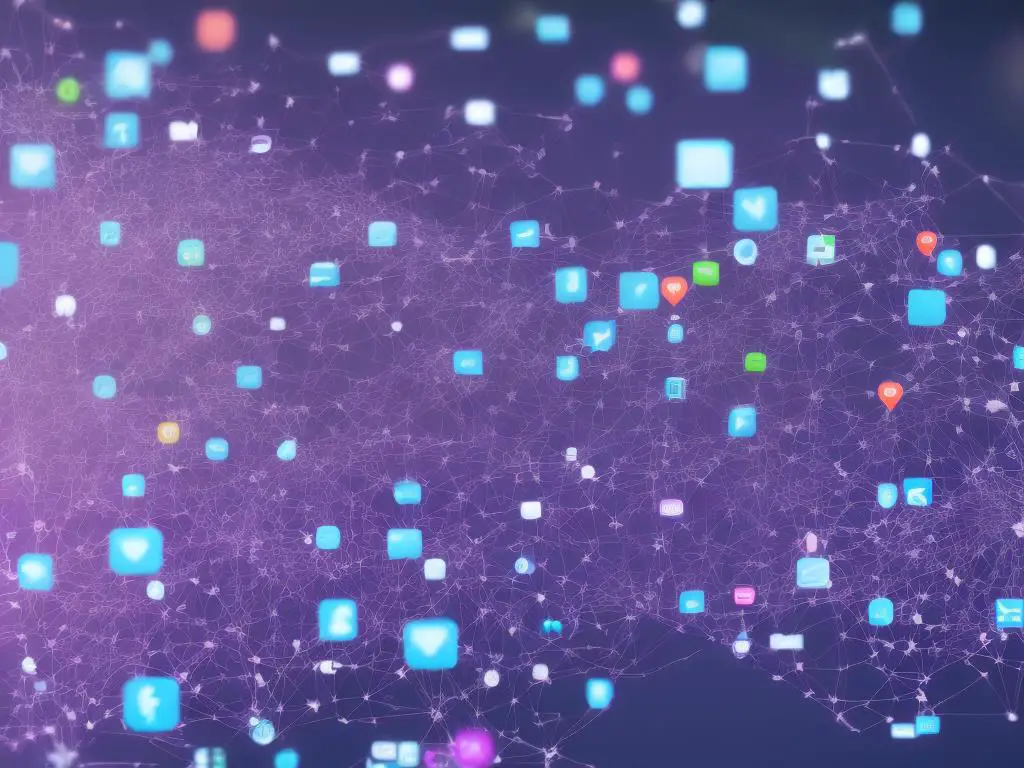
Natural Language Processing (NLP) Techniques
Moving forward, one pivotal aspect of AI in social media analysis is Natural Language Processing (NLP). This subfield of artificial intelligence focuses on the interaction between computers and humans through natural language.
NLP encompasses various techniques and algorithms that enable computers to effectively understand, interpret, and generate human languages. By incorporating NLP into their social media analysis, organizations can glean even richer insights into user behavior, sentiment, and trends, leading to more informed decision-making and improved marketing strategies.
One such crucial application of NLP in social media analysis is sentiment analysis. Sentiment analysis refers to the process of identifying, extracting, and determining the polarity of emotions and opinions expressed in a text. In social media analysis, this technique helps in understanding customers’ opinions towards products, services, or any trending topics, which can be invaluable for businesses, policymakers, and researchers.
Another important NLP technique in social media analysis is text summarization. With the increasing amount of data generated every day on social media platforms, it has become challenging for businesses and researchers to extract relevant insights and make sense of them.
Text summarization helps in generating a condensed version of lengthy text by capturing the most important points. Automatic summarization not only saves time but also provides a way to efficiently comprehend the gist of the content. In the context of social media analysis, this technique can be applied to summarize users’ reviews, comments, or discussions on a particular subject or topic.
Topic modeling is another useful NLP technique for social media analysis. It is a form of unsupervised learning that aims to automatically discover latent topics or themes from a large collection of documents. By employing algorithms like Latent Dirichlet Allocation (LDA), topic modeling groups similar words together and assigns them to corresponding topics.
This helps in understanding the structure and content of a large corpus of textual data and identify underlying patterns in the text. In the realm of social media analysis, exploring the hidden topics of discussion enables businesses and researchers to gather insights on users’ interests and preferences and make better informed decisions.
Furthermore, NLP-based entity recognition is quite useful in analyzing social media data. Named Entity Recognition (NER) is the process of identifying and classifying predefined categories such as names of people, organizations, locations, expressions of time, or other entities within the text.
By identifying and extracting such entities, meaningful patterns and relationships can be drawn, which can further help analyze brand mentions, influencers, or market trends in the context of social media.
One area where Natural Language Processing (NLP) can deliver significant benefits in social media analysis is through machine translation. Considering that social media is a global platform used by people from diverse linguistic backgrounds, it becomes essential to analyze data in multiple languages.
Machine translation helps bridge this language barrier by automating the translation of text from one language to another, ensuring comprehensibility and accessibility of cross-lingual information. This allows businesses and researchers to gain insights into global social media trends and user behavior across different languages.
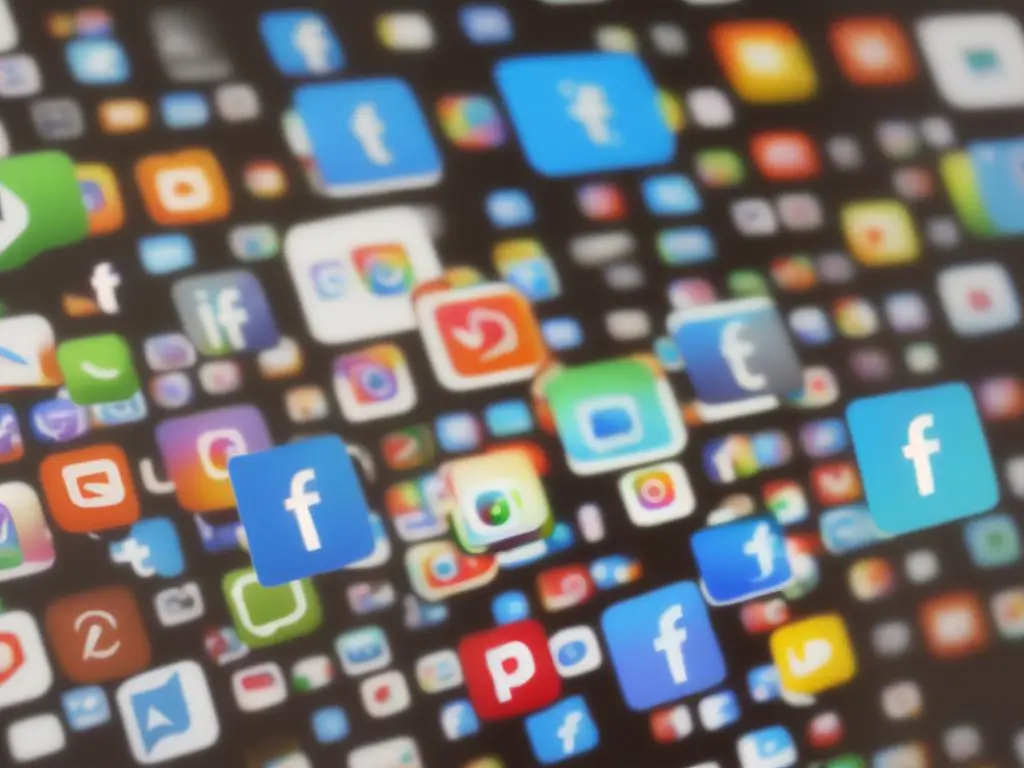
Social Network Analysis
Building upon machine translation, Social Network Analysis (SNA) serves as another powerful tool for understanding the dynamics and structure of social media networks. With the help of AI algorithms, SNA can be further enhanced and optimized.
By examining the connections and relationships between users on social media platforms, AI-driven SNA provides valuable insights into user behavior, online communities, and factors that make certain individuals more influential than others.
As a result, researchers, marketers, and other stakeholders can better understand and engage with their target audience, while also identifying trends and influencers that can drive engagement and conversions.
One of the fundamental aspects of SNA involves assessing the structure of social networks, including the density and centrality of connections between users. AI algorithms can be applied to identify key individuals within a network, as well as to uncover and categorize clusters of users with similar interests, behavior, or demographics.
This information can then be used to inform marketing and communication strategies or to target specific user segments for optimal engagement.
Another significant component of AI-driven SNA is the detection and analysis of communities within social media networks. By leveraging machine learning algorithms, the process of identifying groups of users who share similar interests, beliefs, or affiliations becomes expedited and more accurate.
Through proper identification, and understanding of these communities, organizations can better align their social media strategies to appeal to and engage with distinct user segments effectively.
Predicting influential users within social networks is another critical element of AI-powered SNA. By using various machine learning techniques, such as regression analysis and decision trees, along with metrics like engagement rates, reach, and network centrality, AI can help determine which users are the most influential within their respective networks.
This information is essential to businesses that seek to identify key influencers for marketing campaigns or to assess the credibility and reach of potential brand ambassadors.
Moreover, AI algorithms combined with Social Network Analysis (SNA) play a crucial role in understanding the sentiment of social media content and identifying trends in user opinions and preferences. By employing natural language processing techniques, AI can categorize and examine textual data found in user posts, comments, and messages.
This enables the identification of recurring themes and sentiments among users, which can then be utilized to customize online content, products, or services, catering to the needs of specific user segments. In summary, the application of AI in social network analysis is a burgeoning field with immense potential for improving research and promoting data-driven decision-making in devising social media strategies.
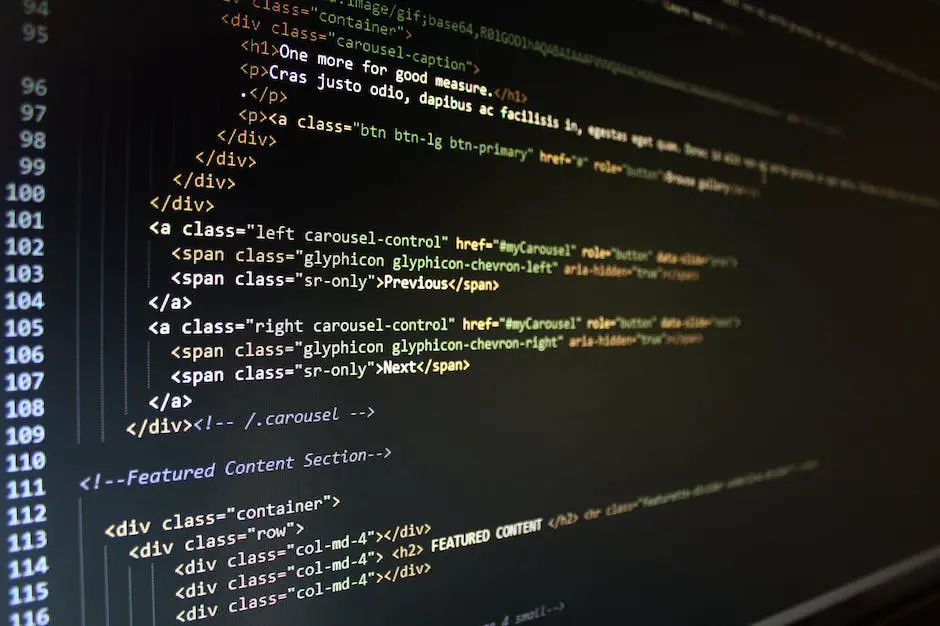
Data Collection and Preparation
AI for Social Media Analysis involves gathering data from various platforms such as Facebook, Twitter, Instagram, and LinkedIn. This can be achieved using APIs or third-party tools like Tweepy, which allow for seamless data collection and integration.
As a result, enthusiasts and hobbyists looking to become skilled in AI for Social Media Analysis can start by familiarizing themselves with these tools and techniques, expanding their knowledge and expertise in the field.
This investment in learning will not only enable them to better understand and navigate the social media landscape but also unlock a plethora of opportunities for innovation and growth in this rapidly evolving domain.
Data cleaning is crucial in ensuring data quality and relevance, which involves removing irrelevant data, handling missing data, and eliminating duplicates. Techniques like data imputation, transformation, tokenization, lemmatization, and stemming can be used.
Preprocessing helps refine data by removing noise, such as special characters, emojis, links, and variations in language usage. Feature extraction and scaling can also be used.Data transformation involves converting the cleaned and preprocessed data into numerical representations using methods like one-hot encoding or word embeddings like Word2Vec or GloVe.
As an enthusiast or hobbyist diving into AI for social media analysis, it is essential to prioritize ethical considerations such as user privacy and responsible use of user-generated content. Implementing proper data management and security measures should be at the forefront of your learning journey to ensure the ethical handling of sensitive information.
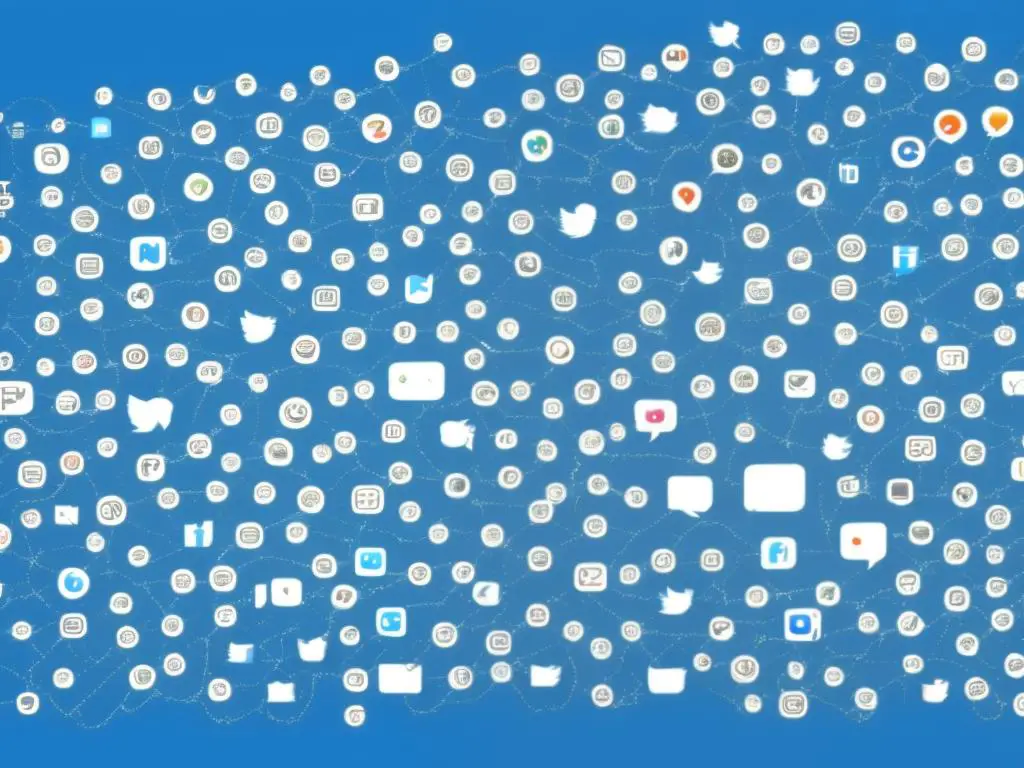
Machine Learning Techniques that AgentGPT Uses
Moving beyond ethics, it’s important to understand that machine learning techniques have become the cornerstone of social media analysis due to their inherent ability to analyze large datasets quickly and efficiently.
One such technique is clustering, which groups similar data points together based on their features. This is particularly useful in social media analysis for discovering patterns and trends among users or identifying communities.
For example, clustering can help identify groups of people who share common interests or opinions, allowing for more targeted marketing or tailored content recommendations. By integrating ethical considerations with AI techniques like clustering, you can responsibly embark on your journey to become skilled in social media analysis using artificial intelligence.
Classification is another machine learning technique that finds application in social media analysis. It involves identifying the categories or classes that different data points belong to, allowing analysts to make predictions about new, unseen data points.
In the context of social media, classification models can be used for tasks such as sentiment analysis and spam detection. Sentiment analysis involves categorizing text data as positive, negative, or neutral, making it useful for gauging public opinion on specific topics, products, or campaigns.
Meanwhile, spam detection helps filter out unwanted content, ensuring users have an enjoyable and safe experience when consuming social media.
Regression models are also valuable when it comes to social media analysis. These models are designed to predict numerical values based on input features, making them ideal for tasks like estimating user engagement or predicting the success of a post.
By using regression models, marketers and content creators can optimize their posts for maximum exposure and engagement, targeting specific user demographics and ensuring a higher return on their investment.
Deep learning techniques, which are a subset of machine learning, have garnered significant attention due to their ability to process and learn patterns from complex data. In social media analysis, deep learning can be used to perform tasks like image and video recognition, natural language processing, and personalized recommendations.
For instance, deep learning algorithms can analyze and extract valuable information from visual content, such as identifying brand logos or popular products in user-generated media. This information can then be used to tailor marketing campaigns, design new products or features, and better understand user preferences.
In summary, utilizing machine learning techniques for social media analysis provides invaluable insights into user behavior and preferences. By harnessing clustering, classification, and regression models, along with advanced deep learning techniques, businesses can gain a competitive edge by better understanding their target audience and optimizing their content for maximum impact.
Ultimately, these techniques empower organizations to engage their audiences on a deeper level and forge stronger connections with their customers.
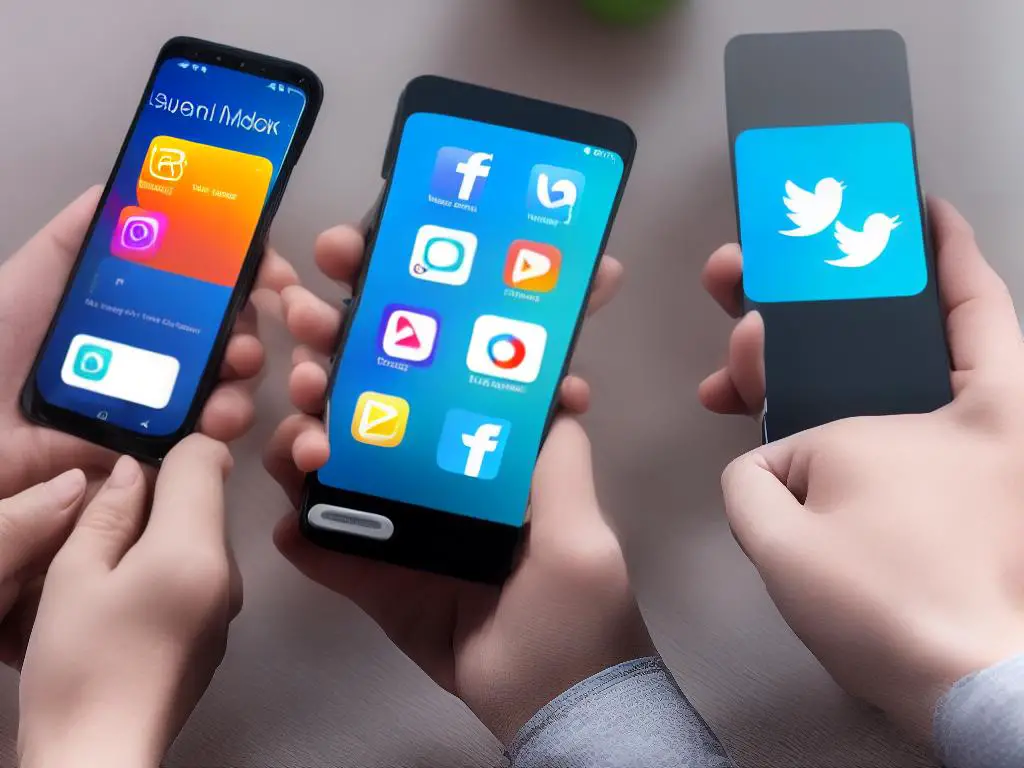
Visualizations and Reporting
Visualizations are crucial in effectively presenting the results of social media analysis, as they transform complex data sets into easily understandable graphical representations, ranging from simple charts and graphs to more sophisticated and interactive dashboards.
By leveraging AI techniques, data scientists can efficiently analyze massive amounts of social media data, uncover patterns and trends, and present them through visually engaging tools. In turn, this not only aids organizations in grasping their audience but also enables them to make data-driven decisions for marketing strategies, product development, and customer engagement.
One method to create effective visualizations is to use AI-powered tools that can automatically analyze textual and visual content in a way that humans cannot. For instance, natural language processing (NLP) algorithms can be employed to understand the sentiment and emotions expressed in text, while computer vision techniques can decipher patterns and trends in multimedia content.
In social media analysis, these AI techniques can be used to generate not just quantitative metrics such as the number of likes and shares but also qualitative aspects like user opinions and brand perception.
In addition to generating deeper insights, AI can also help in developing interactive dashboards that are essential in visualizing real-time social media data.
By incorporating AI algorithms into the dashboard design, businesses can create customized, dynamic visualizations that continuously update and adapt as the underlying data changes. This capability significantly enhances the user experience, allowing stakeholders to explore the data through various angles and understand the impact of their actions on the metrics being measured.
Moreover, visualization techniques are also crucial in identifying influential users on social media platforms. By creating network graphs that map connections between users, AI algorithms can analyze the flow of information and identify key influencers who possess the power to shape public opinion. This information is invaluable for businesses looking to connect with these influencers and leverage their reach for widening their audience and improving brand recognition.
In conclusion, as social media platforms continue to amass large volumes of data, harnessing the power of AI-driven visualizations is indispensable for understanding and interpreting this information. By combining advanced techniques such as NLP and computer vision with interactive dashboards, an engaging and powerful way of presenting social media analysis results can be achieved.
This enables businesses to uncover hidden patterns, trends, and connections, making informed decisions on their engagement strategies and optimizing their presence on social media.
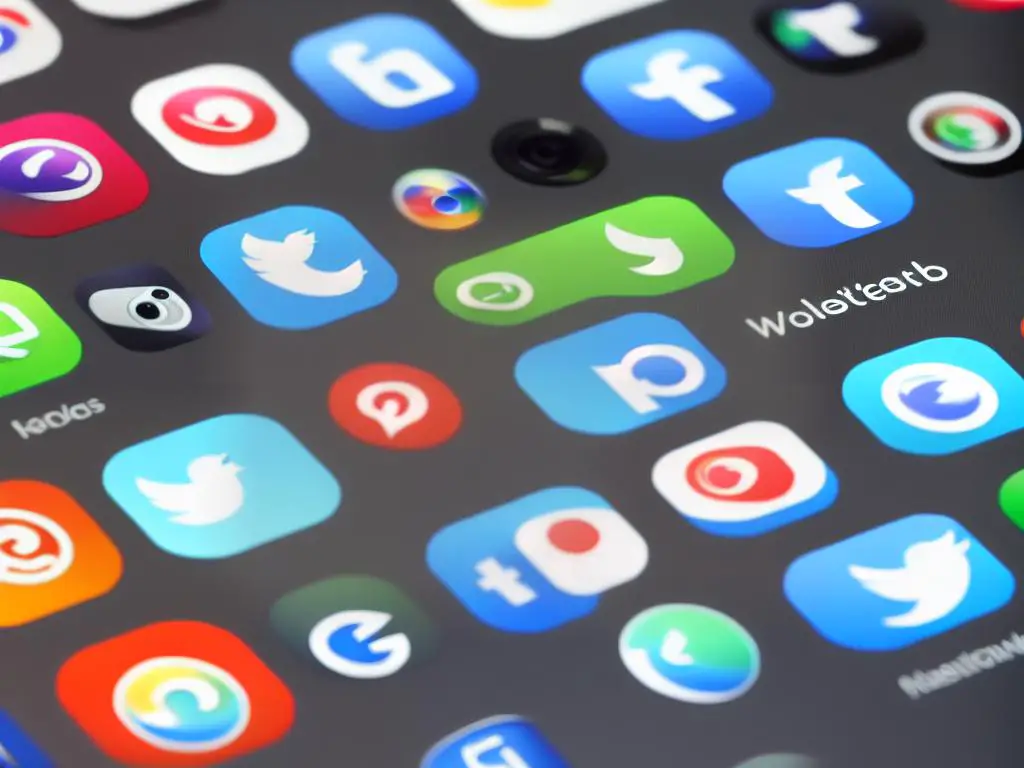
Innovative Tools and Applications
One of the key benefits offered by artificial intelligence in social media analysis is its ability to process vast amounts of data, extracting valuable insights for businesses and users alike.
As AI tools and applications continue to develop and grow, enthusiasts and hobbyists now have the opportunity to harness these innovations, opening up a new world of efficient and data-driven projects for social media analysis. The cutting-edge tools available today have been designed to optimize the way users interpret and utilize the enormous amount of big data available on social media platforms.
A cutting-edge application worth exploring is sentiment analysis. Sentiment analysis, also known as opinion mining, refers to the AI-powered analysis of public sentiment towards a brand or product by interpreting social media conversations.
Tools like Brandwatch, MonkeyLearn, and Lexalytics make sentiment analysis simple and accessible, offering users the ability to track brand sentiment, assess campaign performance, and even detect potential PR crises.
Another powerful AI-driven application is content recommendation. Content recommendation engines collect data from social media platforms to understand user preferences, enabling curated content suggestions tailored to users’ interests. Content recommendation systems, such as Froomle and LiftIgniter, provide businesses with the guidance needed to optimize their social media posts, meet audience interests, and ultimately maximize user engagement.
The innovative field of image recognition has also grown exponentially, thanks to advancements in deep learning and AI. Platforms like Clarifai and Google Cloud Vision use AI-driven algorithms to analyze visual content from social media channels. With image recognition capabilities, these platforms enable businesses to better understand user-generated content, detect trends, and monitor brand or logo exposure. This discovery allows for a more comprehensive perspective on consumer behavior and interests.
Conversational AI is another area of social media analysis where AI tools such as Chatbots and personal assistants are taking center stage. By leveraging natural language processing, these solutions provide real-time customer support, engage users in meaningful conversations, and collect insightful data on user preferences.
Tools like DigitalGenius and IBM Watson Assistant help businesses optimize their social media presence, improve customer relationships, and streamline their social media marketing initiatives. By using these innovative applications, enthusiasts and hobbyists can truly unlock the potential of AI in social media analysis and improve the effectiveness of their data-driven projects.
By understanding and implementing the fundamental principles of AI and its applications in social media analysis, enthusiasts can uncover new possibilities and insights that greatly benefit both individuals and society.
As we continue pushing the limits of what AI can achieve in deciphering social media data, we are presented with a powerful tool that aids in decision-making and understanding patterns, while uncovering hidden connections between users.
The knowledge gained from this exploration will enable hobbyists and professionals alike to harness the power of AI in transforming the way we utilize and interact with social media data.
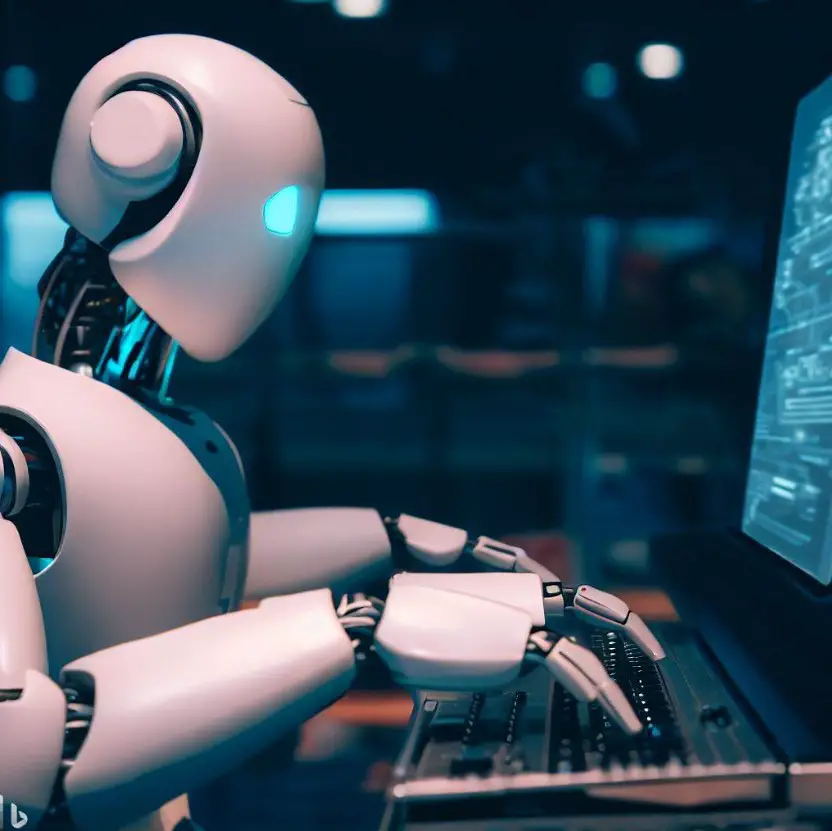
I’m Dave, a passionate advocate and follower of all things AI. I am captivated by the marvels of artificial intelligence and how it continues to revolutionize our world every single day.
My fascination extends across the entire AI spectrum, but I have a special place in my heart for AgentGPT and AutoGPT. I am consistently amazed by the power and versatility of these tools, and I believe they hold the key to transforming how we interact with information and each other.
As I continue my journey in the vast world of AI, I look forward to exploring the ever-evolving capabilities of these technologies and sharing my insights and learnings with all of you. So let’s dive deep into the realm of AI together, and discover the limitless possibilities it offers!
Interests: Artificial Intelligence, AgentGPT, AutoGPT, Machine Learning, Natural Language Processing, Deep Learning, Conversational AI.